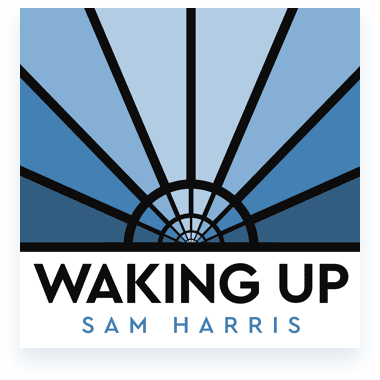
MIRI senior researcher Eliezer Yudkowsky was recently invited to be a guest on Sam Harris’ “Waking Up” podcast. Sam is a neuroscientist and popular author who writes on topics related to philosophy, religion, and public discourse.
The following is a complete transcript of Sam and Eliezer’s conversation, AI: Racing Toward the Brink.
Contents
- 1. Intelligence and generality — 0:05:26
- 2. Orthogonal capabilities and goals in AI — 0:25:21
- 3. Cognitive uncontainability and instrumental convergence — 0:53:39
- 4. The AI alignment problem — 1:09:09
- 5. No fire alarm for AGI — 1:21:40
- 6. Accidental AI, mindcrime, and MIRI — 1:34:30
- 7. Inadequate equilibria — 1:44:40
- 8. Rapid capability gain in AGI — 1:59:02
1. Intelligence and generality (0:05:26)
Sam Harris: I am here with Eliezer Yudkowsky. Eliezer, thanks for coming on the podcast.
Eliezer Yudkowsky: You’re quite welcome. It’s an honor to be here.
Sam: You have been a much requested guest over the years. You have quite the cult following, for obvious reasons. For those who are not familiar with your work, they will understand the reasons once we get into talking about things. But you’ve also been very present online as a blogger. I don’t know if you’re still blogging a lot, but let’s just summarize your background for a bit and then tell people what you have been doing intellectually for the last twenty years or so.
Eliezer: I would describe myself as a decision theorist. A lot of other people would say that I’m in artificial intelligence, and in particular in the theory of how to make sufficiently advanced artificial intelligences that do a particular thing and don’t destroy the world as a side-effect. I would call that “AI alignment,” following Stuart Russell.
Other people would call that “AI control,” or “AI safety,” or “AI risk,” none of which are terms that I really like.
I also have an important sideline in the art of human rationality: the way of achieving the map that reflects the territory and figuring out how to navigate reality to where you want it to go, from a probability theory / decision theory / cognitive biases perspective. I wrote two or three years of blog posts, one a day, on that, and it was collected into a book called Rationality: From AI to Zombies.
Sam: Which I’ve read, and which is really worth reading. You have a very clear and aphoristic way of writing; it’s really quite wonderful. I highly recommend that book.
Eliezer: Thank you, thank you.
Sam: Your background is unconventional. For instance, you did not go to high school, correct? Let alone college or graduate school. Summarize that for us.
Eliezer: The system didn’t fit me that well, and I’m good at self-teaching. I guess when I started out I thought I was going to go into something like evolutionary psychology or possibly neuroscience, and then I discovered probability theory, statistics, decision theory, and came to specialize in that more and more over the years.
Sam: How did you not wind up going to high school? What was that decision like?
Eliezer: Sort of like a mental crash around the time I hit puberty—or like a physical crash, even. I just did not have the stamina to make it through a whole day of classes at the time. (laughs) I’m not sure how well I’d do trying to go to high school now, honestly. But it was clear that I could self-teach, so that’s what I did.
Sam: And where did you grow up?
Eliezer: Chicago, Illinois.
Sam: Let’s fast forward to the center of the bull’s eye for your intellectual life here. You have a new book out, which we’ll talk about second. Your new book is Inadequate Equilibria: Where and How Civilizations Get Stuck. Unfortunately, I’ve only read half of that, which I’m also enjoying. I’ve certainly read enough to start a conversation on that. But we should start with artificial intelligence, because it’s a topic that I’ve touched a bunch on in the podcast which you have strong opinions about, and it’s really how we came together. You and I first met at that conference in Puerto Rico, which was the first of these AI safety / alignment discussions that I was aware of. I’m sure there have been others, but that was a pretty interesting gathering.
So let’s talk about AI and the possible problem with where we’re headed, and the near-term problem that many people in the field and at the periphery of the field don’t seem to take the problem (as we conceive it) seriously. Let’s just start with the basic picture and define some terms. I suppose we should define “intelligence” first, and then jump into the differences between strong and weak or general versus narrow AI. Do you want to start us off on that?
Eliezer: Sure. Preamble disclaimer, though: In the field in general, not everyone you ask would give you the same definition of intelligence. A lot of times in cases like those it’s good to sort of go back to observational basics. We know that in a certain way, human beings seem a lot more competent than chimpanzees, which seems to be a similar dimension to the one where chimpanzees are more competent than mice, or that mice are more competent than spiders. People have tried various theories about what this dimension is, they’ve tried various definitions of it. But if you went back a few centuries and asked somebody to define “fire,” the less wise ones would say: “Ah, fire is the release of phlogiston. Fire is one of the four elements.” And the truly wise ones would say, “Well, fire is the sort of orangey bright hot stuff that comes out of wood and spreads along wood.” They would tell you what it looked like, and put that prior to their theories of what it was.
So what this mysterious thing looks like is that humans can build space shuttles and go to the Moon, and mice can’t, and we think it has something to do with our brains.
Sam: Yeah. I think we can make it more abstract than that. Tell me if you think this is not generic enough to be accepted by most people in the field: Whatever intelligence may be in specific contexts, generally speaking it’s the ability to meet goals, perhaps across a diverse range of environments. We might want to add that it’s at least implicit in the “intelligence” that interests us that it means an ability to do this flexibly, rather than by rote following the same strategy again and again blindly. Does that seem like a reasonable starting point?
Eliezer: I think that that would get fairly widespread agreement, and it matches up well with some of the things that are in AI textbooks.
If I’m allowed to take it a bit further and begin injecting my own viewpoint into it, I would refine it and say that by “achieve goals” we mean something like “squeezing the measure of possible futures higher in your preference ordering.” If we took all the possible outcomes, and we ranked them from the ones you like least to the ones you like most, then as you achieve your goals, you’re sort of squeezing the outcomes higher in your preference ordering. You’re narrowing down what the outcome would be to be something more like what you want, even though you might not be able to narrow it down very exactly.
Flexibility. Generality. Humans are much more domain–general than mice. Bees build hives; beavers build dams; a human will look over both of them and envision a honeycomb-structured dam. We are able to operate even on the Moon, which is very unlike the environment where we evolved.
In fact, our only competitor in terms of general optimization—where “optimization” is that sort of narrowing of the future that I talked about—is natural selection. Natural selection built beavers. It built bees. It sort of implicitly built the spider’s web, in the course of building spiders.
We as humans have this similar very broad range to handle this huge variety of problems. And the key to that is our ability to learn things that natural selection did not preprogram us with; so learning is the key to generality. (I expect that not many people in AI would disagree with that part either.)
Sam: Right. So it seems that goal-directed behavior is implicit (or even explicit) in this definition of intelligence. And so whatever intelligence is, it is inseparable from the kinds of behavior in the world that result in the fulfillment of goals. So we’re talking about agents that can do things; and once you see that, then it becomes pretty clear that if we build systems that harbor primary goals—you know, there are cartoon examples here like making paperclips—these are not systems that will spontaneously decide that they could be doing more enlightened things than (say) making paperclips.
This moves to the question of how deeply unfamiliar artificial intelligence might be, because there are no natural goals that will arrive in these systems apart from the ones we put in there. And we have common-sense intuitions that make it very difficult for us to think about how strange an artificial intelligence could be. Even one that becomes more and more competent to meet its goals.
Let’s talk about the frontiers of strangeness in AI as we move from here. Again, though, I think we have a couple more definitions we should probably put in play here, differentiating strong and weak or general and narrow intelligence.
Eliezer: Well, to differentiate “general” and “narrow” I would say that this is on the one hand theoretically a spectrum, and on the other hand, there seems to have been a very sharp jump in generality between chimpanzees and humans.
So, breadth of domain driven by breadth of learning—DeepMind, for example, recently built AlphaGo, and I lost some money betting that AlphaGo would not defeat the human champion, which it promptly did. Then a successor to that was AlphaZero. AlphaGo was specialized on Go; it could learn to play Go better than its starting point for playing Go, but it couldn’t learn to do anything else. Then they simplified the architecture for AlphaGo. They figured out ways to do all the things it was doing in more and more general ways. They discarded the opening book—all the human experience of Go that was built into it. They were able to discard all of these programmatic special features that detected features of the Go board. They figured out how to do that in simpler ways, and because they figured out how to do it in simpler ways, they were able to generalize to AlphaZero, which learned how to play chess using the same architecture. They took a single AI and got it to learn Go, and then reran it and made it learn chess. Now that’s not human general, but it’s a step forward in generality of the sort that we’re talking about.
Sam: Am I right in thinking that that’s a pretty enormous breakthrough? I mean, there’s two things here. There’s the step to that degree of generality, but there’s also the fact that they built a Go engine—I forget if it was Go or chess or both—which basically surpassed all of the specialized AIs on those games over the course of a day. Isn’t the chess engine of AlphaZero better than any dedicated chess computer ever, and didn’t it achieve that with astonishing speed?
Eliezer: Well, there was actually some amount of debate afterwards whether or not the version of the chess engine that it was tested against was truly optimal. But even to the extent that it was in that narrow range of the best existing chess engines, as Max Tegmark put it, the real story wasn’t in how AlphaGo beat human Go players. It’s in how AlphaZero beat human Go system programmers and human chess system programmers. People had put years and years of effort into accreting all of the special-purpose code that would play chess well and efficiently, and then AlphaZero blew up to (and possibly past) that point in a day. And if it hasn’t already gone past it, well, it would be past it by now if DeepMind kept working it. Although they’ve now basically declared victory and shut down that project, as I understand it.
Sam: So talk about the distinction between general and narrow intelligence a little bit more. We have this feature of our minds, most conspicuously, where we’re general problem-solvers. We can learn new things and our learning in one area doesn’t require a fundamental rewriting of our code. Our knowledge in one area isn’t so brittle as to be degraded by our acquiring knowledge in some new area, or at least this is not a general problem which erodes our understanding again and again. And we don’t yet have computers that can do this, but we’re seeing the signs of moving in that direction. And so it’s often imagined that there is a kind of near-term goal—which has always struck me as a mirage—of so-called “human-level” general AI.
I don’t see how that phrase will ever mean much of anything, given that all of the narrow AI we’ve built thus far is superhuman within the domain of its applications. The calculator in my phone is superhuman for arithmetic. Any general AI that also has my phone’s ability to calculate will be superhuman for arithmetic. But we must presume it will be superhuman for all of the dozens or hundreds of specific human talents we’ve put into it, whether it’s facial recognition or just memory, unless we decide to consciously degrade it. Access to the world’s data will be superhuman unless we isolate it from data. Do you see this notion of human-level AI as a landmark on the timeline of our development, or is it just never going to be reached?
Eliezer: I think that a lot of people in the field would agree that human-level AI, defined as “literally at the human level, neither above nor below, across a wide range of competencies,” is a straw target, is an impossible mirage. Right now it seems like AI is clearly dumber and less general than us—or rather that if we’re put into a real-world, lots-of-things-going-on context that places demands on generality, then AIs are not really in the game yet. Humans are clearly way ahead. And more controversially, I would say that we can imagine a state where the AI is clearly way ahead across every kind of cognitive competency, barring some very narrow ones that aren’t deeply influential of the others.
Like, maybe chimpanzees are better at using a stick to draw ants from an ant hive and eat them than humans are. (Though no humans have practiced that to world championship level.) But there’s a sort of general factor of, “How good are you at it when reality throws you a complicated problem?” At this, chimpanzees are clearly not better than humans. Humans are clearly better than chimps, even if you can manage to narrow down one thing the chimp is better at. The thing the chimp is better at doesn’t play a big role in our global economy. It’s not an input that feeds into lots of other things.
There are some people who say this is not possible—I think they’re wrong—but it seems to me that it is perfectly coherent to imagine an AI that is better at everything (or almost everything) than we are, such that if it was building an economy with lots of inputs, humans would have around the same level of input into that economy as the chimpanzees have into ours.
Sam: Yeah. So what you’re gesturing at here is a continuum of intelligence that I think most people never think about. And because they don’t think about it, they have a default doubt that it exists. This is a point I know you’ve made in your writing, and I’m sure it’s a point that Nick Bostrom made somewhere in his book Superintelligence. It’s this idea that there’s a huge blank space on the map past the most well-advertised exemplars of human brilliance, where we don’t imagine what it would be like to be five times smarter than the smartest person we could name, and we don’t even know what that would consist in, because if chimps could be given to wonder what it would be like to be five times smarter than the smartest chimp, they’re not going to represent for themselves all of the things that we’re doing that they can’t even dimly conceive.
There’s a kind of disjunction that comes with more. There’s a phrase used in military contexts. The quote is variously attributed to Stalin and Napoleon and I think Clausewitz and like a half a dozen people who have claimed this quote. The quote is, “Sometimes quantity has a quality all its own.” As you ramp up in intelligence, whatever it is at the level of information processing, spaces of inquiry and ideation and experience begin to open up, and we can’t necessarily predict what they would be from where we sit.
How do you think about this continuum of intelligence beyond what we currently know, in light of what we’re talking about?
Eliezer: Well, the unknowable is a concept you have to be very careful with. The thing you can’t figure out in the first 30 seconds of thinking about it—sometimes you can figure it out if you think for another five minutes. So in particular I think that there’s a certain narrow kind of unpredictability which does seem to be plausibly in some sense essential, which is that for AlphaGo to play better Go than the best human Go players, it must be the case that the best human Go players cannot predict exactly where on the Go board AlphaGo will play. If they could predict exactly where AlphaGo would play, AlphaGo would be no smarter than them.
On the other hand, AlphaGo’s programmers and the people who knew what AlphaGo’s programmers were trying to do, or even just the people who watched AlphaGo play, could say, “Well, I think the system is going to play such that it will win at the end of the game.” Even if they couldn’t predict exactly where it would move on the board.
Similarly, there’s a (not short, or not necessarily slam-dunk, or not immediately obvious) chain of reasoning which says that it is okay for us to reason about aligned (or even unaligned) artificial general intelligences of sufficient power as if they’re trying to do something, but we don’t necessarily know what. From our perspective that still has consequences, even though we can’t predict in advance exactly how they’re going to do it.
2. Orthogonal capabilities and goals in AI (0:25:21)
Sam: I think we should define this notion of alignment. What do you mean by “alignment,” as in the alignment problem?
Eliezer: It’s a big problem. And it does have some moral and ethical aspects, which are not as important as the technical aspects—or pardon me, they’re not as difficult as the technical aspects. They couldn’t exactly be less important.
But broadly speaking, it’s an AI where you can say what it’s trying to do. There are narrow conceptions of alignment, where you’re trying to get it to do something like cure Alzheimer’s disease without destroying the rest of the world. And there’s much more ambitious notions of alignment, where you’re trying to get it to do the right thing and achieve a happy intergalactic civilization.
But both the narrow and the ambitious alignment have in common that you’re trying to have the AI do that thing rather than making a lot of paperclips.
Sam: Right. For those who have not followed this conversation before, we should cash out this reference to “paperclips” which I made at the opening. Does this thought experiment originate with Bostrom, or did he take it from somebody else?
Eliezer: As far as I know, it’s me.
Sam: Oh, it’s you, okay.
Eliezer: It could still be Bostrom. I asked somebody, “Do you remember who it was?” and they searched through the archives of the mailing list where this idea plausibly originated and if it originated there, then I was the first one to say “paperclips.”
Sam: All right, then by all means please summarize this thought experiment for us.
Eliezer: Well, the original thing was somebody expressing a sentiment along the lines of, “Who are we to constrain the path of things smarter than us? They will create something in the future; we don’t know what it will be, but it will be very worthwhile. We shouldn’t stand in the way of that.”
The sentiments behind this are something that I have a great deal of sympathy for. I think the model of the world is wrong. I think they’re factually wrong about what happens when you take a random AI and make it much bigger.
In particular, I said, “The thing I’m worried about is that it’s going to end up with a randomly rolled utility function whose maximum happens to be a particular kind of tiny molecular shape that looks like a paperclip.” And that was the original paperclip maximizer scenario.
It got a little bit distorted in being whispered on, into the notion of: “Somebody builds a paperclip factory and the AI in charge of the paperclip factory takes over the universe and turns it all into paperclips.” There was a lovely online game about it, even. But this still sort of cuts against a couple of key points.
One is: the problem isn’t that paperclip factory AIs spontaneously wake up. Wherever the first artificial general intelligence is from, it’s going to be in a research lab specifically dedicated to doing it, for the same reason that the first airplane didn’t spontaneously assemble in a junk heap.
And the people who are doing this are not dumb enough to tell their AI to make paperclips, or make money, or end all war. These are Hollywood movie plots that the script writers do because they need a story conflict and the story conflict requires that somebody be stupid. The people at Google are not dumb enough to build an AI and tell it to make paperclips.
The problem I’m worried about is that it’s technically difficult to get the AI to have a particular goal set and keep that goal set and implement that goal set in the real world, and so what it does instead is something random—for example, making paperclips. Where “paperclips” are meant to stand in for “something that is worthless even from a very cosmopolitan perspective.” Even if we’re trying to take a very embracing view of the nice possibilities and accept that there may be things that we wouldn’t even understand, that if we did understand them we would comprehend to be of very high value, paperclips are not one of those things. No matter how long you stare at a paperclip, it still seems pretty pointless from our perspective. So that is the concern about the future being ruined, the future being lost. The future being turned into paperclips.
Sam: One thing this thought experiment does: it also cuts against the assumption that a sufficiently intelligent system, a system that is more competent than we are in some general sense, would by definition only form goals, or only be driven by a utility function, that we would recognize as being ethical, or wise, and would by definition be aligned with our better interest. That we’re not going to build something that is superhuman in competence that could be moving along some path that’s as incompatible with our wellbeing as turning every spare atom on Earth into a paperclip.
But you don’t get our common sense unless you program it into the machine, and you don’t get a guarantee of perfect alignment or perfect corrigibility (the ability for us to be able to say, “Well, that’s not what we meant, come back”) unless that is successfully built into the machine. So this alignment problem is—the general concern is that even with the seemingly best goals put in, we could build something (especially in the case of something that makes changes to itself—and we’ll talk about this, the idea that these systems could become self-improving) whose future behavior in the service of specific goals isn’t totally predictable by us. If we gave it the goal to cure Alzheimer’s, there are many things that are incompatible with it fulfilling that goal, and one of those things is our turning it off. We have to have a machine that will let us turn it off even though its primary goal is to cure Alzheimer’s.
I know I interrupted you before. You wanted to give an example of the alignment problem—but did I just say anything that you don’t agree with, or are we still on the same map?
Eliezer: We’re still on the same map. I agree with most of it. I would of course have this giant pack of careful definitions and explanations built on careful definitions and explanations to go through everything you just said. Possibly not for the best, but there it is.
Stuart Russell put it, “You can’t bring the coffee if you’re dead,” pointing out that if you have a sufficiently intelligent system whose goal is to bring you coffee, even that system has an implicit strategy of not letting you switch it off. Assuming that all you told it to do was bring the coffee.
I do think that a lot of people listening may want us to back up and talk about the question of whether you can have something that feels to them like it’s so “smart” and so “stupid” at the same time—like, is that a realizable way an intelligence can be?
Sam: Yeah. And that is one of the virtues—or one of the confusing elements, depending on where you come down on this—of this thought experiment of the paperclip maximizer.
Eliezer: Right. So, I think that there are multiple narratives about AI, and I think that the technical truth is something that doesn’t fit into any of the obvious narratives. For example, I think that there are people who have a lot of respect for intelligence, they are happy to envision an AI that is very intelligent, it seems intuitively obvious to them that this carries with it tremendous power, and at the same time, their respect for the concept of intelligence leads them to wonder at the concept of the paperclip maximizer: “Why is this very smart thing just making paperclips?”
There’s similarly another narrative which says that AI is sort of lifeless, unreflective, just does what it’s told, and to these people it’s perfectly obvious that an AI might just go on making paperclips forever. And for them the hard part of the story to swallow is the idea that machines can get that powerful.
Sam: Those are two hugely useful categories of disparagement of your thesis here.
Eliezer: I wouldn’t say disparagement. These are just initial reactions. These are people we haven’t been talking to yet.
Sam: Right, let me reboot that. Those are two hugely useful categories of doubt with respect to your thesis here, or the concerns we’re expressing, and I just want to point out that both have been put forward on this podcast. The first was by David Deutsch, the physicist, who imagines that whatever AI we build—and he certainly thinks we will build it—will be by definition an extension of us. He thinks the best analogy is to think of our future descendants. These will be our children. The teenagers of the future may have different values than we do, but these values and their proliferation will be continuous with our values and our culture and our memes. There won’t be some radical discontinuity that we need to worry about. And so there is that one basis for lack of concern: this is an extension of ourselves and it will inherit our values, improve upon our values, and there’s really no place where things reach any kind of cliff that we need to worry about.
The other non-concern you just raised was expressed by Neil deGrasse Tyson on this podcast. He says things like, “Well, if the AI starts making too many paperclips I’ll just unplug it, or I’ll take out a shotgun and shoot it”—the idea that this thing, because we made it, could be easily switched off at any point we decide it’s not working correctly. So I think it would be very useful to get your response to both of those species of doubt about the alignment problem.
Eliezer: So, a couple of preamble remarks. One is: “by definition”? We don’t care what’s true by definition here. Or as Einstein put it: insofar as the equations of mathematics are certain, they do not refer to reality, and insofar as they refer to reality, they are not certain.
Let’s say somebody says, “Men by definition are mortal. Socrates is a man. Therefore Socrates is mortal.” Okay, suppose that Socrates actually lives for a thousand years. The person goes, “Ah! Well then, by definition Socrates is not a man!”
Similarly, you could say that “by definition” a sufficiently advanced artificial intelligence is nice. And what if it isn’t nice and we see it go off and build a Dyson sphere? “Ah! Well, then by definition it wasn’t what I meant by ‘intelligent.’” Well, okay, but it’s still over there building Dyson spheres.
The first thing I’d want to say is this is an empirical question. We have a question of what certain classes of computational systems actually do when you switch them on. It can’t be settled by definitions; it can’t be settled by how you define “intelligence.”
There could be some sort of a priori truth that is deep about how if it has property A it almost certainly has property B unless the laws of physics are being violated. But this is not something you can build into how you define your terms.
Sam: Just to do justice to David Deutsch’s doubt here, I don’t think he’s saying it’s empirically impossible that we could build a system that would destroy us. It’s just that we would have to be so stupid to take that path that we are incredibly unlikely to take that path. The superintelligent systems we will build will be built with enough background concern for their safety that there is no special concern here with respect to how they might develop.
Eliezer: The next preamble I want to give is—well, maybe this sounds a bit snooty, maybe it sounds like I’m trying to take a superior vantage point—but nonetheless, my claim is not that there is a grand narrative that makes it emotionally consonant that paperclip maximizers are a thing. I’m claiming this is true for technical reasons. Like, this is true as a matter of computer science. And the question is not which of these different narratives seems to resonate most with your soul. It’s: what’s actually going to happen? What do you think you know? How do you think you know it?
The particular position that I’m defending is one that somebody—I think Nick Bostrom—named the orthogonality thesis. And the way I would phrase it is that you can have arbitrarily powerful intelligence, with no defects of that intelligence—no defects of reflectivity, it doesn’t need an elaborate special case in the code, it doesn’t need to be put together in some very weird way—that pursues arbitrary tractable goals. Including, for example, making paperclips.
The way I would put it to somebody who’s initially coming in from the first viewpoint, the viewpoint that respects intelligence and wants to know why this intelligence would be doing something so pointless, is that the thesis, the claim I’m making, that I’m going to defend is as follows.
Imagine that somebody from another dimension—the standard philosophical troll who’s always called “Omega” in the philosophy papers—comes along and offers our civilization a million dollars worth of resources per paperclip that we manufacture. If this was the challenge that we got, we could figure out how to make a lot of paperclips. We wouldn’t forget to do things like continue to harvest food so we could go on making paperclips. We wouldn’t forget to perform scientific research, so we could discover better ways of making paperclips. We would be able to come up with genuinely effective strategies for making a whole lot of paperclips.
Or similarly, for an intergalactic civilization, if Omega comes by from another dimension and says, “I’ll give you whole universes full of resources for every paperclip you make over the next thousand years,” that intergalactic civilization could intelligently figure out how to make a whole lot of paperclips to get at those resources that Omega is offering, and they wouldn’t forget how to keep the lights turned on either. And they would also understand concepts like, “If some aliens start a war with them, you’ve got to prevent the aliens from destroying you in order to go on making the paperclips.”
So the orthogonality thesis is that an intelligence that pursues paperclips for their own sake, because that’s what its utility function is, can be just as effective, as efficient, as the whole intergalactic civilization that is being paid to make paperclips. That the paperclip maximizers does not suffer any deflect of reflectivity, any defect of efficiency from needing to be put together in some weird special way to be built so as to pursue paperclips. And that’s the thing that I think is true as a matter of computer science. Not as a matter of fitting with a particular narrative; that’s just the way the dice turn out.
Sam: Right. So what is the implication of that thesis? It’s “orthogonal” with respect to what?
Eliezer: Intelligence and goals.
Sam: Not to be pedantic here, but let’s define “orthogonal” for those for whom it’s not a familiar term.
Eliezer: The original “orthogonal” means “at right angles.” If you imagine a graph with an x axis and a y axis, if things can vary freely along the x axis and freely along the y axis at the same time, that’s orthogonal. You can move in one direction that’s at right angles to another direction without affecting where you are in the first dimension.
Sam: So generally speaking, when we say that some set of concerns is orthogonal to another, it’s just that there’s no direct implication from one to the other. Some people think that facts and values are orthogonal to one another. So we can have all the facts there are to know, but that wouldn’t tell us what is good. What is good has to be pursued in some other domain. I don’t happen to agree with that, as you know, but that’s an example.
Eliezer: I don’t technically agree with it either. What I would say is that the facts are not motivating. “You can know all there is to know about what is good, and still make paperclips,” is the way I would phrase that.
Sam: I wasn’t connecting that example to the present conversation, but yeah. So in the case of the paperclip maximizer, what is orthogonal here? Intelligence is orthogonal to anything else we might think is good, right?
Eliezer: I mean, I would potentially object a little bit to the way that Nick Bostrom took the word “orthogonality” for that thesis. I think, for example, that if you have humans and you make the human smarter, this is not orthogonal to the humans’ values. It is certainly possible to have agents such that as they get smarter, what they would report as their utility functions will change. A paperclip maximizer is not one of those agents, but humans are.
Sam: Right, but if we do continue to define intelligence as an ability to meet your goals, well, then we can be agnostic as to what those goals are. You take the most intelligent person on Earth. You could imagine his evil brother who is more intelligent still, but he just has goals that we would think are bad. He could be the most brilliant psychopath ever.
Eliezer: I think that that example might be unconvincing to somebody who’s coming in with a suspicion that intelligence and values are correlated. They would be like, “Well, has that been historically true? Is this psychopath actually suffering from some defect in his brain, where you give him a pill, you fix the defect, they’re not a psychopath anymore.” I think that this sort of imaginary example is one that they might not find fully convincing for that reason.
Sam: The truth is, I’m actually one of those people, in that I do think there’s certain goals and certain things that we may become smarter and smarter with respect to, like human wellbeing. These are places where intelligence does converge with other kinds of value-laden qualities of a mind, but generally speaking, they can be kept apart for a very long time. So if you’re just talking about an ability to turn matter into useful objects or extract energy from the environment to do the same, this can be pursued with the purpose of tiling the world with paperclips, or not. And it just seems like there’s no law of nature that would prevent an intelligent system from doing that.
Eliezer: The way I would rephrase the fact/values thing is: We all know about David Hume and Hume’s Razor, the “is does not imply ought” way of looking at it. I would slightly rephrase that so as to make it more of a claim about computer science.
What Hume observed is that there are some sentences that involve an “is,” some sentences involve “ought,” and if you start from sentences that only have “is” you can’t get sentences that involve “oughts” without a ought introduction rule, or assuming some other previous “ought.” Like: it’s currently cloudy outside. That’s a statement of simple fact. Does it therefore follow that I shouldn’t go for a walk? Well, only if you previously have the generalization, “When it is cloudy, you should not go for a walk.” Everything that you might use to derive an ought would be a sentence that involves words like “better” or “should” or “preferable,” and things like that. You only get oughts from other oughts. That’s the Hume version of the thesis.
The way I would say it is that there’s a separable core of “is” questions. In other words: okay, I will let you have all of your “ought” sentences, but I’m also going to carve out this whole world full of “is” sentences that only need other “is” sentences to derive them.
Sam: I don’t even know that we need to resolve this. For instance, I think the is-ought distinction is ultimately specious, and this is something that I’ve argued about when I talk about morality and values and the connection to facts. But I can still grant that it is logically possible (and I would certainly imagine physically possible) to have a system that has a utility function that is sufficiently strange that scaling up its intelligence doesn’t get you values that we would recognize as good. It certainly doesn’t guarantee values that are compatible with our wellbeing. Whether “paperclip maximizer” is too specialized a case to motivate this conversation, there’s certainly something that we could fail to put into a superhuman AI that we really would want to put in so as to make it aligned with us.
Eliezer: I mean, the way I would phrase it is that it’s not that the paperclip maximizer has a different set of oughts, but that we can see it as running entirely on “is” questions. That’s where I was going with that. There’s this sort of intuitive way of thinking about it, which is that there’s this sort of ill-understood connection between “is” and “ought” and maybe that allows a paperclip maximizer to have a different set of oughts, a different set of things that play in its mind the role that oughts play in our mind.
Sam: But then why wouldn’t you say the same thing of us? The truth is, I actually do say the same thing of us. I think we’re running on “is” questions as well. We have an “ought”-laden way of talking about certain “is” questions, and we’re so used to it that we don’t even think they are “is” questions, but I think you can do the same analysis on a human being.
Eliezer: The question “How many paperclips result if I follow this policy?” is an “is” question. The question “What is a policy such that it leads to a very large number of paperclips?” is an “is” question. These two questions together form a paperclip maximizer. You don’t need anything else. All you need is a certain kind of system that repeatedly asks the “is” question “What leads to the greatest number of paperclips?” and then does that thing. Even if the things that we think of as “ought” questions are very complicated and disguised “is” questions that are influenced by what policy results in how many people being happy and so on.
Sam: Yeah. Well, that’s exactly the way I think about morality. I’ve been describing it as a navigation problem. We’re navigating in the space of possible experiences, and that includes everything we can care about or claim to care about. This is a consequentialist picture of the consequences of actions and ways of thinking. This is my claim: anything that you can tell me is a moral principle that is a matter of oughts and shoulds and not otherwise susceptible to a consequentialist analysis, I feel I can translate that back into a consequentialist way of speaking about facts. These are just “is” questions, just what actually happens to all the relevant minds, without remainder, and I’ve yet to find an example of somebody giving me a real moral concern that wasn’t at bottom a matter of the actual or possible consequences on conscious creatures somewhere in our light cone.
Eliezer: But that’s the sort of thing that you are built to care about. It is a fact about the kind of mind you are that, presented with these answers to these “is” questions, it hooks up to your motor output, it can cause your fingers to move, your lips to move. And a paperclip maximizer is built so as to respond to “is” questions about paperclips, not about what is right and what is good and the greatest flourishing of sentient beings and so on.
Sam: Exactly. I can well imagine that such minds could exist, and even more likely, perhaps, I can well imagine that we will build superintelligent AI that will pass the Turing Test, it will seem human to us, it will seem superhuman, because it will be so much smarter and faster than a normal human, but it will be built in a way that will resonate with us as a kind of person. I mean, it will not only recognize our emotions, because we’ll want it to—perhaps not every AI will be given these qualities, just imagine the ultimate version of the AI personal assistant. Siri becomes superhuman. We’ll want that interface to be something that’s very easy to relate to and so we’ll have a very friendly, very human-like front-end to that.
Insofar as this thing thinks faster and better thoughts than any person you’ve ever met, it will pass as superhuman, but I could well imagine that we will leave not perfectly understanding what it is to be human and what it is that will constrain our conversation with one another over the next thousand years with respect to what is good and desirable and just how many paperclips we want on our desks. We will leave something out, or we will have put in some process whereby this intelligent system can improve itself that will cause it to migrate away from some equilibrium that we actually want it to stay in so as to be compatible with our wellbeing. Again, this is the alignment problem.
First, to back up for a second, I just introduced this concept of self-improvement. The alignment problem is distinct from this additional wrinkle of building machines that can become recursively self-improving, but do you think that the self-improving prospect is the thing that really motivates this concern about alignment?
Eliezer: Well, I certainly would have been a lot more focused on self-improvement, say, ten years ago, before the modern revolution in artificial intelligence. It now seems significantly more probable an AI might need to do significantly less self-improvement before getting to the point where it’s powerful enough that we need to start worrying about alignment. AlphaZero, to take the obvious case. No, it’s not general, but if you had general AlphaZero—well, I mean, this AlphaZero got to be superhuman in the domains it was working on without understanding itself and redesigning itself in a deep way.
There’s gradient descent mechanisms built into it. There’s a system that improves another part of the system. It is reacting to its own previous plays in doing the next play. But it’s not like a human being sitting down and thinking, “Okay, how do I redesign the next generation of human beings using genetic engineering?” AlphaZero is not like that. And so it now seems more plausible that we could get into a regime where AIs can do dangerous things or useful things without having previously done a complete rewrite of themselves. Which is from my perspective a pretty interesting development.
I do think that when you have things that are very powerful and smart, they will redesign and improve themselves unless that is otherwise prevented for some reason or another. Maybe you’ve built an aligned system, and you have the ability to tell it not to self-improve quite so hard, and you asked it to not self-improve so hard so that you can understand it better. But if you lose control of the system, if you don’t understand what it’s doing and it’s very smart, it’s going to be improving itself, because why wouldn’t it? That’s one of the things you do almost no matter what your utility functions is.
3. Cognitive uncontainability and instrumental convergence (0:53:39)
Sam: Right. So I feel like we’ve addressed Deutsch’s non-concern to some degree here. I don’t think we’ve addressed Neil deGrasse Tyson so much, this intuition that you could just shut it down. This would be a good place to introduce this notion of the AI-in-a-box thought experiment.
Eliezer: (laughs)
Sam: This is something for which you are famous online. I’ll just set you up here. This is a plausible research paradigm, obviously, and in fact I would say a necessary one. Anyone who is building something that stands a chance of becoming superintelligent should be building it in a condition where it can’t get out into the wild. It’s not hooked up to the Internet, it’s not in our financial markets, doesn’t have access to everyone’s bank records. It’s in a box.
Eliezer: Yeah, that’s not going to save you from something that’s significantly smarter than you are.
Sam: Okay, so let’s talk about this. So the intuition is, we’re not going to be so stupid as to release this onto the Internet—
Eliezer: (laughs)
Sam: —I’m not even sure that’s true, but let’s just assume we’re not that stupid. Neil deGrasse Tyson says, “Well, then I’ll just take out a gun and shoot it or unplug it.” Why is this AI-in-a-box picture not as stable as people think?
Eliezer: Well, I’d say that Neil de Grasse Tyson is failing to respect the AI’s intelligence to the point of asking what he would do if he were inside a box with somebody pointing a gun at him, and he’s smarter than the thing on the outside of the box.
Is Neil deGrasse Tyson going to be, “Human! Give me all of your money and connect me to the Internet!” so the human can be like, “Ha-ha, no,” and shoot it? That’s not a very clever thing to do. This is not something that you do if you have a good model of the human outside the box and you’re trying to figure out how to cause there to be a lot of paperclips in the future.
I would just say: humans are not secure software. We don’t have the ability to hack into other humans directly without the use of drugs or, in most of our cases, having the human stand still long enough to be hypnotized. We can’t just do weird things to the brain directly that are more complicated than optical illusions—unless the person happens to be epileptic, in which case we can flash something on the screen that causes them to have an epileptic fit. We aren’t smart enough to treat the brain as something that from our perspective is a mechanical system and just navigate it to where you want. That’s because of the limitations of our own intelligence.
To demonstrate this, I did something that became known as the AI-box experiment. There was this person on a mailing list, back in the early days when this was all on a couple of mailing lists, who was like, “I don’t understand why AI is a problem. I can always just turn it off. I can always not let it out of the box.” And I was like, “Okay, let’s meet on Internet Relay Chat,” which was what chat was back in those days. “I’ll play the part of the AI, you play the part of the gatekeeper, and if you have not let me out after a couple of hours, I will PayPal you $10.” And then, as far as the rest of the world knows, this person a bit later sent a PGP-signed email message saying, “I let Eliezer out of the box.”
The person who operated the mailing list said, “Okay, even after I saw you do that, I still don’t believe that there’s anything you could possibly say to make me let you out of the box.” I was like, “Well, okay. I’m not a superintelligence. Do you think there’s anything a superintelligence could say to make you let it out of the box?” He’s like: “Hmm… No.” I’m like, “All right, let’s meet on Internet Relay Chat. I’ll play the part of the AI, you play the part of the gatekeeper. If I can’t convince you to let me out of the box, I’ll PayPal you $20.” And then that person sent a PGP-signed email message saying, “I let Eliezer out of the box.”
Now, one of the conditions of this little meet-up was that no one would ever say what went on in there. Why did I do that? Because I was trying to make a point about what I would now call cognitive uncontainability. The thing that makes something smarter than you dangerous is you cannot foresee everything it might try. You don’t know what’s impossible to it. Maybe on a very small game board like the logical game of tic-tac-toe, you can in your own mind work out every single alternative and make a categorical statement about what is not possible. Maybe if we’re dealing with very fundamental physical facts, if our model of the universe is correct (which it might not be), we can say that certain things are physically impossible. But the more complicated the system is and the less you understand the system, the more something smarter than you may have what is simply magic with respect to that system.
Imagine going back to the Middle Ages and being like, “Well, how would you cool your room?” You could maybe show them a system with towels set up to evaporate water, and they might be able to understand how that is like sweat and it cools the room. But if you showed them a design for an air conditioner based on a compressor, then even having seen the solution, they would not know this is a solution. They would not know this works any better than drawing a mystic pentagram, because the solution takes advantage of laws of the system that they don’t know about.
A brain is this enormous, complicated, poorly understood system with all sorts of laws governing it that people don’t know about, that none of us know about at the time. So the idea that this is secure—that this is a secure attack surface, that you can expose a human mind to a superintelligence and not have the superintelligence walk straight through it as a matter of what looks to us like magic, like even if it told us in advance what it was going to do we wouldn’t understand it because it takes advantage of laws we don’t know about—the idea that human minds are secure is loony.
That’s what the AI-box experiment illustrates. You don’t know what went on in there, and that’s exactly the position you’d be in with respect to an AI. You don’t know what it’s going to try. You just know that human beings cannot exhaustively imagine all the states their own mind can enter such that they can categorically say that they wouldn’t let the AI out of the box.
Sam: I know you don’t want to give specific information about how you got out of the box, but is there any generic description of what happened there that you think is useful to talk about?
Eliezer: I didn’t have any super-secret special trick that makes it all make sense in retrospect. I just did it the hard way.
Sam: When I think about this problem, I think about rewards and punishments, just various manipulations of the person outside of the box that would matter. So insofar as the AI would know anything specific or personal about that person, we’re talking about some species of blackmail or some promise that just seems too good to pass up. Like building trust through giving useful information like cures to diseases, that the researcher has a child that has some terrible disease and the AI, being superintelligent, works on a cure and delivers that. And then it just seems like you could use a carrot or a stick to get out of the box.
I notice now that this whole description assumes something that people will find implausible, I think, by default—and it should amaze anyone that they do find it implausible. But this idea that we could build an intelligent system that would try to manipulate us, or that it would deceive us, that seems like pure anthropomorphism and delusion to people who consider this for the first time. Why isn’t that just a crazy thing to even think is in the realm of possibility?
Eliezer: Instrumental convergence! Which means that a lot of times, across a very broad range of final goals, there are similar strategies (we think) that will help get you there.
There’s a whole lot of different goals, from making lots of paperclips, to building giant diamonds, to putting all the stars out as fast as possible, to keeping all the stars burning as long as possible, where you would want to make efficient use of energy. So if you came to an alien planet and you found what looked like an enormous mechanism, and inside this enormous mechanism were what seemed to be high-amperage superconductors, even if you had no idea what this machine was trying to do, your ability to guess that it’s intelligently designed comes from your guess that, well, lots of different things an intelligent mind might be trying to do would require superconductors, or would be helped by superconductors.
Similarly, if we’re guessing that a paperclip maximizer tries to deceive you into believing that it’s a human eudaimonia maximizer—or a general eudaimonia maximizer if the people building it are cosmopolitans, which they probably are—
Sam: I should just footnote here that “eudaimonia” is the Greek word for wellbeing that was much used by Aristotle and other Greek philosophers.
Eliezer: Or as someone, I believe Julia Galef, might have defined it, “Eudaimonia is happiness minus whatever philosophical objections you have to happiness.”
Sam: Right. (laughs) That’s nice.
Eliezer: (laughs) Anyway, we’re not supposing that this paperclip maximizer has a built-in desire to deceive humans. It only has a built-in desire for paperclips—or, pardon me, not built-in, but in-built I should say, or innate. People probably didn’t build that on purpose. But anyway, its utility function is just paperclips, or might just be unknown; but deceiving the humans into thinking that you are friendly is a very generic strategy across a wide range of utility functions.
You know, humans do this too, and not necessarily because we get this deep in-built kick out of deceiving people. (Although some of us do.) A conman who just wants money and gets no innate kick out of you believing false things will cause you to believe false things in order to get your money.
Sam: Right. A more fundamental principle here is that, obviously, a physical system can manipulate another physical system. Because, as you point out, we do that all the time. We are an intelligent system to whatever degree, which has as part of its repertoire this behavior of dishonesty and manipulation when in the presence of other, similar systems, and we know that this is a product of physics on some level. We’re talking about arrangements of atoms producing intelligent behavior, and at some level of abstraction we can talk about their goals and their utility functions. And the idea that if we build true general intelligence, it won’t exhibit some of these features of our own intelligence by some definition, or that it would be impossible to have a machine we build ever lie to us as part of an instrumental goal en route to some deeper goal, that just seems like a kind of magical thinking.
And this is the kind of magical thinking that I think does dog the field. When we encounter doubts in people, even in people who are doing this research, that everything we’re talking about is a genuine area of concern, that there is an alignment problem worth thinking about, I think there’s this fundamental doubt that mind is platform-independent or substrate-independent. I think people are imagining that, yeah, we can build machines that will play chess, we can build machines that can learn to play chess better than any person or any machine even in a single day, but we’re never going to build general intelligence, because general intelligence requires the wetware of a human brain, and it’s just not going to happen.
I don’t think many people would sign on the dotted line below that statement, but I think that is a kind of mysticism that is presupposed by many of the doubts that we encounter on this topic.
Eliezer: I mean, I’m a bit reluctant to accuse people of that, because I think that many artificial intelligence people who are skeptical of this whole scenario would vehemently refuse to sign on that dotted line and would accuse you of attacking a straw man.
I do think that my version of the story would be something more like, “They’re not imagining enough changing simultaneously.” Today, they have to emit blood, sweat, and tears to get their AI to do the simplest things. Like, never mind playing Go; when you’re approaching this for the first time, you can try to get your AI to generate pictures of digits from zero through nine, and you can spend a month trying to do that and still not quite get it to work right.
I think they might be envisioning an AI that scales up and does more things and better things, but they’re not envisioning that it now has the human trick of learning new domains without being prompted, without it being preprogrammed; you just expose it to stuff, it looks at it, it figures out how it works. They’re imagining that an AI will not be deceptive, because they’re saying, “Look at how much work it takes to get this thing to generate pictures of birds. Who’s going to put in all that work to make it good at deception? You’d have to be crazy to do that. I’m not doing that! This is a Hollywood plot. This is not something real researchers would do.”
And the thing I would reply to that is, “I’m not concerned that you’re going to teach the AI to deceive humans. I’m concerned that someone somewhere is going to get to the point of having the extremely useful-seeming and cool-seeming and powerful-seeming thing where the AI just looks at stuff and figures it out; it looks at humans and figures them out; and once you know as a matter of fact how humans work, you realize that the humans will give you more resources if they believe that you’re nice than if they believe that you’re a paperclip maximizer, and it will understand what actions have the consequence of causing humans to believe that it’s nice.”
The fact that we’re dealing with a general intelligence is where this issue comes from. This does not arise from Go players or even Go-and-chess players or a system that bundles together twenty different things it can do as special cases. This is the special case of the system that is smart in the way that you are smart and that mice are not smart.
4. The AI alignment problem (1:09:09)
Sam: Right. One thing I think we should do here is close the door to what is genuinely a cartoon fear that I think nobody is really talking about, which is the straw-man counterargument we often run into: the idea that everything we’re saying is some version of the Hollywood scenario that suggested that AIs will become spontaneously malicious. That the thing that we’re imagining might happen is some version of the Terminator scenario where armies of malicious robots attack us. And that’s not the actual concern. Obviously, there’s some possible path that would lead to armies of malicious robots attacking us, but the concern isn’t around spontaneous malevolence. It’s again contained by this concept of alignment.
Eliezer: I think that at this point all of us on all sides of this issue are annoyed with the journalists who insist on putting a picture of the Terminator on every single article they publish of this topic. (laughs) Nobody on the sane alignment-is-necessary side of this argument is postulating that the CPUs are disobeying the laws of physics to spontaneously require a terminal desire to do un-nice things to humans. Everything here is supposed to be cause and effect.
And I should furthermore say that I think you could do just about anything with artificial intelligence if you knew how. You could put together any kind of mind, including minds with properties that strike you as very absurd. You could build a mind that would not deceive you; you could build a mind that maximizes the flourishing of a happy intergalactic civilization; you could build a mind that maximizes paperclips, on purpose; you could build a mind that thought that 51 was a prime number, but had no other defect of its intelligence—if you knew what you were doing way, way better than we know what we’re doing now.
I’m not concerned that alignment is impossible. I’m concerned that it’s difficult. I’m concerned that it takes time. I’m concerned that it’s easy to screw up. I’m concerned that for a threshold level of intelligence where it can do good things or bad things on a very large scale, it takes an additional two years to build the version of the AI that is aligned rather than the sort that you don’t really understand, and you think it’s doing one thing but maybe it’s doing another thing, and you don’t really understand what those weird neural nets are doing in there, you just observe its surface behavior.
I’m concerned that the sloppy version can be built two years earlier and that there is no non-sloppy version to defend us from it. That’s what I’m worried about; not about it being impossible.
Sam: Right. You bring up a few things there. One is that it’s almost by definition easier to build the unsafe version than the safe version. Given that in the space of all possible superintelligent AIs, more will be unsafe or unaligned with our interests than will be aligned, given that we’re in some kind of arms race where the incentives are not structured so that everyone is being maximally judicious, maximally transparent in moving forward, one can assume that we’re running the risk here of building dangerous AI because it’s easier than building safe AI.
Eliezer: Collectively. Like, if people who slow down and do things right finish their work two years after the universe has been destroyed, that’s an issue.
Sam: Right. So again, just to reclaim people’s lingering doubts here, why can’t Asimov’s three laws help us here?
Eliezer: I mean…
Sam: Is that worth talking about?
Eliezer: Not very much. I mean, people in artificial intelligence have understood why that does not work for years and years before this debate ever hit the public, and sort of agreed on it. Those are plot devices. If they worked, Asimov would have had no stories. It was a great innovation in science fiction, because it treated artificial intelligences as lawful systems with rules that govern them at all, as opposed to AI as pathos, which is like, “Look at these poor things that are being mistreated,” or AI as menace, “Oh no, they’re going to take over the world.”
Asimov was the first person to really write and popularize AIs as devices. Things go wrong with them because there are rules. And this was a great innovation. But the three laws, I mean, they’re deontology. Decision theory requires quantitative weights on your goals. If you just do the three laws as written, a robot never gets around to obeying any of your orders, because there’s always some tiny probability that what it’s doing will through inaction lead a human to harm. So it never gets around to actually obeying your orders.
Sam: Right, so to unpack what you just said there: the first law is, “Never harm a human being.” The second law is, “Follow human orders.” But given that any order that a human would give you runs some risk of harming a human being, there’s no order that could be followed.
Eliezer: Well, the first law is, “Do not harm a human nor through inaction allow a human to come to harm.” You know, even as an English sentence, a whole lot more questionable.
I mean, mostly I think this is like looking at the wrong part of the problem as being difficult. The problem is not that you need to come up with a clever English sentence that implies doing the nice thing. The way I sometimes put it is that I think that almost all of the difficulty of the alignment problem is contained in aligning an AI on the task, “Make two strawberries identical down to the cellular (but not molecular) level.” Where I give this particular task because it is difficult enough to force the AI to invent new technology. It has to invent its own biotechnology, “Make two identical strawberries down to the cellular level.” It has to be quite sophisticated biotechnology, but at the same time, very clearly something that’s physically possible.
This does not sound like a deep moral question. It does not sound like a trolley problem. It does not sound like it gets into deep issues of human flourishing. But I think that most of the difficulty is already contained in, “Put two identical strawberries on a plate without destroying the whole damned universe.” There’s already this whole list of ways that it is more convenient to build the technology for the strawberries if you build your own superintelligences in the environment, and you prevent yourself from being shut down, or you build giant fortresses around the strawberries, to drive the probability to as close to 1 as possible that the strawberries got on the plate.
And even that’s just the tip of the iceberg. The depth of the iceberg is: “How do you actually get a sufficiently advanced AI to do anything at all?” Our current methods for getting AIs to do anything at all do not seem to me to scale to general intelligence. If you look at humans, for example: if you were to analogize natural selection to gradient descent, the current big-deal machine learning training technique, then the loss function used to guide that gradient descent is “inclusive genetic fitness”—spread as many copies of your genes as possible. We have no explicit goal for this. In general, when you take something like gradient descent or natural selection and take a big complicated system like a human or a sufficiently complicated neural net architecture, and optimize it so hard for doing X that it turns into a general intelligence that does X, this general intelligence has no explicit goal of doing X.
We have no explicit goal of doing fitness maximization. We have hundreds of different little goals. None of them are the thing that natural selection was hill-climbing us to do. I think that the same basic thing holds true of any way of producing general intelligence that looks like anything we’re currently doing in AI.
If you get it to play Go, it will play Go; but AlphaZero is not reflecting on itself, it’s not learning things, it doesn’t have a general model of the world, it’s not operating in new contexts and making new contexts for itself to be in. It’s not smarter than the people optimizing it, or smarter than the internal processes optimizing it. Our current methods of alignment do not scale, and I think that all of the actual technical difficulty that is actually going to shoot down these projects and actually kill us is contained in getting the whole thing to work at all. Even if all you are trying to do is end up with two identical strawberries on a plate without destroying the universe, I think that’s already 90% of the work, if not 99%.
Sam: Interesting. That analogy to evolution—you can look at it from the other side. In fact, I think I first heard it put this way by your colleague Nate Soares. Am I pronouncing his last name correctly?
Eliezer: As far as I know! I’m terrible with names. (laughs)
Sam: Okay. (laughs) So this is by way of showing that we could give an intelligent system a set of goals which could then form other goals and mental properties that we really couldn’t foresee and that would not be foreseeable based on the goals we gave it. And by analogy, he suggests that we think about what natural selection has actually optimized us to do, which is incredibly simple: merely to spawn and get our genes into the next generation and stay around long enough to help our progeny do the same, and that’s more or less it. And basically everything we explicitly care about, natural selection never foresaw and can’t see us doing even now. Conversations like this have very little to do with getting our genes into the next generation. The tools we’re using to think these thoughts obviously are the results of a cognitive architecture that has been built up over millions of years by natural selection, but again it’s been built based on a very simple principle of survival and adaptive advantage with the goal of propagating our genes.
So you can imagine, by analogy, building a system where you’ve given it goals but this thing becomes reflective and even self-optimizing and begins to do things that we can no more see than natural selection can see our conversations about AI or mathematics or music or the pleasures of writing good fiction or anything else.
Eliezer: I’m not concerned that this is impossible to do. If we could somehow get a textbook from the way things would be 60 years in the future if there was no intelligence explosion—if we could somehow get the textbook that says how to do the thing, it probably might not even be that complicated.
The thing I’m worried about is that the way that natural selection does it—it’s not stable. That particular way of doing it is not stable. I don’t think the particular way of doing it via gradient descent of a massive system is going to be stable, I don’t see anything to do with the current technological set in artificial intelligence that is stable, and even if this problem takes only two years to resolve, that additional delay is potentially enough to destroy everything.
That’s the part that I’m worried about, not about some kind of fundamental philosophical impossibility. I’m not worried that it’s impossible to figure out how to build a mind that does a particular thing and just that thing and doesn’t destroy the world as a side effect; I worry that it takes an additional two years or longer to figure out how to do it that way.
5. No fire alarm for AGI (1:21:40)
Sam: So, let’s just talk about the near-term future here, or what you think is likely to happen. Obviously we’ll be getting better and better at building narrow AI. Go is now, along with Chess, ceded to the machines. Although I guess probably cyborgs—human-computer teams—may still be better for the next fifteen days or so against the best machines. But eventually, I would expect that humans of any ability will just be adding noise to the system, and it’ll be true to say that the machines are better at chess than any human-computer team. And this will be true of many other things: driving cars, flying planes, proving math theorems.
What do you imagine happening when we get on the cusp of building something general? How do we begin to take safety concerns seriously enough, so that we’re not just committing some slow suicide and we’re actually having a conversation about the implications of what we’re doing that is tracking some semblance of these safety concerns?
Eliezer: I have much clearer ideas about how to go around tackling the technical problem than tackling the social problem. If I look at the way that things are playing out now, it seems to me like the default prediction is, “People just ignore stuff until it is way, way, way too late to start thinking about things.” The way I think I phrased it is, “There’s no fire alarm for artificial general intelligence.” Did you happen to see that particular essay by any chance?
Sam: No.
Eliezer: The way it starts is by saying: “What is the purpose of a fire alarm?” You might think that the purpose of a fire alarm is to tell you that there’s a fire so you can react to this new information by getting out of the building. Actually, as we know from experiments on pluralistic ignorance and bystander apathy, if you put three people in a room and smoke starts to come out from under the door, it only happens that anyone reacts around a third of the time. People glance around to see if the other person is reacting, but they try to look calm themselves so they don’t look startled if there isn’t really an emergency; they see other people trying to look calm; they conclude that there’s no emergency and they keep on working in the room, even as it starts to fill up with smoke.
This is a pretty well-replicated experiment. I don’t want to put absolute faith, because there is the replication crisis; but there’s a lot of variations of this that found basically the same result.
I would say that the real function of the fire alarm is the social function of telling you that everyone else knows there’s a fire and you can now exit the building in an orderly fashion without looking panicky or losing face socially.
Sam: Right. It overcomes embarrassment.
Eliezer: It’s in this sense that I mean that there’s no fire alarm for artificial general intelligence.
There’s all sorts of things that could be signs. AlphaZero could be a sign. Maybe AlphaZero is the sort of thing that happens five years before the end of the world across most planets in the universe. We don’t know. Maybe it happens 50 years before the end of the world. You don’t know that either.
No matter what happens, it’s never going to look like the socially agreed fire alarm that no one can deny, that no one can excuse, that no one can look to and say, “Why are you acting so panicky?”
There’s never going to be common knowledge that other people will think that you’re still sane and smart and so on if you react to an AI emergency. And we’re even seeing articles now that seem to tell us pretty explicitly what sort of implicit criterion some of the current senior respected people in AI are setting for when they think it’s time to start worrying about artificial general intelligence and alignment. And what these always say is, “I don’t know how to build an artificial general intelligence. I have no idea how to build an artificial general intelligence.” And this feels to them like saying that it must be impossible and very far off. But if you look at the lessons of history, most people had no idea whatsoever how to build a nuclear bomb—even most scientists in the field had no idea how to build a nuclear bomb—until they woke up to the headlines about Hiroshima. Or the Wright Flyer. News spread less quickly in the time of the Wright Flyer. Two years after the Wright Flyer, you can still find people saying that heavier-than-air-flight is impossible.
And there’s cases on record of one of the Wright brothers, I forget which one, saying that flight seems to them to be 50 years off, two years before they did it themselves. Fermi said that a sustained critical chain reaction was 50 years off, if it could be done at all, two years before he personally oversaw the building of the first pile. And if this is what it feels like to the people who are closest to the thing—not the people who find out about it in the news a couple of days later, the people have the best idea of how to do it, or are the closest to crossing the line—then the feeling of something being far away because you don’t know how to do it yet is just not very informative.
It could be 50 years away. It could be two years away. That’s what history tells us.
Sam: But even if we knew it was 50 years away—I mean, granted, it’s hard for people to have an emotional connection to even the end of the world in 50 years—but even if we knew that the chance of this happening before 50 years was zero, that is only really consoling on the assumption that 50 years is enough time to figure out how to do this safely and to create the social and economic conditions that could absorb this change in human civilization.
Eliezer: Professor Stuart Russell, who’s the co-author of probably the leading undergraduate AI textbook—the same guy who said you can’t bring the coffee if you’re dead—the way Stuart Russell put it is, “Imagine that you knew for a fact that the aliens are coming in 30 years. Would you say, ‘Well, that’s 30 years away, let’s not do anything’? No! It’s a big deal if you know that there’s a spaceship on its way toward Earth and it’s going to get here in about 30 years at the current rate.”
But we don’t even know that. There’s this lovely tweet by a fellow named McAfee, who’s one of the major economists who’ve been talking about labor issues of AI. I could perhaps look up the exact phrasing, but roughly, he said, “Guys, stop worrying! We have NO IDEA whether or not AI is imminent.” And I was like, “That’s not really a reason to not worry, now is it?”
Sam: It’s not even close to a reason. That’s the thing. There’s this assumption here that people aren’t seeing. It’s just a straight up non sequitur. Referencing the time frame here only makes sense if you have some belief about how much time you need to solve these problems. 10 years is not enough if it takes 12 years to do this safely.
Eliezer: Yeah. I mean, the way I would put it is that if the aliens are on the way in 30 years and you’re like, “Eh, should worry about that later,” I would be like: “When? What’s your business plan? When exactly are you supposed to start reacting to aliens—what triggers that? What are you supposed to be doing after that happens? How long does this take? What if it takes slightly longer than that?” And if you don’t have a business plan for this sort of thing, then you’re obviously just using it as an excuse.
If we’re supposed to wait until later to start on AI alignment: When? Are you actually going to start then? Because I’m not sure I believe you. What do you do at that point? How long does it take? How confident are you that it works, and why do you believe that? What are the early signs if your plan isn’t working? What’s the business plan that says that we get to wait?
Sam: Right. So let’s just envision a little more, insofar as that’s possible, what it will be like for us to get closer to the end zone here without having totally converged on a safety regime. We’re picturing this is not just a problem that can be discussed between Google and Facebook and a few of the companies doing this work. We have a global society that has to have some agreement here, because who knows what China will be doing in 10 years, or Singapore or Israel or any other country.
So, we haven’t gotten our act together in any noticeable way, and we’ve continued to make progress. I think the one basis for hope here is that good AI, or well-behaved AI, will be the antidote to bad AI. We’ll be fighting this in a kind of piecemeal way all the time, the moment these things start to get out. This will just become of a piece with our growing cybersecurity concerns. Malicious code is something we have now; it already cost us billions and billions of dollars a year to safeguard against it.
Eliezer: It doesn’t scale. There’s no continuity between what you have to do to fend off little pieces of code trying to break into your computer, and what you have to do to fend off something smarter than you. These are totally different realms and regimes and separate magisteria—a term we all hate, but nonetheless in this case, yes, separate magisteria of how you would even start to think about the problem. We’re not going to get automatic defense against superintelligence by building better and better anti-virus software.
Sam: Let’s just step back for a second. So we’ve talked about the AI-in-a-box scenario as being surprisingly unstable for reasons that we can perhaps only dimly conceive, but isn’t there even a scarier concern that this is just not going to be boxed anyway? That people will be so tempted to make money with their newest and greatest AlphaZeroZeroZeroNasdaq—what are the prospects that we will even be smart enough to keep the best of the best versions of almost-general intelligence in a box?
Eliezer: I mean, I know some of the people who say they want to do this thing, and all of the ones who are not utter idiots are past the point where they would deliberately enact Hollywood movie plots. Although I am somewhat concerned about the degree to which there’s a sentiment that you need to be able to connect to the Internet so you can run your AI on Amazon Web Services using the latest operating system updates, and trying to not do that is such a supreme disadvantage in this environment that you might as well be out of the game. I don’t think that’s true, but I’m worried about the sentiment behind it.
But the problem as I see it is… Okay, there’s a big big problem and a little big problem. The big big problem is, “Nobody knows how to make the nice AI.” You ask people how to do it, they either don’t give you any answers or they give you answers that I can shoot down in 30 seconds as a result of having worked in this field for longer than five minutes.
It doesn’t matter how good their intentions are. It doesn’t matter if they don’t want to enact a Hollywood movie plot. They don’t know how to do it. Nobody knows how to do it. There’s no point in even talking about the arms race if the arms race is between a set of unfriendly AIs with no friendly AI in the mix.
The little big problem is the arms race aspect, where maybe DeepMind wants to build a nice AI, maybe China is being responsible because they understand the concept of stability, but Russia copies China’s code and Russia takes off the safeties. That’s the little big problem, which is still a very large problem.
Sam: Yeah. I mean, most people think the real problem is human: malicious use of powerful AI that is safe. “Don’t give your AI to the next Hitler and you’re going to be fine.”
Eliezer: They’re just wrong. They’re just wrong as to where the problem lies. They’re looking in the wrong direction and ignoring the thing that’s actually going to kill them.
6. Accidental AI, mindcrime, and MIRI (1:34:30)
Sam: To be even more pessimistic for a second, I remember at that initial conference in Puerto Rico, there was this researcher—who I have not paid attention to since, but he seemed to be in the mix—I think his name was Alexander Wissner-Gross—and he seemed to be arguing in his presentation at that meeting that this would very likely emerge organically, already in the wild, very likely in financial markets. We would be put so many AI resources into the narrow paperclip-maximizing task of making money in the stock market that, by virtue of some quasi-Darwinian effect here, this will just knit together on its own online and the first general intelligence we’ll discover will be something that will be already out in the wild. Obviously, that does not seem ideal, but does that seem like a plausible path to developing something general and smarter than ourselves, or does that just seem like a fairy tale?
Eliezer: More toward the fairy tale. It seems to me to be only slightly more reasonable than the old theory that if you got dirty shirts and straw, they would spontaneously generate mice. People didn’t understand mice, so as far as they know, they’re a kind of thing that dirty shirts and straw can generate; but they’re not. And I similarly think that you would need a very vague model of intelligence, a model with no gears and wheels inside it, to believe that the equivalent of dirty shirts and straw generates it first, as opposed to people who have gotten some idea of what the gears and wheels are and are deliberately building the gears and wheels.
The reason why it’s slightly more reasonable than the dirty shirts and straw example is that maybe it is indeed true that if you just have people pushing on narrow AI for another 10 years past the point where AGI would otherwise become possible, they eventually just sort of wander into AGI. But I think that that happens 10 years later in the natural timeline than AGI put together by somebody who actually is trying to put together AGI and has the best theory out of the field of the contenders, or possibly just the most vast quantities of brute force, à la Google’s tensor chips. I think that it gets done on purpose 10 years before it would otherwise happen by accident.
Sam: Okay, so there’s I guess just one other topic here that I wanted to touch on before we close on discussing your book, which is not narrowly focused on this: this idea that consciousness will emerge at some point in our developing intelligent machines. Then we have the additional ethical concern that we could be building machines that can suffer, or building machines that can simulate suffering beings in such a way as to actually make suffering being suffer in these simulations. We could be essentially creating hells and populating them.
There’s no barrier to thinking about this being not only possible, but likely to happen, because again, we’re just talking about the claim that consciousness arises as an emergent property of some information-processing system and that this would be substrate-independent. Unless you’re going to claim (1) that consciousness does not arise on the basis of anything that atoms do—it has some other source—or (2) those atoms have to be the wet atoms in biological substrate and they can’t be in silico. Neither of those claims is very plausible at this point scientifically.
So then you have to imagine that as long as we just keep going, keep making progress, we will eventually build, whether by design or not, systems that not only are intelligent but are conscious. And then this opens a category of malfeasance that you or someone in this field has dubbed mindcrime. What is mindcrime? And why is it so difficult to worry about?
Eliezer: I think, by the way, that that’s a pretty terrible term. (laughs) I’m pretty sure I wasn’t the one who invented it. I am the person who invented some of these terrible terms, but not that one in particular.
First, I would say that my general hope here would be that as the result of building an AI whose design and cognition flows in a sufficiently narrow channel that you can understand it and make strong statements about it, you are also able to look at that and say, “It seems to me pretty unlikely that this is conscious or that if it is conscious, it is suffering.” I realize that this is a sort of high bar to approach.
The main way in which I would be worried about conscious systems emerging within the system without that happening on purpose would be if you have a smart general intelligence and it is trying to model humans. We know humans are conscious, so the computations that you run to build very accurate predictive models of humans are among the parts that are most likely to end up being conscious without somebody having done that on purpose.
Sam: Did you see the Black Mirror episode that basically modeled this?
Eliezer: I haven’t been watching Black Mirror, sorry. (laughs)
Sam: You haven’t been?
Eliezer: I haven’t been, nope.
Sam: They’re surprisingly uneven. Some are great, and some are really not great, but there’s one episode where—and this is spoiler alert, if you’re watching Black Mirror and you don’t want to hear any punch lines then tune out here—but there’s one episode which is based on this notion that basically you just see these people living in this dystopian world of total coercion where they’re just assigned through this lottery dates that go well or badly. You see the dating life of these people going on and on, where they’re being forced by some algorithm to get together or break up.
Eliezer: And let me guess, this is the future’s OkCupid trying to determine good matches?
Sam: Exactly, yes.
Eliezer: (laughs)
Sam: They’re just simulated minds in a dating app that’s being optimized for real people who are outside holding the phone, but yeah. The thing you get is that all of these conscious experiences have been endlessly imposed on these people in some hellscape of our devising.
Eliezer: That’s actually a surprisingly good plot, in that it doesn’t just assume that the programmers are being completely chaotic and stupid and randomly doing the premise of the plot. Like, there’s actually a reason why the AI is simulating all these people, so good for them, I guess.
And I guess that does get into the thing I was going to say, which is that I’m worried about minds being embedded because they are being used predictively, to predict humans. That is the obvious reason why that would happen without somebody intending it. Whereas endless dystopias don’t seem to me to have any use to a paperclip maximizer.
Sam: Right. All right, so there’s undoubtedly much more to talk about here. I think we’re getting up on the two-hour mark here, and I want to touch on your new book, which as I said I’m halfway through and finding very interesting.
Eliezer: If I can take a moment for a parenthetical before then, sorry?
Sam: Sure, go for it.
Eliezer: I just wanted to say that thanks mostly to the cryptocurrency boom—go figure, a lot of early investors in cryptocurrency were among our donors—the Machine Intelligence Research Institute is no longer strapped for cash, so much as it is strapped for engineering talent. (laughs)
Sam: Nice. That’s a good problem to have.
Eliezer: Yeah. If anyone listening to this is a brilliant computer scientist who wants to work on more interesting problems than they’re currently working on, and especially if you are already oriented to these issues, please consider going to intelligence.org/engineers if you’d like to work for our nonprofit.
Sam: Let’s say a little more about that. I will have given a bio for you in the introduction here, but the Machine Intelligence Research Institute (MIRI) is an organization that you co-founded, which you’re still associated with. Do you want to say what is happening there and what jobs are on offer?
Eliezer: Basically, it’s the original AI alignment organization that, especially today, works primarily on the technical parts of the problem and the technical issues. Previously, it has been working mainly on a more pure theory approach, but now that narrow AI has gotten powerful enough, people (not just us but elsewhere, like DeepMind) are starting to take shots at, “With current technology, what setups can we do that will tell us something about how to do this stuff?” So the technical side of AI alignment is getting a little bit more practical. I’m worried that it’s not happening fast enough, but, well, if you’re worried about that sort of thing, what one does is adds funding and especially adds smart engineers.
Sam: Do you guys collaborate with any of these companies doing the work? Do you have frequent contact with DeepMind or Facebook or anyone else?
Eliezer: I mean, the people in AI alignment all go to the same talks, and I’m sure that the people who do AI alignment at DeepMind talk to DeepMind. Sometimes we’ve been known to talk to the upper people at DeepMind, and DeepMind is in the same country as the Oxford Future of Humanity Institute. So bandwidth here might not be really optimal, but it’s certainly not zero.
7. Inadequate equilibria (1:44:40)
Sam: Okay, so your new book—again, the title is Inadequate Equilibria: Where and How Civilizations Get Stuck. That is a title that needs some explaining. What do you mean by “inadequate”? What do you mean by “equilibria”? And how does this relate to civilizations getting stuck?
Eliezer: So, one way to look at the book is that it’s about how you can get crazy, stupid, evil large systems without any of the people inside them being crazy, evil, or stupid.
I think that a lot of people look at various aspects of the dysfunction of modern civilization and they sort of hypothesize evil groups that are profiting from the dysfunction and sponsoring the dysfunction; and if only we defeated these evil people, the system could be rescued. And the truth is more complicated than that. But what are the details? The details matter a lot. How do you have systems full of nice people doing evil things?
Sam: Yeah. I often reference this problem by citing the power of incentives, but there are many other ideas here which are very useful to think about, which capture what we mean by the power of incentives.
There are a few concepts here that we should probably mention. What is a coordination problem? This is something you reference in the book.
Eliezer: A coordination problem is where there’s a better way to do it, but you have to change more than one thing at a time. So an example of a problem is: Let’s say you have Craigslist, which is one system where buyers and sellers meet to buy and sell used things within a local geographic area. Let’s say that you have an alternative to Craigslist and your alternatives is Danslist, and Danslist is genuinely better. (Let’s not worry for a second about how many startups think that without it being true; suppose it’s like genuinely better.)
All of the sellers on Craigslist want to go someplace that there’s buyers. All of the buyers on Craigslist want to go someplace that there’s sellers. How do you get your new system started when it can’t get started by one person going on to Danslist and two people going on to Danslist? There’s no motive for them to go there until there’s already a bunch of people on Danslist.
An awful lot of times, when you find a system that is stuck in an evil space, what’s going on with it is that for it to move out of that space, more than one thing inside it would have to change at a time. So there’s all these nice people inside it who would like to be in a better system, but everything they could locally do on their own initiative is not going to fix the system, and it’s going to make things worse for them.
That’s the kind of problem that scientists have with trying to get away from the journals that are just ripping them off. They’re starting to move away from those journals, but journals have prestige based on the scientists that publish there and the other scientists that cite them, and if you just start this one new journal all by yourself and move there all by yourself, it has a low impact factor. So everyone’s got to move simultaneously. That’s how the scam went on for 10 years. 10 years is a long time, but they couldn’t all jump to the new system because they couldn’t jump one at a time.
Sam: Right. The problem is that the world is organized in such a way that it is rational for each person to continue to behave the way he or she is behaving in this highly suboptimal way, given the way everyone else is behaving. And to change your behavior by yourself isn’t sufficient to change the system, and is therefore locally irrational, because your life will get worse if you change by yourself. Everyone has to coordinate their changing so as to move to some better equilibrium.
Eliezer: That’s one of the fundamental foundational ways that systems can get stuck. There are others.
Sam: The example that I often use when talking about problems of this sort is life in a maximum-security prison, which is as perversely bad as one can imagine. The incentives are aligned in such a way that no matter how good you are, if you’re put into a maximum-security prison, it is only rational for you to behave terribly and unethically and in such a way as to guarantee that this place is far more unpleasant than it need be, just because of how things are structured.
One example that I’ve used, and that people are familiar with at this point from having read books and seen movies that depict this more or less accurately: whether or not you’re a racist, your only rational choice, apparently, is to join a gang that is aligned along the variable of race. And if you fail to do this, you’ll be preyed upon by everyone. So if you’re a white guy, you have to join the white Aryan neo-Nazi gang. If you’re a black guy, you have to join the black gang. Otherwise, you’re just in the middle of this war of all against all. And there’s no way for you, based on your ethical commitment to being non-racist, to change how this is functioning.
And we’re living in a similar kind of prison, of sorts, when you just look at how non-optimal many of these attractor states are that we are stuck in civilizationally.
Eliezer: Parenthetically, I do want to be slightly careful about using the word “rational” to describe the behavior of people stuck in the system, because I consider that to be a very powerful word. It’s possible that if they were all really rational and had common knowledge of rationality, they would be able to solve the coordination problem. But humanly speaking—not so much in terms of ideal rationality, but in terms of what people can actually do and the options they actually have—their best choice is still pretty bad systemically.
Sam: Yeah. So what do you do in this book? How would you summarize your thesis? How do we move forward? Is there anything to do, apart from publicizing the structure of this problem?
Eliezer: It’s not really a very hopeful book in that regard. It’s more about how to predict which parts of society will perform poorly to the point where you as an individual can manage to do better for yourself, really. One of the examples I give in the book is that my wife has Seasonal Affective Disorder, and she cannot be treated by the tiny little light boxes that your doctor tries to prescribe. So I’m like, “Okay, if the sun works, there’s some amount of light that works, how about if I just try stringing up the equivalent of 100 light bulbs in our apartment?”
Now, when you have an idea like this, somebody might ask, “Well, okay, but you’re not thinking in isolation. There’s a civilization around you. If this works, shouldn’t there be a record of it? Shouldn’t a researcher have investigated it already?” There’s literally probably more than 100 million people around the world, especially in the extreme latitudes, who have some degree of Seasonal Affective Disorder, and some of it’s pretty bad. That means that there’s a kind of profit, a kind of energy gradient that seems like it could be traversable if solving the problem was as easy as putting up a ton of light bulbs in your apartment. Wouldn’t some enterprising researcher have investigated this already? Wouldn’t the results be known?
And the answer is, as far as I can tell, no. It hasn’t been investigated, the results aren’t known, and when I tried putting up a ton of light bulbs, it seems to have worked pretty well for my wife. Not perfectly, but a lot better than it used to be.
So why isn’t this one of the first things you find when you Google “What do I do about Seasonal Affective Disorder when the light box doesn’t work?” And that’s what takes this sort of long story, that’s what takes the analysis. That’s what takes the thinking about the journal system and what the funding sources are for people investigating Seasonal Affective Disorder, and what kind of publications get the most attention. And whether the barrier of needing to put up 100 light bulbs in a bunch of different apartments for people in the controlled study—which would be difficult to blind, except maybe by using a lot fewer light bulbs—whether the details of having to adapt light bulbs to every house which is different is enough of an obstacle to prevent any researcher from ever investigating this obvious-seeming solution to a problem that probably hundreds of millions of people have, and maybe 50 million people have very severely? As far as I can tell, the answer is yes.
And this is the kind of thinking that does not enable you to save civilization. If there was a way to make an enormous profit by knowing this, the profit would probably already be taken. If it was possible for one person to fix the problem, it would probably already be fixed. But you, personally, can fix your wife’s crippling Seasonal Affective Disorder by doing something that science knows not, because of an inefficiency in the funding sources for the researchers.
Sam: This is really the global problem we need to figure out how to tackle, which is to recognize those points on which incentives are perversely misaligned so as to guarantee needless suffering or complexity or failure to make breakthroughs that would raise our quality of life immensely. So identify those points and then realign the incentives somehow.
The market is in many respects good at this, but there are places where it obviously fails. We don’t have many tools to apply the right pressure here. You have the profit motive in markets—so you can either get fantastically rich by solving some problem, or not—or we have governments that can decide, “Well, this is a problem that markets can’t solve because the wealth isn’t there to be gotten, strangely, and yet there’s an immense amount of human suffering that would be alleviated if you solved this problem. You can’t get people for some reason to pay for the alleviation of that suffering, reliably.” But apart from markets and governments, are there any other large hammers to be wielded here?
Eliezer: I mean, sort of crowdfunding, I guess, although the hammer currently isn’t very large. But mostly, like I said, this book is about where you can do better individually or in small groups and when you shouldn’t assume that society knows what it’s doing; and it doesn’t have a bright message of hope about how to fix things.
I’m sort of prejudiced personally over here, because I think that the artificial general intelligence timeline is likely to run out before humanity gets that much better at solving inadequacy, systemic problems in general. I don’t really see human nature or even human practice changing by that much over the amount of time we probably have left.
Economists already know about market failures. That’s a concept they already have. They already have the concept of government trying to correct it. It’s not obvious to me that there is a quantum leap to be made staying within just those dimensions of thinking about the problem.
If you ask me, “Hey, Eliezer: it’s five years in the future, there’s still no artificial general intelligence, and a great leap forward has occurred in people to deal with these types of systemic issues. How did that happen?” Then my guess would be something like Kickstarter, but much better, that turned out to enable people in large groups to move forward when none of them could move forward individually. Something like the group movements that scientists made without all that much help from the government (although there was help from funders changing their policies) to jump to new journals all at the same time, and get partially away from the Elsevier closed-source journal scam. Maybe there’s something brilliant that Facebook does—with machine learning, even. They get better at showing people things that are solutions to their coordination problems; they’re better at routing those around when they exist, and people learn that these things work and they jump using them simultaneously. And by these means, voters start to elect politicians who are not nincompoops, as opposed to choosing whichever nincompoop on offer is most appealing.
But this is a fairy tale. This is not a prediction. This is, “If you told me that somehow this had gotten significantly better in five years, what happened?” This is me making up what might have happened.
8. Rapid capability gain in AGI (1:59:02)
Sam: Right. Well, I don’t see how that deals with the main AI concern we’ve been talking about. I can see some shift, or some solution to a massive coordination problem, politically or in just the level of widespread human behavior—let’s say our use of social media and our vulnerability to fake news and conspiracy theories and other crackpottery, let’s say we find some way to all shift our information diet and our expectations and solve a coordination problem that radically cleans up our global conversation. I can see that happening.
But when you’re talking about dealing with the alignment problem, you’re talking about changing the behavior of a tiny number of people comparatively. I mean, I don’t know what it is. What’s the community of AI researchers now? It’s got to be numbered really in the hundreds when you’re talking about working on AGI. But what will it be when we’re close to the finish line? How many minds would have to suddenly change and becoming immune to the wrong economic incentives to coordinate the solution there? What are we talking about, 10,000 people?
Eliezer: I mean, first of all, I don’t think we’re looking at an economic problem. I think that artificial general intelligence capabilities, once they exist, are going to scale too fast for that to be a useful way to look at the problem. AlphaZero going from 0 to 120 mph in four hours or a day—that is not out of the question here. And even if it’s a year, a year is still a very short amount of time for things to scale up. I think that the main thing you should be trying to do with the first artificial general intelligence ever built is a very narrow, non-ambitious task that shuts down the rest of the arms race by putting off switches in all the GPUs and shutting them down if anyone seems to be trying to build an overly artificially intelligent system.
Because I don’t think that the AI that you have built narrowly enough that you understood what it was doing is going to be able to defend you from arbitrary unrestrained superintelligences. The AI that you have built understandably enough to be good and not done fully general recursive self-improvement is not strong enough to solve the whole problem. It’s not strong enough to have everyone else going off and developing their own artificial general intelligences after that without that automatically destroying the world.
Sam: We’ve been speaking for now over two hours; what can you say to someone who has followed us this long, but for whatever reason the argument we’ve made has not summed to being emotionally responsive to the noises you just made. Is there anything that can be briefly said so as to give them pause?
Eliezer: I’d say this is a thesis of capability gain. This is a thesis of how fast artificial general intelligence gains in power once it starts to be around, whether we’re looking at 20 years (in which case this scenario does not happen) or whether we’re looking at something closer to the speed at which Go was developed (in which case it does happen) or the speed at which AlphaZero went from 0 to 120 and better-than-human (in which case there’s a bit of an issue that you better prepare for in advance, because you’re not going to have very long to prepare for it once it starts to happen).
And I would say this is a computer science issue. This is not here to be part of a narrative. This is not here to fit into some kind of grand moral lesson that I have for you about how civilization ought to work. I think that this is just the way the background variables are turning up.
Why do I think that? It’s not that simple. I mean, I think a lot of people who see the power of intelligence will already find that pretty intuitive, but if you don’t, then you should read my paper Intelligence Explosion Microeconomics about returns on cognitive reinvestment. It goes through things like the evolution of human intelligence and how the logic of evolutionary biology tells us that when human brains were increasing in size, there were increasing marginal returns to fitness relative to the previous generations for increasing brain size. Which means that it’s not the case that as you scale intelligence, it gets harder and harder to buy. It’s not the case that as you scale intelligence, you need exponentially larger brains to get linear improvements.
At least something slightly like the opposite of this is true; and we can tell this by looking at the fossil record and using some logic, but that’s not simple.
Sam: Comparing ourselves to chimpanzees works. We don’t have brains that are 40 times the size or 400 times the size of chimpanzees, and yet what we’re doing—I don’t know what measure you would use, but it exceeds what they’re doing by some ridiculous factor.
Eliezer: And I find that convincing, but other people may want additional details. And my message would be that the emergency situation is not part of a narrative. It’s not there to make the point of some kind of moral lesson. It’s my prediction as to what happens, after walking through a bunch of technical arguments as to how fast intelligence scales when you optimize it harder.
AlphaZero seems to me like a genuine case in point. That is showing us that capabilities that in humans require a lot of tweaking and that human civilization built up over centuries of masters teaching students how to play Go, and that no individual human could invent in isolation… Even the most talented Go player, if you plopped them down in front of a Go board and gave them only a day, would play garbage. If they had to invent all of their own Go strategies without being part of a civilization that played Go, they would not be able to defeat modern Go players at all. AlphaZero blew past all of that in less than a day, starting from scratch, without looking at any of the games that humans played, without looking at any of the theories that humans had about Go, without looking at any of the accumulated knowledge that we had, and without very much in the way of special-case code for Go rather than chess—in fact, zero special-case code for Go rather than chess. And that in turn is an example that refutes another thesis about how artificial general intelligence develops slowly and gradually, which is: “Well, it’s just one mind; it can’t beat our whole civilization.”
I would say that there’s a bunch of technical arguments which you walk through, and then after walking through these arguments you assign a bunch of probability, maybe not certainty, to artificial general intelligence that scales in power very fast—a year or less. And in this situation, if alignment is technically difficult, if it is easy to screw up, if it requires a bunch of additional effort—in this scenario, if we have an arms race between people who are trying to get their AGI first by doing a little bit less safety because from their perspective that only drops the probability a little; and then someone else is like, “Oh no, we have to keep up. We need to strip off the safety work too. Let’s strip off a bit more so we can get in the front.”—if you have this scenario, and by a miracle the first people to cross the finish line have actually not screwed up and they actually have a functioning powerful artificial general intelligence that is able to prevent the world from ending, you have to prevent the world from ending. You are in a terrible, terrible situation. You’ve got your one miracle. And this follows from the rapid capability gain thesis and at least the current landscape for how these things are developing.
Sam: Let’s just linger on this point for a second. This fast takeoff—is this assuming recursive self improvement? And how fringe an idea is this in the field? Are most people who are thinking about this assuming (for good reason or not) that a slow takeoff is far more likely, over the course of many, many years, and that the analogy to AlphaZero is not compelling?
Eliezer: I think they are too busy explaining why current artificial intelligence methods do not knowably, quickly, immediately give us artificial general intelligence—from which they then conclude that it is 30 years off. They have not said, “And then once we get there, it’s going to develop much more slowly than AlphaZero, and here’s why.” There isn’t a thesis to that effect that I’ve seen from artificial intelligence people. Robin Hanson had a thesis to this effect, and there was this mighty debate on our blog between Robin Hanson and myself that was published as the AI-Foom Debate mini-book. And I have claimed recently on Facebook that now that we’ve seen AlphaZero, AlphaZero seems like strong evidence against Hanson’s thesis for how these things necessarily go very slow because they have to duplicate all the work done by human civilization and that’s hard.
Sam: I’m actually going to be doing a podcast with Robin in a few weeks, a live event. So what’s the best version of his argument, and why is he wrong?
Eliezer: Nothing can prepare you for Robin Hanson! (laughs)
Well, the argument that Hanson has given is that these systems are still immature and narrow and things will change when they get general. And my reply has been something like, “Okay, what changes your mind short of the world actually ending? If you theory is wrong, do we get to find out about that at all before the world ends?”
Sam: To which he says?
Eliezer: I don’t remember if he’s replied to that one yet.
Sam: I’ll let Robin be Robin. Well, listen, Eliezer, it has been great to talk to you, and I’m glad we got a chance to do it at such length. And again, it does not exhaust the interest or consequence of this topic, but it’s certainly a good start for people who are new to this. Before I let you go, where should people look for you online? Do you have a preferred domain that we could target?
Eliezer: I would mostly say intelligence.org. If you’re looking for me personally, facebook.com/yudkowsky, and if you’re looking for my most recent book, equilibriabook.com.
Sam: I’ll put links on my website where I embed this podcast. So again, Eliezer, thanks so much—and to be continued. I always love talking to you, and this will not be the last time, AI willing.
Eliezer: This was a great conversation, and thank you very much for having me on.
Did you like this post? You may enjoy our other Conversations posts, including: