This post is a transcript of a discussion between Paul Christiano, Ajeya Cotra, and Eliezer Yudkowsky on AGI forecasting, following up on Paul and Eliezer’s “Takeoff Speeds” discussion.
Color key:
Chat by Paul and Eliezer | Chat by Ajeya | Inline comments |
8. September 20 conversation
8.1. Chess and Evergrande
I still feel like you are overestimating how big a jump alphago is, or something. Do you have a mental prediction of how the graph of (chess engine quality) vs (time) looks, and whether neural net value functions are a noticeable jump in that graph?
Like, people investing in “Better Software” doesn’t predict that you won’t be able to make progress at playing go. The reason you can make a lot of progress at go is that there was extremely little investment in playing better go.
So then your work is being done by the claim “People won’t be working on the problem of acquiring a decisive strategic advantage,” not that people won’t be looking in quite the right place and that someone just had a cleverer idea
I think I’d expect something like… chess engine slope jumps a bit for Deep Blue, then levels off with increasing excitement, then jumps for the Alpha series? Albeit it’s worth noting that Deepmind’s efforts there were going towards generality rather than raw power; chess was solved to the point of being uninteresting, so they tried to solve chess with simpler code that did more things. I don’t think I do have strong opinions about what the chess trend should look like, vs. the Go trend; I have no memories of people saying the chess trend was breaking upwards or that there was a surprise there.
Incidentally, the highly well-traded financial markets are currently experiencing sharp dips surrounding the Chinese firm of Evergrande, which I was reading about several weeks before this.
I don’t see the basic difference in the kind of reasoning that says “Surely foresightful firms must produce investments well in advance into earlier weaker applications of AGI that will double the economy”, and the reasoning that says “Surely world economic markets and particular Chinese stocks should experience smooth declines as news about Evergrande becomes better-known and foresightful financial firms start to remove that stock from their portfolio or short-sell it”, except that in the latter case there are many more actors with lower barriers to entry than presently exist in the auto industry or semiconductor industry never mind AI.
or if not smooth because of bandwagoning and rational fast actors, then at least the markets should (arguendo) be reacting earlier than they’re reacting now, given that I heard about Evergrande earlier; and they should have options-priced Covid earlier; and they should have reacted to the mortgage market earlier. If even markets there can exhibit seemingly late wild swings, how is the economic impact of AI – which isn’t even an asset market! – forced to be earlier and smoother than that, as a result of wise investing?
There’s just such a vast gap between hopeful reasoning about how various agents and actors should all do the things the speaker finds very reasonable, thereby yielding smooth behavior of the Earth, versus reality.
9. September 21 conversation
9.1. AlphaZero, innovation vs. industry, the Wright Flyer, and the Manhattan Project
(For benefit of readers, the market is down 1.5% from friday close -> tuesday open, after having drifted down 2.5% over the preceding two weeks. Draw whatever lesson you want from that.)
Also for the benefit of readers, here is the SSDF list of computer chess performance by year. I think the last datapoint is with the first version of neural net evaluations, though I think to see the real impact we want to add one more datapoint after the neural nets are refined (which is why I say I also don’t know what the impact is)

No one keeps similarly detailed records for Go, and there is much less development effort, but the rate of progress was about 1 stone per year from 1980 until 2015 (see https://intelligence.org/files/AlgorithmicProgress.pdf, written way before AGZ). In 2012 go bots reached about 4-5 amateur dan. By DeepMind’s reckoning here (https://www.nature.com/articles/nature16961, figure 4) Fan AlphaGo about 4-5 stones stronger-4 years later, with 1 stone explained by greater runtime compute. They could then get further progress to be superhuman with even more compute, radically more than were used for previous projects and with pretty predictable scaling. That level is within 1-2 stones of the best humans (professional dan are greatly compressed relative to amateur dan), so getting to “beats best human” is really just not a big discontinuity and the fact that DeepMind marketing can find an expert who makes a really bad forecast shouldn’t be having such a huge impact on your view.
This understates the size of the jump from AlphaGo, because that was basically just the first version of the system that was superhuman and it was still progressing very rapidly as it moved from prototype to slightly-better-prototype, which is why you saw such a close game. (Though note that the AlphaGo prototype involved much more engineering effort than any previous attempt to play go, so it’s not surprising that a “prototype” was the thing to win.)
So to look at actual progress after the dust settles and really measure how crazy this was, it seems much better to look at AlphaZero which continued to improve further, see (https://sci-hub.se/https://www.nature.com/articles/nature24270, figure 6b). Their best system got another ~8 stones of progress over AlphaGo. Now we are like 7-10 stones ahead of trend, of which I think about 3 stones are explained by compute. Maybe call it 6 years ahead of schedule?
So I do think this is pretty impressive, they were slightly ahead of schedule for beating the best humans but they did it with a huge margin of error. I think the margin is likely overstated a bit by their elo evaluation methodology, but I’d still grant like 5 years ahead of the nearest competition.
I’d be interested in input from anyone who knows more about the actual state of play (+ is allowed to talk about it) and could correct errors.
Mostly that whole thread is just clearing up my understanding of the empirical situation, probably we still have deep disagreements about what that says about the world, just as e.g. we read very different lessons from market movements.
Probably we should only be talking about either ML or about historical technologies with meaningful economic impacts. In my view your picture is just radically unlike how almost any technologies have been developed over the last few hundred years. So probably step 1 before having bets is to reconcile our views about historical technologies, and then maybe as a result of that we could actually have a bet about future technology. Or we could try to shore up the GDP bet.
Like, it feels to me like I’m saying: AI will be like early computers, or modern semiconductors, or airplanes, or rockets, or cars, or trains, or factories, or solar panels, or genome sequencing, or basically anything else. And you are saying: AI will be like nuclear weapons.
I think from your perspective it’s more like: AI will be like all the historical technologies, and that means there will be a hard takeoff. The only way you get a soft takeoff forecast is by choosing a really weird thing to extrapolate from historical technologies.
So we’re both just forecasting that AI will look kind of like other stuff in the near future, and then both taking what we see as the natural endpoint of that process.
To me it feels like the nuclear weapons case is the outer limit of what looks plausible, where someone is able to spend $100B for a chance at a decisive strategic advantage.
Go-wise, I’m a little concerned about that “stone” metric – what would the chess graph look like if it was measuring pawn handicaps? Are the professional dans compressed in Elo, not just “stone handicaps”, relative to the amateur dans? And I’m also hella surprised by the claim, which I haven’t yet looked at, that Alpha Zero got 8 stones of progress over AlphaGo – I would not have been shocked if you told me that God’s Algorithm couldn’t beat Lee Se-dol with a 9-stone handicap.
Like, the obvious metric is Elo, so if you go back and refigure in “stone handicaps”, an obvious concern is that somebody was able to look into the past and fiddle their hindsight until they found a hindsightful metric that made things look predictable again. My sense of Go said that 5-dan amateur to 9-dan pro was a HELL of a leap for 4 years, and I also have some doubt about the original 5-dan-amateur claims and whether those required relatively narrow terms of testing (eg timed matches or something).
One basic point seems to be whether AGI is more like an innovation or like a performance metric over an entire large industry.
Another point seems to be whether the behavior of the world is usually like that, in some sense, or if it’s just that people who like smooth graphs can go find some industries that have smooth graphs for particular performance metrics that happen to be smooth.
Among the smoothest metrics I know that seems like a convergent rather than handpicked thing to cite, is world GDP, which is the sum of more little things than almost anything else, and whose underlying process is full of multiple stages of converging-product-line bottlenecks that make it hard to jump the entire GDP significantly even when you jump one component of a production cycle… which, from my standpoint, is a major reason to expect AI to not hit world GDP all that hard until AGI passes the critical threshold of bypassing it entirely. Having 95% of the tech to invent a self-replicating organism (eg artificial bacterium) does not get you 95%, 50%, or even 10% of the impact.
(it’s not so much the 2% reaction of world markets to Evergrande that I was singling out earlier, 2% is noise-ish, but the wider swings in the vicinity of Evergrande particularly)
Yeah, I’m just using “stone” to mean “elo difference that is equal to 1 stone at amateur dan / low kyu,” you can see DeepMind’s conversion (which I also don’t totally believe) in figure 4 here (https://sci-hub.se/https://www.nature.com/articles/nature16961). Stones are closer to constant elo than constant handicap, it’s just a convention to name them that way.
k then
But my description above still kind of understates the gap I think. They call 230 elo 1 stone, and I think prior rate of progress is more like 200 elo/year. They put AlphaZero about 3200 elo above the 2012 system, so that’s like 16 years ahead = 11 years ahead of schedule. At least 2 years are from test-time hardware, and self-play systematically overestimates elo differences at the upper end of that. But 5 years ahead is still too low and that sounds more like 7-9 years ahead. ETA: and my actual best guess all things considered is probably 10 years ahead, which I agree is just a lot bigger than 5. And I also understated how much of the gap was getting up to Lee Sedol.
The go graph I posted wasn’t made with hindsight, that was from 2014
I mean, I’m fine with you saying that people who like smooth graphs are cherry-picking evidence, but do you want to give any example other than nuclear weapons of technologies with the kind of discontinuous impact you are describing?
I do agree that the difference in our views is like “innovation” vs “industry.” And a big part of my position is that innovation-like things just don’t usually have big impacts for kind of obvious reasons, they start small and then become more industry-like as they scale up. And current deep learning seems like an absolutely stereotypical industry that is scaling up rapidly in an increasingly predictable way.
As far as I can tell the examples we know of things changing continuously aren’t handpicked, we’ve been looking at all the examples we can find, and no one is proposing or even able to find almost anything that looks like you are imagining AI will look.
Like, we’ve seen deep learning innovations in the form of prototypes (most of all AlexNet), and they were cool and represented giant fast changes in people’s views. And more recently we are seeing bigger much-less-surprising changes that are still helping a lot in raising the tens of billions of dollars that people are raising. And the innovations we are seeing are increasingly things that trade off against modest improvements in model size, there are fewer and fewer big surprises, just like you’d predict. It’s clearer and clearer to more and more people what the roadmap is—the roadmap is not yet quite as clear as in semiconductors, but as far as I can tell that’s just because the field is still smaller.
I sure wasn’t imagining there was a roadmap to AGI! Do you perchance have one which says that AGI is 30 years out?
From my perspective, you could as easily point to the Wright Flyer as an atomic bomb. Perhaps this reflects again the “innovation vs industry” difference, where I think in terms of building a thing that goes foom thereby bypassing our small cute world GDP, and you think in terms of industries that affect world GDP in an invariant way throughout their lifetimes.
Would you perhaps care to write off the atomic bomb too? It arguably didn’t change the outcome of World War II or do much that conventional weapons in great quantity couldn’t; Japan was bluffed into believing the US could drop a nuclear bomb every week, rather than the US actually having that many nuclear bombs or them actually being used to deliver a historically outsized impact on Japan. From the industry-centric perspective, there is surely some graph you can draw which makes nuclear weapons also look like business as usual, especially if you go by destruction per unit of whole-industry non-marginal expense, rather than destruction per bomb.
seems like you have to make the wright flyer much better before it’s important, and that it becomes more like an industry as that happens, and that this is intimately related to why so few people were working on it
I think the atomic bomb is further on the spectrum than almost anything, but it still doesn’t feel nearly as far as what you are expecting out of AI
the manhattan project took years and tens of billions; if you wait an additional few years and spend an additional few tens of billions then it would be a significant improvement in destruction or deterrence per $ (but not totally insane)
I do think it’s extremely non-coincidental that the atomic bomb was developed in a country that was practically outspending the whole rest of the world in “killing people technology”
and took a large fraction of that country’s killing-people resources
eh, that’s a bit unfair, the us was only like 35% of global spending on munitions
and the manhattan project itself was only a couple percent of total munitions spending
a lot of why I expect AGI to be a disaster is that I am straight-up expecting AGI to be different. if it was just like coal or just like nuclear weapons or just like viral biology then I would not be way more worried about AGI than I am worried about those other things.
that definitely sounds right
but it doesn’t seem like you have any short-term predictions about AI being different
9.2. AI alignment vs. biosafety, and measuring progress
are you more worried about AI than about bioengineering?
I’m more worried about AI because (i) alignment is a thing, unrelated to takeoff speed, (ii) AI is a (ETA: likely to be) huge deal and bioengineering is probably a relatively small deal
(in the sense of e.g. how much $ people spend, or how much $ it makes, or whatever other metric of size you want to use)
what’s the disanalogy to (i) biosafety is a thing, unrelated to the speed of bioengineering? why expect AI to be a huge deal and bioengineering to be a small deal? is it just that investing in AI is scaling faster than investment in bioengineering?
no, alignment is a really easy x-risk story, bioengineering x-risk seems extraordinarily hard
It’s really easy to mess with the future by creating new competitors with different goals, if you want to mess with the future by totally wiping out life you have to really try at it and there’s a million ways it can fail. The bioengineering seems like it basically requires deliberate and reasonably competent malice whereas alignment seems like it can only be averted with deliberate effort, etc.
I’m mostly asking about historical technologies to try to clarify expectations, I’m pretty happy if the outcome is: you think AGI is predictably different from previous technologies in ways we haven’t seen yet
though I really wish that would translate into some before-end-of-days prediction about a way that AGI will eventually look different
in my ontology a whole lot of threat would trace back to “AI hits harder, faster, gets too strong to be adjusted”; tricks with proteins just don’t have the raw power of intelligence
in my view it’s nearly totally orthogonal to takeoff speed, though fast takeoffs are a big reason that preparation in advance is more useful
(but not related to the basic reason that alignment is unprecedentedly scary)
It feels to me like you are saying that the AI-improving-AI will move very quickly from “way slower than humans” to “FOOM in <1 year,” but it just looks like that is very surprising to me.
However I do agree that if AI-improving-AI was like AlphaZero, then it would happen extremely fast.
It seems to me like it’s pretty rare to have these big jumps, and it gets much much rarer as technologies become more important and are more industry-like rather than innovation like (and people care about them a lot rather than random individuals working on them, etc.). And I can’t tell whether you are saying something more like “nah big jumps happen all the time in places that are structurally analogous to the key takeoff jump, even if the effects are blunted by slow adoption and regulatory bottlenecks and so on” or if you are saying “AGI is atypical in how jumpy it will be”
I don’t know about slower; GPT-3 may be able to type faster than a human
Yeah, I guess we’ve discussed how you don’t like the abstraction of “speed of making progress”
but, basically less useful in fundamental ways than a human civilization, because they are less complete, less self-contained
Even if we just assume that your AI needs to go off in the corner and not interact with humans, there’s still a question of why the self-contained AI civilization is making ~0 progress and then all of a sudden very rapid progress
unfortunately a lot of what you are saying, from my perspective, has the flavor of, “but can’t you tell me about your predictions earlier on of the impact on global warming at the Homo erectus level”
you have stories about why this is like totally not a fair comparison
I do not share these stories
I don’t understand either your objection nor the reductio
like, here’s how I think it works: AI systems improve gradually, including on metrics like “How long does it take them to do task X?” or “How high-quality is their output on task X?”
I feel like the thing we know is something like, there is a sufficiently high level where things go whooosh humans-from-hominids style
We can measure the performance of AI on tasks like “Make further AI progress, without human input”
Any way I can slice the analogy, it looks like AI will get continuously better at that task
how would you measure progress from GPT-2 to GPT-3, and would you feel those metrics really captured the sort of qualitative change that lots of people said they felt?
And it seems like we have a bunch of sources of data we can use about how fast AI will get better
Could we talk about some application of GPT-2 or GPT-3?
also that’s a lot of progress, spending 100x more is a lot more money
my world, GPT-3 has very few applications because it is not quite right and not quite complete
also it’s still really dumb
like a self-driving car that does great at 99% of the road situations
economically almost worthless
I think the “being dumb” is way more important than “covers every case”
(albeit that if new cities could still be built, we could totally take those 99%-complete AI cars and build fences and fence-gates around them, in a city where they were the only cars on the road, in which case they would work, and get big economic gains from these new cities with driverless cars, which ties back into my point about how current world GDP is unwilling to accept tech inputs)
like, it is in fact very plausible to me that there is a neighboring branch of reality with open borders and no housing-supply-constriction laws and no medical-supply-constriction laws, and their world GDP does manage to double before AGI hits them really hard, albeit maybe not in 4 years. this world is not Earth. they are constructing new cities to take advantage of 99%-complete driverless cars right now, or rather, they started constructing them 5 years ago and finished 4 years and 6 months ago.
9.3. Requirements for FOOM
I really feel like the important part is the jumpiness you are imagining on the AI side / why AGI is different from other things
It’s actually not obvious to me that Eliezer is imagining that much more jumpiness on the AI technology side than you are, Paul
E.g. he’s said in the past that while the gap from “subhuman to superhuman AI” could be 2h if it’s in the middle of FOOM, it could also be a couple years if it’s more like scaling alphago
Indeed! We observed this jumpiness with hominids. A lot of stuff happened at once with hominids, but a critical terminal part of the jump was the way that hominids started scaling their own food supply, instead of being ultimately limited by the food supply of the savanna.
A couple years is basically what Paul believes
(discord is not a great place for threaded conversations :()
What are the probabilities you’re each placing on the 2h-2y spectrum? I feel like Paul is like “no way on 2h, likely on 2y” and Eliezer is like “who knows” on the whole spectrum, and a lot of the disagreement is the impact of the previous systems?
yeah, I’m basically at “no way,” because it seems obvious that the AI that can foom in 2h is preceded by the AI that can foom in 2y
well, we surely agree there!
OK, and it seems to me like it is preceded by years
we disagree on whether the AI that can foom in 2y clearly comes more than 2y before the AI that fooms in 2h
yeah
perhaps we can all agree it’s preceded by at least 2h
so I have some view like: for any given AI we can measure “how long does it take to foom?” and it seems to me like this is just a nice graph
and it’s not exactly clear how quickly that number is going down, but a natural guess to me is something like “halving each year” based on the current rate of progress in hardware and software
and you see localized fast progress most often in places where there hasn’t yet been much attention
and my best guess for your view is that actually that’s not a nice graph at all, there is some critical threshold or range where AI quickly moves from “not fooming for a really long time” to “fooming really fast,” and that seems like the part I’m objecting to
Paul, is your take that there’s a non-infinity number for time to FOOM that’d be associated with current AI systems (unassisted by humans)?
And it’s going down over time?
I feel like I would have said something more like “there’s a $ amount it takes to build a system that will FOOM in X amount of time, and that’s going down”
where it’s like quadrillions of dollars today
I think it would be a big engineering project to make such an AI, which no one is doing because it would be uselessly slow even if successful
I… don’t think GPT-3 fooms given 2^30 longer time to think about than the systems that would otherwise exist 30 years from now, on timelines I’d consider relatively long, and hence generous to this viewpoint? I also don’t think you can take a quadrillion dollars and scale GPT-3 to foom today?
I would agree with your take on GPT-3 fooming, and I didn’t mean a quadrillion dollars just to scale GPT-3, would probably be a difft architecture
I also agree that GPT-3 doesn’t foom, it just keeps outputting <EOT>[next web page]<EOT>…
But I think the axes of “smart enough to foom fast” and “wants to foom” are pretty different. I also agree there is some minimal threshold below which it doesn’t even make sense to talk about “wants to foom,” which I think is probably just not that hard to reach.
(Also there are always diminishing returns as you continue increasing compute, which become very relevant if you try to GPT-3 for a billion billion years as in your hypothetical even apart from “wants to foom”.)
I think maybe you and EY then disagree on where the threshold from “infinity” to “a finite number” for “time for this AI system to FOOM” begins? where eliezer thinks it’ll drop from infinity to a pretty small finite number and you think it’ll drop to a pretty large finite number, and keep going down from there
I also think we will likely jump down to a foom-ing system only after stuff is pretty crazy, but I think that’s probably less important
I think what you said is probably the main important disagreement
as in before that point it’ll be faster to have human-driven progress than FOOM-driven progress bc the FOOM would be too slow?
and there’s some crossover point around when the FOOM time is just a bit faster than the human-driven progress time
yeah, I think most likely (AI+humans) is faster than (AI alone) because of complementarity. But I think Eliezer and I would still disagree even if I thought there was 0 complementarity and it’s just (humans improving AI) and separately (AI improving AI)
on that pure substitutes model I expect “AI foom” to start when the rate of AI-driven AI progress overtakes the previous rate of human-driven AI progress
like, I expect the time for successive “doublings” of AI output to be like 1 year, 1 year, 1 year, 1 year, [AI takes over] 6 months, 3 months, …
and the most extreme fast takeoff scenario that seems plausible is that kind of perfect substitutes + no physical economic impact from the prior AI systems
and then by that point fast enough physical impact is really hard so it happens essentially after the software-only singularity
I consider that view kind of unlikely but at least coherent
9.4. AI-driven accelerating economic growth
I’m expecting that the economy doesn’t accept much inputs from chimps, and then the economy doesn’t accept much input from village idiots, and then the economy doesn’t accept much input from weird immigrants. I can imagine that there may or may not be a very weird 2-year or 3-month period with strange half-genius systems running around, but they will still not be allowed to build houses. In the terminal phase things get more predictable and the AGI starts its own economy instead.
I guess you can go even faster, by having a big and accelerating ramp-up in human investment right around the end, so that the “1 year” is faster (e.g. if recursive self-improvement was like playing go, and you could move from “a few individuals” to “google spending $10B” over a few years)
My model prophecy doesn’t rule that out as a thing that could happen, but sure doesn’t emphasize it as a key step that needs to happen.
I think it’s very likely that AI will mostly be applied to further hardware+software progress
[Cotra: ➕] |
I don’t really understand why you keep talking about houses and healthcare
Eliezer, what about stuff like Google already using ML systems to automate its TPU load-sharing decisions, and people starting ot use Codex to automate routine programming, and so on? Seems like there’s a lot of stuff like that starting to already happen and markets are pricing in huge further increases
it seems like the non-AI up-for-grabs zone are things like manufacturing, not things like healthcare
[Cotra: ➕] |
(I mean on your timelines obviously not much time for acceleration anyway, but that’s distinct from the regulation not allowing weak AIs to do stuff story)
Because I think that a key thing of what makes your prophecy less likely is the way that it happens inside the real world, where, economic gains or not, the System is unwilling/unable to take the things that are 99% self-driving cars and start to derive big economic benefits from those.
but it seems like huge economic gains could happen entirely in industries mostly not regulated and not customer-facing, like hardware/software R&D, manufacturing. shipping logistics, etc
Ajeya, I’d consider Codex of far greater could-be-economically-important-ness than automated TPU load-sharing decisions
i would agree with that, it’s smarter and more general
and i think that kind of thing could be applied on the hardware chip design side too
no, because the TPU load-sharing stuff has an obvious saturation point as a world economic input, while superCodex could be a world economic input in many more places
the TPU load sharing thing was not a claim that this application could scale up to crazy impacts, but that it was allowed to happen, and future stuff that improves that kind of thing (back-end hardware/software/logistics) would probably also be allowed
my sense is that dectupling the number of programmers would not lift world GDP much, but it seems a lot more possible for me to be wrong about that
the point is that housing and healthcare are not central examples of things that scale up at the beginning of explosive growth, regardless of whether it’s hard or soft
they are slower and harder, and also in efficient markets-land they become way less important during the transition
so they aren’t happening that much on anyone’s story
and also it doesn’t make that much difference whether they happen, because they have pretty limited effects on other stuff
like, right now we have an industry of ~hundreds of billions that is producing computing hardware, building datacenters, mining raw inputs, building factories to build computing hardware, solar panels, shipping around all of those parts, etc. etc.
I’m kind of interested in the question of whether all that stuff explodes, although it doesn’t feel as core as the question of “what are the dynamics of the software-only singularity and how much $ are people spending initiating it?”
but I’m not really interested in the question of whether human welfare is spiking during the transition or only after
All of world GDP has never felt particularly relevant to me on that score, since twice as much hardware maybe corresponds to being 3 months earlier, or something like that.
that sounds like the stuff of predictions?
But if complete chip manufacturing cycles have accepted much more effective AI input, with no non-AI bottlenecks, then that… sure is a much more material element of a foom cycle than I usually envision.
like, do you think it’s often the case that 3 months of software progress = doubling compute spending? or do you think AGI is different from “normal” AI on this perspective?
I don’t think that’s that far off anyway
I would guess like ~1 year
Like, world GDP that goes up by only 10%, but that’s because producing compute capacity was 2.5% of world GDP and that quadrupled, starts to feel much more to me like it’s part of a foom story.
I expect software-beats-hardware to hit harder and harder as you get closer to AGI, yeah.
the prediction is firmer near the terminal phase, but I think this is also a case where I expect that to be visible earlier
I think that by the time that the AI-improving-AI takes over, it’s likely that hardware+software manufacturing+R&D represents like 10-20% of GDP, and that the “alien accountants” visiting earth would value those companies at like 80%+ of GDP
9.5. Brain size and evolutionary history
On software beating hardware, how much of your view is dependent on your belief that the chimp -> human transition was probably not mainly about brain size because if it were about brain size it would have happened faster? My understanding is that you think the main change is a small software innovation which increased returns to having a bigger brain. If you changed your mind and thought that the chimp -> human transition was probably mostly about raw brain size, what (if anything) about your AI takeoff views would change?
I think that’s a pretty different world in a lot of ways!
but yes it hits AI takeoff views too
regarding software vs hardware, here is an example of asking this question for imagenet classification (“how much compute to train a model to do the task?”), with a bit over 1 year doubling times (https://openai.com/blog/ai-and-efficiency/). I guess my view is that we can make a similar graph for “compute required to make your AI FOOM” and that it will be falling significantly slower than 2x/year. And my prediction for other tasks is that the analogous graphs will also tend to be falling slower than 2x/year.
to the extent that I modeled hominid evolution as having been “dutifully schlep more of the same stuff, get predictably more of the same returns” that would correspond to a world in which intelligence was less scary, different, dangerous-by-default
thanks, that’s helpful. I looked around in IEM and other places for a calculation of how quickly we should have evolved to humans if it were mainly about brain size, but I only found qualitative statements. If there’s a calculation somewhere I would appreciate a pointer to it, because currently it seems to me that a story like “selection pressure toward general intelligence was weak-to-moderate because it wasn’t actually that important for fitness, and this degree of selection pressure is consistent with brain size being the main deal and just taking a few million years to happen” is very plausible
well, for one thing, the prefrontal cortex expanded twice as fast as the rest
and iirc there’s evidence of a lot of recent genetic adaptation… though I’m not as sure you could pinpoint it as being about brain-stuff or that the brain-stuff was about cognition rather than rapidly shifting motivations or something.
elephant brains are 3-4 times larger by weight than human brains (just looked up)
if it’s that easy to get returns on scaling, seems like it shouldn’t have taken that long for evolution to go there
but they have fewer synapses (would compute to less FLOP/s by the standard conversion)
how long do you think it should have taken?
early dinosaurs should’ve hopped onto the predictable returns train
is there a calculation?
you said in IEM that evolution increases organ sizes quickly but there wasn’t a citation to easily follow up on there
I mean, you could produce a graph of smooth fitness returns to intelligence, smooth cognitive returns on brain size/activity, linear metabolic costs for brain activity, fit that to humans and hominids, then show that obviously if hominids went down that pathway, large dinosaurs should’ve gone down it first because they had larger bodies and the relative metabolic costs of increased intelligence would’ve been lower at every point along the way
I do not have a citation for that ready, if I’d known at the time you’d want one I’d have asked Luke M for it while he still worked at MIRI 😐
cool thanks, will think about the dinosaur thing (my first reaction is that this should depend on the actual fitness benefits to general intelligence which might have been modest)
I suspect we’re getting off Paul’s crux, though
yeah we can go back to that convo (though i think paul would also disagree about this thing, and believes that the chimp to human thing was mostly about size)
sorry for hijacking
well, if at some point I can produce a major shift in EA viewpoints by coming up with evidence for a bunch of non-brain-size brain selection going on over those timescales, like brain-related genes where we can figure out how old the mutation is, I’d then put a lot more priority on digging up a paper like that
I’d consider it sufficiently odd to imagine hominids->humans as being primarily about brain size, given the evidence we have, that I do not believe this is Paul’s position until Paul tells me so
I would guess it’s primarily about brain size / neuron count / cortical neuron count
and that the change in rate does mostly go through changing niche, where both primates and birds have this cycle of rapidly accelerating brain size increases that aren’t really observed in other animals
it seems like brain size is increasing extremely quickly on both of those lines
why aren’t elephants GI?
mostly they have big brains to operate big bodies, and also my position obviously does not imply (big brain) ==(necessarily implies)==> general intelligence
I don’t understand, in general, how your general position manages to strongly imply a bunch of stuff about AGI and not strongly imply similar stuff about a bunch of other stuff that sure sounds similar to me
don’t elephants have very few synapses relative to humans?
[Cotra: ➕] |
how does the scale hypothesis possibly take a strong stand on synapses vs neurons? I agree that it takes a modest predictive hit from “why aren’t the big animals much smarter?”
if adding more synapses just scales, elephants should be able to pay hominid brain costs for a much smaller added fraction of metabolism and also not pay the huge death-in-childbirth head-size tax
because their brains and heads are already 4x as huge as they need to be for GI
and now they just need some synapses, which are a much tinier fraction of their total metabolic costs
I mean, you can also make smaller and cheaper synapses as evidenced by birds
I’m not sure I understand what you are saying
it’s clear that you can’t say “X is possible metabolically, so evolution would do it”
or else you are confused about why primate brains are so bad
great, then smaller and cheaper synapses should’ve scaled many eons earlier and taken over the world
this isn’t about general intelligence, this is a reductio of your position…
and here I had thought it was a reductio of your position…
indeed
like, we all grant that it’s metabolically possible to have small smart brains
and evolution doesn’t do it
and I’m saying that it’s also possible to have small smart brains
and that scaling brains up matters a lot
no, you grant that it’s metabolically possible to have cheap brains full of synapses, which are therefore, on your position, smart
birds are just smart
we know they are smart
this isn’t some kind of weird conjecture
like, we can debate whether they are a “general” intelligence, but it makes no difference to this discussion
the point is that they do more with less metabolic cost
on my position, the brain needs to invent the equivalents of ReLUs and Transformers and really rather a lot of other stuff because it can’t afford nearly that many GPUs, and then the marginal returns on adding expensive huge brains and synapses have increased enough that hominids start to slide down the resulting fitness slope, which isn’t even paying off in guns and rockets yet, they’re just getting that much intelligence out of it once the brain software has been selected to scale that well
but all of the primates and birds have brain sizes scaling much faster than the other animals
like, the relevant “things started to scale” threshold is way before chimps vs humans
isn’t it?
to clarify, my understanding is that paul’s position is “Intelligence is mainly about synapse/neuron count, and evolution doesn’t care that much about intelligence; it cared more for birds and primates, and both lines are getting smarter+bigger-brained.” And eliezer’s position is that “evolution should care a ton about intelligence in most niches, so if it were mostly about brain size then it should have gone up to human brain sizes with the dinosaurs”
or like, what is the evidence you think is explained by the threshold being between chimps and humans
if hominids have less efficient brains than birds, on this theory, it’s because (post facto handwave) birds are tiny, so whatever cognitive fitness gradients they face, will tend to get paid more in software and biological efficiency and biologically efficient software, and less paid in Stack More Neurons (even compared to hominids)
elephants just don’t have the base software to benefit much from scaling synapses even though they’d be relatively cheaper for elephants
@ajeya I think that intelligence is about a lot of things, but that size (or maybe “more of the same” changes that had been happening recently amongst primates) is the big difference between chimps and humans
[Cotra: 👍] |
got it yeah i was focusing on chimp-human gap when i said “intelligence” there but good to be careful
I have not actually succeeded in understanding Why On Earth Anybody Would Think That If Not For This Really Weird Prior I Don’t Get Either
re: the “more of the same” theory of humans
do you endorse my characterization of your position above? “evolution should care a ton about intelligence in most niches, so if it were mostly about brain size then it should have gone up to human brain sizes with the dinosaurs”
in which case the disagreement is about how much evolution should care about intelligence in the dinosaur niche, vs other things it could put its skill points into?
Eliezer, it seems like chimps are insanely smart compared to other animals, basically as smart as they get
so it’s natural to think that the main things that make humans unique are also present in chimps
or at least, there was something going on in chimps that is exceptional
and should be causally upstream of the uniqueness of humans too
otherwise you have too many coincidences on your hands
ajeya: no, I’d characterize that as “the human environmental niche per se does not seem super-special enough to be unique on a geological timescale, the cognitive part of the niche derives from increased cognitive abilities in the first place and so can’t be used to explain where they got started, dinosaurs are larger than humans and would pay lower relative metabolic costs for added brain size and it is not the case that every species as large as humans was in an environment where they would not have benefited as much from a fixed increment of intelligence, hominids are probably distinguished from dinosaurs in having better neural algorithms that arose over intervening evolutionary time and therefore better returns in intelligence on synapses that are more costly to humans than to elephants or large dinosaurs”
I don’t understand how you can think that hominids are the special step relative to something earlier
or like, I can see how it’s consistent, but I don’t see what evidence or argument supports it
it seems like the short evolutionary time, and the fact that you also have to explain the exceptional qualities of other primates, cut extremely strongly against it
paul: indeed, the fact that dinosaurs didn’t see their brain sizes and intelligences ballooning, says there must be a lot of stuff hominids had that dinosaurs didn’t, explaining why hominids got much higher returns on intelligence per synapse. natural selection is enough of a smooth process that 95% of this stuff should’ve been in the last common ancestor of humans and chimps.
it seems like brain size basically just increases faster in the smarter animals? though I mostly just know about birds and primates
that is what you’d predict from smartness being about algorithms!
and it accelerates further and further within both lines
it’s what you’d expect if smartness is about algorithms and chimps and birds have good algorithms
if smartness was about brain size, smartness and brain size would increase faster in the larger animals or the ones whose successful members ate more food per day
well, sure, I do model that birds have better algorithms than dinosaurs
it seems like you’ve given arguments for “there was algorithmic innovation between dinosaurs and humans” but not yet arguments for “there was major algorithmic innovation between chimps and humans”?
(much less that the algorithmic changes were not just more-of-the-same)
oh, that’s not mandated by the model the same way. (between LCA of chimps and humans)
isn’t that exactly what we are discussing?
…I hadn’t thought so, no.
original q was:
On software beating hardware, how much of your view is dependent on your belief that the chimp -> human transition was probably not mainly about brain size because if it were about brain size it would have happened faster? My understanding is that you think the main change is a small software innovation which increased returns to having a bigger brain. If you changed your mind and thought that the chimp -> human transition was probably mostly about raw brain size, what (if anything) about your AI takeoff views would change?
so i thought we were talking about if there’s a cool innovation from chimp->human?
I can see how this would have been the more obvious intended interpretation on your viewpoint, and apologize
(though i think paul would also disagree about this thing, and believes that the chimp to human thing was mostly about size)
Is what I was responding to in part
I am open to saying that I’m conflating size and “algorithmic improvements that are closely correlated with size in practice and are similar to the prior algorithmic improvements amongst primates”
from my perspective, the question is “how did that hominid->human transition happen, as opposed to there being an elephant->smartelephant or dinosaur->smartdinosaur transition”?
I expect there were substantial numbers of brain algorithm stuffs going on during this time, however
because I don’t think that synapses scale that well with the baseline hominid boost
FWIW, it seems quite likely to me that there would be an elephant->smartelephant transition within tens of millions or maybe 100M years, and a dinosaur->smartdinosaur transition in hundreds of millions of years
and those are just cut off by the fastest lines getting there first
which I think does circle back to that point? actually I think my memory glitched and forgot the original point while being about this subpoint and I probably did interpret the original point as intended.
namely primates beating out birds by a hair
that sounds like a viewpoint which would also think it much more likely that GPT-3 would foom in a billion years
where maybe you think that’s unlikely, but I still get the impression your “unlikely” is, like, 5 orders of magnitude likelier than mine before applying overconfidence adjustments against extreme probabilities on both sides
yeah, I think I need to back up
Is your position something like “at some point after dinosaurs, there was an algorithmic innovation that increased returns to brain size, which meant that the birds and the humans see their brains increasing quickly while the dinosaurs didn’t”?
it also seems to me like the chimp->human difference is in basically the same ballpark of the effect of brain size within humans, given modest adaptations for culture
which seems like a relevant sanity-check that made me take the “mostly hardware” view more seriously
there’s a part of my model which very strongly says that hominids scaled better than elephants and that’s why “hominids->humans but not elephants->superelephants”
previously I had assumed that analysis would show that chimps were obviously way dumber than an extrapolation of humans
there’s another part of my model which says “and it still didn’t scale that well without algorithms, so we should expect a lot of alleles affecting brain circuitry which rose to fixation over the period when hominid brains were expanding”
this part is strong and I think echoes back to AGI stuff, but it is not as strong as the much more overdetermined position that hominids started with more scalable algorithms than dinosaurs.
I do agree with the point that there are structural changes in brains as you scale them up, and this is potentially a reason why brain size changes more slowly than e.g. bone size. (Also there are small structural changes in ML algorithms as you scale them up, not sure how much you want to push the analogy but they feel fairly similar.)
it also seems to me like the chimp->human difference is in basically the same ballpark of the effect of brain size within humans, given modest adaptations for culture
this part also seems pretty blatantly false to me
is there, like, a smooth graph that you looked at there?
I think the extrapolated difference would be about 4 standard deviations, so we are comparing a chimp to an IQ 40 human
I’m really not sure how much of a fair comparison that is
IQ 40 humans in our society may be mostly sufficiently-damaged humans, not scaled-down humans
doesn’t seem easy, but the point is that the extrapolated difference is huge, it corresponds to completely debilitating developmental problems
if you do enough damage to a human you end up with, for example, a coma victim who’s not competitive with other primates at all
yes, that’s more than 4 SD down
I agree with this general point
I’d guess I just have a lot more respect for chimps than you do
I feel like I have a bunch of respect for chimps but more respect for humans
like, that stuff humans do
that is really difficult stuff!
it is not just scaled-up chimpstuff!
Carl convinced me chimps wouldn’t go to space, but I still really think it’s about domesticity and cultural issues rather than intelligence
the chimpstuff is very respectable but there is a whole big layer cake of additional respect on top
not a prediction to be resolved until after the singularity
I mean, the space prediction isn’t very confident 🙂
and it involved a very large planet of apes
9.6. Architectural innovation in AI and in evolutionary history
I feel like if GPT-based systems saturate and require any architectural innovation rather than Stack More Layers to get much further, this is a pre-Singularity point of observation which favors humans probably being more qualitatively different from chimp-LCA
(LCA=last common ancestor)
any seems like a kind of silly bar?
because single architectural innovations are allowed to have large effects!
like there were already small changes to normalization from GPT-2 to GPT-3, so isn’t it settled?
natural selection can’t afford to deploy that many of them!
and the model really eventually won’t work if you increase layers but don’t fix the normalization, there are severe problems that only get revealed at high scale
that I wouldn’t call architectural innovation
transformers were
this is a place where I would not discuss specific ideas because I do not actually want this event to occur
sure
have you seen a graph of LSTM scaling vs transformer scaling?
I think LSTM with ongoing normalization-style fixes lags like 3x behind transformers on language modeling
no, does it show convergence at high-enough scales?
figure 7 here: https://arxiv.org/pdf/2001.08361.pdf
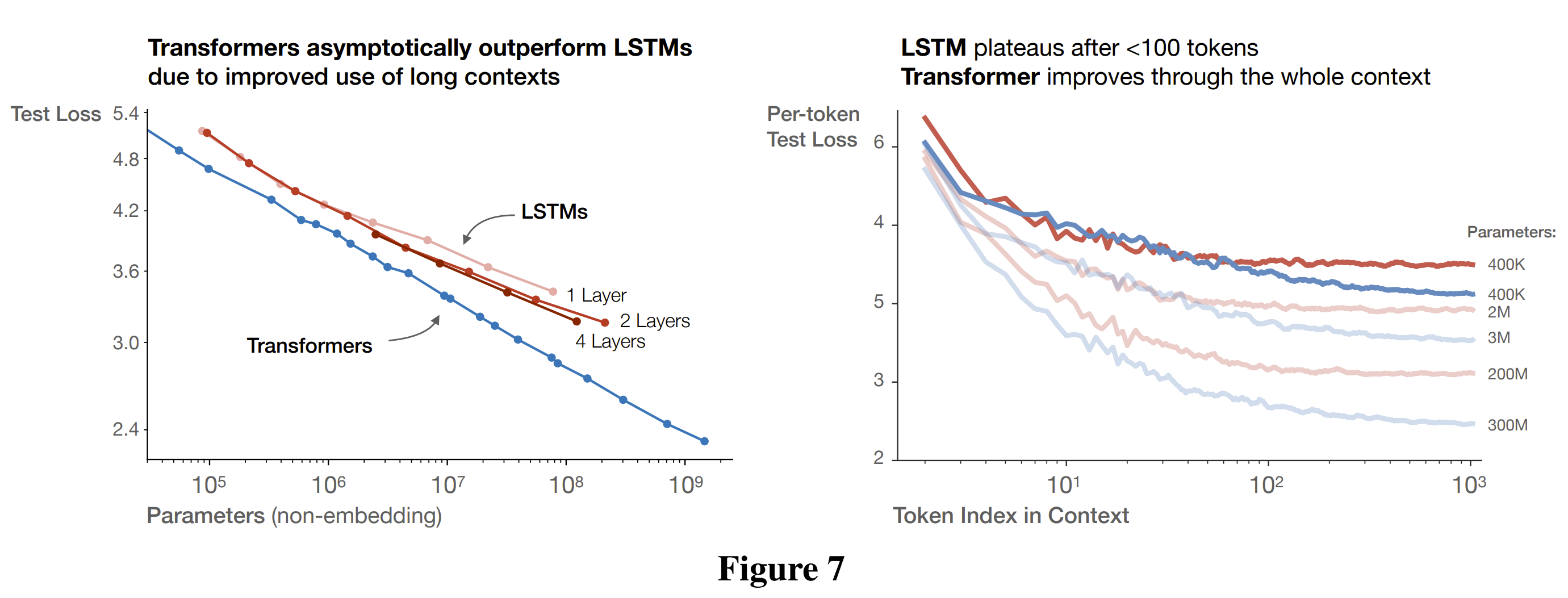
yeah… I unfortunately would rather not give other people a sense for which innovations are obviously more of the same and which innovations obviously count as qualitative
I think smart money is that careful initialization and normalization on the RNN will let it keep up for longer
anyway, I’m very open to differences like LSTM vs transformer between humans and 3x-smaller-brained-ancestors, as long as you are open to like 10 similar differences further back in the evolutionary history
what if there’s 27 differences like that and 243 differences further back in history?
sure
is that a distinctly Yudkowskian view vs a Paul view…
apparently not
I am again feeling confused about cruxes
I mean, 27 differences like transformer vs LSTM isn’t actually plausible, so I guess we could talk about it
Here’s a potential crux articulation that ties it back to the animals stuff: paul thinks that we first discover major algorithmic innovations that improve intelligence at a low level of intelligence, analogous to evolution discovering major architectural innovations with tiny birds and primates, and then there will be a long period of scaling up plus coming up with routine algorithmic tweaks to get to the high level, analogous to evolution schlepping on the same shit for a long time to get to humans. analogously, he thinks when big innovations come onto the scene the actual product is crappy af (e.g. wright brother’s plane), and it needs a ton of work to scale up to usable and then to great.
you both seem to think both evolution and tech history consiliently point in your direction
that sounds vaguely right, I guess the important part of “routine” is “vaguely predictable,” like you mostly work your way down the low-hanging fruit (including new fruit that becomes more important as you scale), and it becomes more and more predictable the more people are working on it and the longer you’ve been at it
and deep learning is already reasonably predictable (i.e. the impact of successive individual architectural changes is smaller, and law of large numbers is doing its thing) and is getting more so, and I just expect that to continue
yeah, like it’s a view that points to using data that relates effort to algorithmic progress and using that to predict future progress (in combination with predictions of future effort)
yeah
and for my part, it feels like this is how most technologies look and also how current ML progress looks
and also how evolution looks, right?
you aren’t seeing big jumps in translation or in self-driving cars or in image recognition, you are just seeing a long slog, and you see big jumps in areas where few people work (usually up to levels that are not in fact that important, which is very correlated with few people working there)
I don’t know much about evolution, but it at least looks very consistent with what I know and the facts eliezer cites
(not merely consistent, but “explains the data just about as well as the other hypotheses on offer”)
9.7. Styles of thinking in forecasting
I do observe that this would seem, on the surface of things, to describe the entire course of natural selection up until about 20K years ago, if you were looking at surface impacts
by 20k years ago I think it’s basically obvious that you are tens of thousands of years from the singularity
like, I think natural selection is going crazy with the brains by millions of years ago, and by hundreds of thousands of years ago humans are going crazy with the culture, and by tens of thousands of years ago the culture thing has accelerated and is almost at the finish line
really? I don’t know if I would have been able to call that in advance if I’d never seen the future or any other planets. I mean, maybe, but I sure would have been extrapolating way out onto a further limb than I’m going here.
Yeah, I agree singularity is way more out on a limb—or like, where the singularity stops is more uncertain since that’s all that’s really at issue from my perspective
but the point is that everything is clearly crazy in historical terms, in the same way that 2000 is crazy, even if you don’t know where it’s going
and the timescale for the crazy changes is tens of thousands of years
I frankly model that, had I made any such prediction 20K years ago of hominids being able to pull of moon landings or global warming – never mind the Singularity – I would have faced huge pushback from many EAs, such as, for example, Robin Hanson, and you.
like I think this can’t go on would have applied just as well: https://www.lesswrong.com/posts/5FZxhdi6hZp8QwK7k/this-can-t-go-on
I don’t think that’s the case at all
and I think you still somehow don’t understand my position?
https://www.lesswrong.com/posts/XQirei3crsLxsCQoi/surprised-by-brains is my old entry here
like, what is the move I’m making here, that you think I would have made in the past?
and would have led astray?
I sure do feel in a deeper sense that I am trying very hard to account for perspective shifts in how unpredictable the future actually looks at the time, and the Other is looking back at the past and organizing it neatly and expecting the future to be that neat
I don’t even feel like I’m expecting the future to be neat
are you just saying you have a really broad distribution over takeoff speed, and that “less than a month” gets a lot of probability because lots of numbers are less than a month?
not exactly?
in what way is your view the one that is preferred by things being messy or unpredictable?
like, we’re both agreeing X will eventually happen, and I’m making some concrete prediction about how some other X’ will happen first, and that’s the kind of specific prediction that’s likely to be wrong?
more like, we sure can tell a story today about how normal and predictable AlphaGo was, but we can always tell stories like that about the past. I do not particularly recall the AI field standing up one year before AlphaGo and saying “It’s time, we’re coming for the 8-dan pros this year and we’re gonna be world champions a year after that.” (Which took significantly longer in chess, too, matching my other thesis about how these slides are getting steeper as we get closer to the end.)
it’s more like, you are offering AGZ as an example of why things are crazy, and I’m doubtful / think it’s pretty lame
maybe I don’t understand how it’s functioning as bayesian evidence
for what over what
I feel like the whole smoothness-reasonable-investment view, if evaluated on Earth 5My ago without benefit of foresight, would have dismissed the notion of brains overtaking evolution; evaluated 1My ago, it would have dismissed the notion of brains overtaking evolution; evaluated 20Ky ago, it would have barely started to acknowledge that brains were doing anything interesting at all, but pointed out how the hominids could still only eat as much food as their niche offered them and how the cute little handaxes did not begin to compare to livers and wasp stings.
there is a style of thinking that says, “wow, yeah, people in the past sure were surprised by stuff, oh, wait, I’m also in the past, aren’t I, I am one of those people”
and a view where you look back from the present and think about how reasonable the past all seems now, and the future will no doubt be equally reasonable
(the AGZ example may fall flat, because the arguments we are making about it now we were also making in the past)
I am not sure this is resolvable, but it is among my primary guesses for a deep difference in believed styles of thought
I think that’s a useful perspective, but still don’t see how it favors your bottom line
where I look at the style of thinking you’re using, and say, not, “well, that’s invalidated by a technical error on line 3 even on Paul’s own terms” but “isn’t this obviously a whole style of thought that never works and ends up unrelated to reality”
I think the first AlphaGo was the larger shock, AlphaGo Zero was a noticeable but more mild shock on account of how it showed the end of game programming and not just the end of Go
sorry, I lumped them together
it didn’t feel like the same level of surprise; it was precedented by then
the actual accomplishment may have been larger in an important sense, but a lot of the – epistemic landscape of lessons learned? – is about the things that surprise you at the time
also AlphaGo was also quite easy to see coming after this paper (as was discussed extensively at the time): https://www.cs.toronto.edu/~cmaddis/pubs/deepgo.pdf
Paul, are you on the record as arguing with me that AlphaGo will win at Go because it’s predictably on-trend?
back then?
Hm, it sounds like Paul is saying “I do a trend extrapolation over long time horizons and if things seem to be getting faster and faster I expect they’ll continue to accelerate; this extrapolation if done 100k years ago would have seen that things were getting faster and faster and projected singularity within 100s of K years”
Do you think Paul is in fact doing something other than the trend extrap he says he’s doing, or that he would have looked at a different less informative trend than the one he says he would have looked at, or something else?
my methodology for answering that question is looking at LW comments mentioning go by me, can see if it finds any
Different less informative trend, is most of my suspicion there?
though, actually, I should revise that, I feel like relatively little of the WHA was AlphaGo v2 whose name I forget beating Lee Se-dol, and most was in the revelation that v1 beat the high-dan pro whose name I forget.
Paul having himself predicted anything at all like this would be the actually impressive feat
that would cause me to believe that the AI world is more regular and predictable than I experienced it as, if you are paying more attention to ICLR papers than I do
9.8. Moravec’s prediction
And jtbc, the trend extrap paul is currently doing is something like:
- Look at how effort leads to hardware progress measured in FLOP/$ and software progress measured in stuff like “FLOP to do task X” or “performance on benchmark Y”
- Look at how effort in the ML industry as a whole is increasing, project forward with maybe some adjustments for thinking markets are more inefficient now and will be less inefficient later
and this is the wrong trend, because he shouldn’t be looking at hardware/software progress across the whole big industry and should be more open to an upset innovation coming from an area with a small number of people working on it?
and he would have similarly used the wrong trends while trying to do trend extrap in the past?
because I feel like this general style of thought doesn’t work when you use it on Earth generally, and then fails extremely hard if you try to use it on Earth before humans to figure out where the hominids are going because that phenomenon is Different from Previous Stuff
like, to be clear, I have seen this used well on solar
I feel like I saw some people calling the big solar shift based on graphs, before that happened
I have seen this used great by Moravec on computer chips to predict where computer chips would be in 2012
and also witnessed Moravec completely failing as soon as he tried to derive literally anything but the graph itself namely his corresponding prediction for human-equivalent AI in 2012 (I think, maybe it was 2010) or something
(I think in his 1988 book Moravec estimated human-level AI in ~2030, not sure if you are referring to some earlier prediction?)
(I have seen Ray Kurzweil project out Moore’s Law to the $1,000,000 human brain in, what was it, 2025, followed by the $1000 human brain in 2035 and the $1 human brain in 2045, and when I asked Ray whether machine superintelligence might shift the graph at all, he replied that machine superintelligence was precisely how the graph would be able to continue on trend. This indeed is sillier than EAs.)
moravec’s prediction appears to actually be around 2025, looking at his hokey graph? https://jetpress.org/volume1/moravec.htm
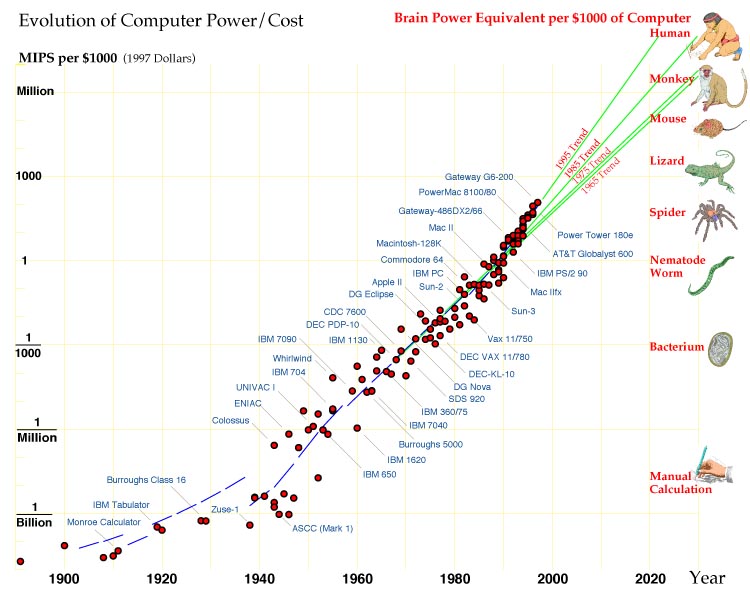
but even there, it does feel to me like there is a commonality between Kurzweil’s sheer graph-worship and difficulty in appreciating the graphs as surface phenomena that are less stable than deep phenomena, and something that Hanson was doing wrong in the foom debate
which is…like, your timelines?
that’s 1998
Mind Children in 1988 I am pretty sure had an earlier prediction
I should think you’d be happy to bet against me on basically any prediction, shouldn’t you?
any prediction that sounds narrow and isn’t like “this graph will be on trend in 3 more years”
…maybe I’m wrong, an online source says Mind Children in 1988 predicted AGI in “40 years” but I sure do seem to recall an extrapolated graph that reached “human-level hardware” in 2012 based on an extensive discussion about computing power to duplicate the work of the retina
don’t think it matters too much other than for Moravec’s honor, doesn’t really make a big difference for the empirical success of the methodology
I think it’s on page 68 if you have the physical book
p60 via Google Books says 10 teraops for a human-equivalent mind
I have a general read of history where trend extrapolation works extraordinarily well relative to other kinds of forecasting, to the extent that the best first-pass heuristic for whether a prediction is likely to be accurate is whether it’s a trend extrapolation and how far in the future it is
which, incidentally, strikes me as entirely plausible if you had algorithms as sophisticated as the human brain
my sense is that Moravec nailed the smooth graph of computing power going on being smooth, but then all of his predictions about the actual future were completely invalid on account of a curve interacting with his curve that he didn’t know things about and so simply omitted as a step in his calculations, namely, AGI algorithms
though again, from your perspective 2030 is still a reasonable bottom-line forecast that makes him one of the most accurate people at that time?
you could be right about all the local behaviors that your history is already shouting out at you as having smooth curve (where by “local” I do mean to exclude stuff like world GDP extrapolated into the indefinite future) and the curves that history isn’t shouting at you will tear you down
(I don’t know if he even forecast that)
I don’t remember that part from the 1988 book
my memory of the 1988 book is “10 teraops, based on what it takes to rival the retina” and he drew a graph of Moore’s Law
yeah, I think that’s what he did
(and got 2030)
“If this rate of improvement were to continue into the next century, the 10 teraops required for a humanlike computer would be available in a $10 million supercomputer before 2010 and in a $1,000 personal computer by 2030.”
or like, he says “human equivalent in 40 years” and predicts that in 50 years we will have robots with superhuman reasoning ability, not clear he’s ruling out human-equivalent AGI before 40 years but I think the tone is clear
so 2030 for AGI on a personal computer and 2010 for AGI on a supercomputer, and I expect that on my first reading I simply discarded the former prediction as foolish extrapolation past the model collapse he had just predicted in 2010.
(p68 in “Powering Up”)
yeah, that makes sense
I do think the PC number seems irrelevant
I think both in that book and in the 98 article he wants you to pay attention to the “very cheap human-size computers” threshold, not the “supercomputer” threshold, i think intentionally as a way to handwave in “we need people to be able to play around with these things”
(which people criticized him at the time for not more explicitly modeling iirc)
but! I mean! there are so many little places where the media has a little cognitive hiccup about that and decides in 1998 that it’s fine to describe that retrospectively as “you predicted in 1988 that we’d have true AI in 40 years” and then the future looks less surprising than people at the time using Trend Logic were actually surprised by it!
all these little ambiguities and places where, oh, you decide retroactively that it would have made sense to look at this Trend Line and use it that way, but if you look at what people said at the time, they didn’t actually say that!
I mean, in fairness reading the book it just doesn’t seem like he is predicting human-level AI in 2010 rather than 2040, but I do agree that it seems like the basic methodology (why care about the small computer thing?) doesn’t really make that much sense a priori and only leads to something sane if it cancels out with a weird view
9.9. Prediction disagreements and bets
anyway, I’m pretty unpersuaded by the kind of track record appeal you are making here
if the future goes the way I predict and yet anybody somehow survives, perhaps somebody will draw a hyperbolic trendline on some particular chart where the trendline is retroactively fitted to events including those that occurred in only the last 3 years, and say with a great sage nod, ah, yes, that was all according to trend, nor did anything depart from trend
trend lines permit anything
like from my perspective the fundamental question is whether I would do better or worse by following the kind of reasoning you’d advocate, and it just looks to me like I’d do worse, and I’d love to make any predictions about anything to help make that more clear and hindsight-proof in advance
you just look into the past and find a line you can draw that ended up where reality went
it feels to me like you really just waffle on almost any prediction about the before-end-of-days
I don’t think I know a lot about the before-end-of-days
like if you make a prediction I’m happy to trade into it, or you can pick a topic and I can make a prediction and you can trade into mine
but you know enough to have strong timing predictions, e.g. your bet with caplan
it’s daring enough that I claim to know anything about the Future at all!
surely with that difference of timelines there should be some pre-2030 difference as well
but you are the one making the track record argument against my way of reasoning about things!
how does that not correspond to believing that your predictions are better!
what does that mean?
yes and if you say something narrow enough or something that my model does at least vaguely push against, we should bet
my point is that I’m willing to make a prediction about any old thing, you can name your topic
I think the way I’m reasoning about the future is just better in general
and I’m going to beat you on whatever thing you want to bet on
but if you say, “well, Moore’s Law on trend, next 3 years”, then I’m like, “well, yeah, sure, since I don’t feel like I know anything special about that, that would be my prediction too”
sure
you can pick the topic
pick a quantity
or a yes/no question
or whatever
you may know better than I would where your Way of Thought makes strong, narrow, or unusual predictions
I’m going to trend extrapolation everywhere
spoiler
okay but any superforecaster could do that and I could do the same by asking a superforecaster
but there must be places where you’d strongly disagree w the superforecaster
since you disagree with them eventually, e.g. >2/3 doom by 2030
“>2/3 doom by 2030” isn’t an actual Eliezer-prediction, and is based on a misunderstanding of something Eliezer said. See Eliezer’s comment on LessWrong.
in the terminal phase, sure
right, but there are no disagreements before jan 1 2030?
no places where you’d strongly defy the superforecasters/trend extrap?
superforecasters were claiming that AlphaGo had a 20% chance of beating Lee Se-dol and I didn’t disagree with that at the time, though as the final days approached I became nervous and suggested to a friend that they buy out of a bet about that
what about like whether we get some kind of AI ability (e.g. coding better than X) before end days
though that was more because of having started to feel incompetent and like I couldn’t trust the superforecasters to know more, than because I had switched to a confident statement that AlphaGo would win
seems like EY’s deep intelligence / insight-oriented view should say something about what’s not possible before we get the “click” and the FOOM
I mean, I’m OK with either (i) evaluating arguments rather than dismissive and IMO totally unjustified track record, (ii) making bets about stuff
I don’t see how we can both be dismissing things for track record reasons and also not disagreeing about things
if our methodologies agree about all questions before end of days (which seems crazy to me) then surely there is no track record distinction between them…
[Cotra: 👍] |
do you think coding models will be able to 2x programmer productivity before end days? 4x?
what about hardware/software R&D wages? will they get up to $20m/yr for good ppl?
will someone train a 10T param model before end days?
things I’m happy to bet about: economic value of LMs or coding models at 2, 5, 10 years, benchmark performance of either, robotics, wages in various industries, sizes of various industries, compute/$, someone else’s views about “how ML is going” in 5 years
maybe the “any GDP acceleration before end of days?” works, but I didn’t like how you don’t win until the end of days
okay, so here’s an example place of a weak general Yudkowskian prediction, that is weaker than terminal-phase stuff of the End Days: (1) I predict that cycles of ‘just started to be able to do Narrow Thing -> blew past upper end of human ability at Narrow Thing’ will continue to get shorter, the same way that, I think, this happened faster with Go than with chess.
great, I’m totally into it
what’s a domain?
coding?
Does Paul disagree? Can Paul point to anything equally specific out of Paul’s viewpoint?
benchmarks for LMs?
robotics?
well, for these purposes, we do need some Elo-like ability to measure at all where things are relative to humans
problem-solving benchmarks for code?
MATH benchmark?
well, for coding and LM’ing we have lots of benchmarks we can use
this unfortunately does feel a bit different to me from Chess benchmarks where the AI is playing the whole game; Codex is playing part of the game
in general the way I’d measure is by talking about how fast you go from “weak human” to “strong human” (e.g. going from top-10,000 in chess to top-10 or whatever, going from jobs doable by $50k/year engineer to $500k/year engineer…)
golly, that sounds like a viewpoint very favorable to mine
what do you mean?
that way of measuring would be favorable to your viewpoint?
if we measure how far it takes AI to go past different levels of paying professionals, I expect that the Chess duration is longer than the Go duration and that by the time Codex is replacing a most paid $50k/year programmers the time to replacing a most programmers paid as much as a top Go player will be pretty darned short
top Go players don’t get paid, do they?
they tutor students and win titles
but I mean, they are like low-paid engineers
yeah that’s part of the issue here
I’m using wages as a way to talk about the distribution of human abilities, not the fundamental number
I would expect something similar to hold over going from low-paying welder to high-paying welder
like, how long to move from “OK human” to “pretty good human” to “best human”
says salary of $350k/yr for lee: https://www.fameranker.com/lee-sedol-net-worth
but I also mostly expect that AIs will not be allowed to weld things on Earth
why don’t we just do an in vitro benchmark instead of wages?
what, machines already do virtually all welding?
just pick a benchmark?
yoouuuu do not want to believe sites like that (fameranker)
yeah, I’m happy with any benchmark, and then we can measure various human levels at that benchmark
what about MATH? https://arxiv.org/abs/2103.03874
also I don’t know what “shorter and shorter” means, the time in go and chess was decades to move from “strong amateur” to “best human,” I do think these things will most likely be shorter than decades
seems like we can just predict concrete #s though
[Cotra: 👍] |
like I can say how long I think it will take to get from “median high schooler” to “IMO medalist” and you can bet against me?
and if we just agree about all of those predictions then again I’m back to being very skeptical of a claimed track record difference between our models
(I do think that it’s going to take years rather than decades on all of these things)
possibly! I worry this ends up in a case where Katja or Luke or somebody goes back and collects data about “amateur to pro performance times” and Eliezer says “Ah yes, these are shortening over time, just as I predicted” and Paul is like “oh, well, I predict they continue to shorten on this trend drawn from the data” and Eliezer is like “I guess that could happen for the next 5 years, sure, sounds like something a superforecaster would predict as default”
i’m pretty sure paul’s methodology here will just be to look at the MATH perf trend based on model size and combine with expectations of when ppl will make big enough models, not some meta trend thing like that?
so I feel like… a bunch of what I feel is the real disagreement in our models, is a bunch of messy stuff Suddenly Popping Up one day and then Eliezer is like “gosh, I sure didn’t predict that” and Paul is like “somebody could have totally predicted that” and Eliezer is like “people would say exactly the same thing after the world ended in 3 minutes”
if we’ve already got 2 years of trend on a dataset, I’m not necessarily going to predict the trend breaking
hm, you’re presenting your view as more uncertain and open to anything here than paul’s view, but in fact it’s picking out a narrower distribution. you’re more confident in powerful AGI soon
seems hard to play the “who is more confident?” game
so there should be some places where you make a strong positive prediction paul disagrees with
I might want to buy options on a portfolio of trends like that, if Paul is willing to sell me insurance against all of the trends breaking upward at a lower price than I think is reasonable
I mean, from my perspective Paul is the one who seems to think the world is well-organized and predictable in certain ways
yeah, and you are saying that I’m overconfident about that
I keep wanting Paul to go on and make narrower predictions than I do in that case
so you should be happy to bet with me about anything
and I’m letting you pick anything at all you want to bet about
i mean we could do a portfolio of trends like MATH and you could bet on at least a few of them having strong surprises in the sooner direction
but that means we could just bet about MATH and it’d just be higher variance
ok but you’re not going to sell me cheap options on sharp declines in the S&P 500 even though in a very reasonable world there would not be any sharp declines like that
if we’re betting $ rather than bayes points, then yes I’m going to weigh worlds based on the value of $ in those worlds
wouldn’t paul just sell you options at the price the options actually trade for? i don’t get it
but my sense is that I’m just generally across the board going to be more right than you are, and I’m frustrated that you just keep saying that “people like me” are wrong about stuff
Paul’s like “we’ll see smooth behavior in the end days” and I feel like I should be able to say “then Paul, sell me cheap options against smooth behavior now” but Paul is just gonna wanna sell at market price
and so I want to hold you to that by betting about anything
ideally just tons of stuff
random things about what AI will be like, and other technologies, and regulatory changes
paul’s view doesn’t seem to imply that he should value those options less than the market
he’s more EMH-y than you not less
but then the future should behave like that market
what do you mean?
it should have options on wild behavior that are not cheap!
you mean because people want $ more in worlds where the market drops a lot?
I don’t understand the analogy
no, because jumpy stuff happens more than it would in a world of ideal agents
I think EY is saying the non-cheap option prices are because P(sharp declines) is pretty high
ok, we know how often markets jump, if that’s the point of your argument can we just talk about that directly?
or sharp rises, for that matter
(much lower than option prices obviously)
I’m probably happy to sell you options for sharp rises
I’ll give you better than market odds in that direction
that’s how this works
now I am again confused, for I thought you were the one who expected world GDP to double in 4 years at some point
and indeed, drew such graphs with the rise suggestively happening earlier than the sharp spike
yeah, and I have exposure to that by buying stocks, options prices are just a terrible way of tracking these things
suggesting that such a viewpoint is generally favor to near timelines for that
I mean, I have bet a lot of money on AI companies doing well
well, not compared to the EA crowd, but compared to my meager net worth 🙂
and indeed, it has been true so far
and I’m continuing to make the bet
it seems like on your view it should be surprising that AI companies just keep going up
aren’t you predicting them not to get to tens of trillions of valuation before the end of days?
I believe that Nate, of a generally Yudkowskian view, did the same (bought AI companies). and I focused my thoughts elsewhere, because somebody needs to, but did happen to buy my first S&P 500 on its day of exact minimum in 2020
point is, that’s how you get exposure to the crazy growth stuff with continuous ramp-ups
and I’m happy to make the bet on the market
or on other claims
I don’t know if my general vibe makes sense here, and why it seems reasonable to me that I’m just happy to bet on anything
as a way of trying to defend my overall attack
and that if my overall epistemic approach is vulnerable to some track record objection, then it seems like it ought to be possible to win here
9.10. Prediction disagreements and bets: Standard superforecaster techniques
I’m still kind of surprised that Eliezer isn’t willing to bet that there will be a faster-than-Paul expects trend break on MATH or whatever other benchmark. Is it just the variance of MATH being one benchmark? Would you make the bet if it were 6?
a large problem here is that both of us tend to default strongly to superforecaster standard techniques
it’s true, though it’s less true for longer things
but you think the superforecasters would suck at predicting end days because of the surface trends thing!
before I bet against Paul on MATH I would want to know that Paul wasn’t arriving at the same default I’d use, which might be drawn from trend lines there, or from a trend line in trend lines
I mean the superforecasters did already suck once in my observation, which was AlphaGo, but I did not bet against them there, I bet with them and then updated afterwards
I’d mostly try to eyeball how fast performance was improving with size; I’d think about difficulty effects (where e.g. hard problems will be flat for a while and then go up later, so you want to measure performance on a spectrum of difficulties)
what if you bet against a methodology instead of against paul’s view? the methodology being the one i described above, of looking at the perf based on model size and then projecting model size increases by cost?
seems safer to bet against my view
yeah
mostly I’d just be eyeballing size, thinking about how much people will in fact scale up (which would be great to factor out if possible), assuming performance trends hold up
are there any other examples of surface trends vs predictable deep changes, or is AGI the only one?
(that you have thought a lot about)
yeah seems even better to bet on the underlying “will the model size to perf trends hold up or break upward”
so from my perspective, there’s this whole thing where unpredictably something breaks above trend because the first way it got done was a way where somebody could do it faster than you expected
(makes sense for it to be the domain where you’ve thought a lot)
you mean, it’s unpredictable what will break above trend?
IEM has a financial example
I mean that I could not have said “Go will break above trend” in 2015
yeah
ok, here’s another example
it feels like if I want to make a bet with imaginary Paul in 2015 then I have to bet on a portfolio
and I also feel like as soon as we make it that concrete, Paul does not want to offer me things that I want to bet on
because Paul is also like, sure, something might break upward
I remark that I have for a long time been saying that I wish Paul had more concrete images and examples attached to a lot of his stuff
surely the view is about the probability of each thing breaking upward. or the expected number from a basket
I mean, if you give me any way of quantifying how much stuff breaks upwards we have a bet
not literally that one single thing breaks upward
I don’t understand how concreteness is an accusation here, I’ve offered 10 quantities I’d be happy to bet about, and also allowed you to name literally any other quantity you want
and I agree that we mostly agree about things
and some of my sense here is that if Paul offered a portfolio bet of this kind, I might not take it myself, but EAs who were better at noticing their own surprise might say, “Wait, that’s how unpredictable Paul thinks the world is?”
so from my perspective, it is hard to know specific anti-superforecaster predictions that happen long before terminal phase, and I am not sure we are really going to get very far there.
but you agree that the eventual prediction is anti-superforecaster?
both of us probably have quite high inhibitions against selling conventionally priced options that are way not what a superforecaster would price them as
why does it become so much easier to know these things and go anti-superforecaster at terminal phase?
I assume you think that the superforecasters will continue to predict that big impactful AI applications are made by large firms spending a lot of money, even through the end of days
I do think it’s very often easy to beat superforecasters in-domain
like I expect to personally beat them at most ML prediction
and so am also happy to do bets where you defer to superforecasters on arbitrary questions and I bet against you
well, they’re anti-prediction-market in the sense that, at the very end, bets can no longer settle. I’ve been surprised of late by how much AGI ruin seems to be sneaking into common knowledge; perhaps in the terminal phase the superforecasters will be like, “yep, we’re dead”. I can’t even say that in this case, Paul will disagree with them, because I expect the state on alignment to be so absolutely awful that even Paul is like “You were not supposed to do it that way” in a very sad voice.
I’m just thinking about takeoff speeds here
I do think it’s fairly likely I’m going to be like “oh no this is bad” (maybe 50%?), but not that I’m going to expect fast takeoff
and similarly for the superforecasters
9.11. Prediction disagreements and bets: Late-stage predictions, and betting against superforecasters
so, one specific prediction you made, sadly close to terminal phase but not much of a surprise there, is that the world economy must double in 4 years before the End Times are permitted to begin
well, before it doubles in 1 year…
I think most people would call the 4 year doubling the end times
this seems like you should also be able to point to some least impressive thing that is not permitted to occur before WGDP has doubled in 4 years
and it means that the normal planning horizon includes the singularity
it may not be much but we would be moving back the date of first concrete disagreement
I can list things I don’t think would happen first, since that’s a ton
and EAs might have a little bit of time in which to say “Paul was falsified, uh oh”
the only things that aren’t permitted are the ones that would have caused the world economy to double in 4 years
and by the same token, there are things Eliezer thinks you are probably not going to be able to do before you slide over the edge. a portfolio of these will have some losing options because of adverse selection against my errors of what is hard, but if I lose more than half the portfolio, this may said to be a bad sign for Eliezer.
(though those can happen at the beginning of the 4 year doubling)
this is unfortunately late for falsifying our theories but it would be progress on a kind of bet against each other
but I feel like the things I’ll say are like fully automated construction of fully automated factories at 1-year turnarounds, and you’re going to be like “well duh”
…unfortunately yes
the reason I like betting about numbers is that we’ll probably just disagree on any given number
I don’t think I know numbers.
it does seem like a drawback that this can just turn up object-level differences in knowledge-of-numbers more than deep methodological advantages
the last important number I had a vague suspicion I might know was that Ethereum ought to have a significantly larger market cap in pre-Singularity equilibrium.
and I’m not as sure of that one since El Salvador supposedly managed to use Bitcoin L2 Lightning.
(though I did not fail to act on the former belief)
do you see why I find it weird that you think there is this deep end-times truth about AGI, that is very different from a surface-level abstraction and that will take people like Paul by surprise, without thinking there are other facts like that about the world?
I do see how this annoying situation can come about
and I also understand the symmetry of the situation
we unfortunately both have the belief that the present world looks a lot like our being right, and therefore that the other person ought to be willing to bet against default superforecasterish projections
paul says that he would bet against superforecasters too though
I would in ML
like, where specifically?
or on any other topic where I can talk with EAs who know about the domain in question
I don’t know if they have standing forecasts on things, but e.g.: (i) benchmark performance, (ii) industry size in the future, (iii) how large an LM people will train, (iv) economic impact of any given ML system like codex, (v) when robotics tasks will be plausible
I have decided that, as much as it might gain me prestige, I don’t think it’s actually the right thing for me to go spend a bunch of character points on the skills to defeat superforecasters in specific domains, and then go around doing that to prove my epistemic virtue.
that seems fair
you don’t need to bet with me to prove your epistemic virtue in this way, though
okay, but, if I’m allowed to go around asking Carl Shulman who to ask in order to get the economic impact of Codex, maybe I can also defeat superforecasters.
I think the deeper disagreement is that (i) I feel like my end-of-days prediction is also basically just a default superforecaster prediction (and if you think yours is too then we can bet about what some superforecasters will say on it), (ii) I think you are leveling a much stronger “people like paul get taken by surprise by reality” claim whereas I’m just saying that I don’t like your arguments
it seems to me like the contest should be more like our intuitions in advance of doing that
yeah, I think that’s fine, and also cheaper since research takes so much time
I feel like those asymmetries are pretty strong though
9.12. Self-duplicating factories, AI spending, and Turing test variants
so, here’s an idea that is less epistemically virtuous than our making Nicely Resolvable Bets
what if we, like, talked a bunch about our off-the-cuff senses of where various AI things are going in the next 3 years
and then 3 years later, somebody actually reviewed that
I do think just saying a bunch of stuff about what we expect will happen so that we can look back on it would have a significant amount of the value
and any time the other person put a thumbs-up on the other’s prediction, that prediction coming true was not taken to distinguish them
i’d suggest doing this in a format other than discord for posterity
even if the originator was like HOW IS THAT ALSO A PREDICTION OF YOUR THEORY
well, Discord has worked better than some formats
something like a spreadsheet seems easier for people to look back on and score and stuff
discord transcripts are pretty annoying to read
something like a spreadsheet seems liable to be high-cost and not actually happen
I think a conversation is probably easier and about as good for our purposes though?
ok fair
I think money can be inserted into humans in order to turn Discord into spreadsheets
and it’s possible we will both think we are right in retrospect
and that will also be revealing
but, besides that, I do want to boop on the point that I feel like Paul should be able to predict intuitively, rather than with necessity, things that should not happen before the world economy doubled in 4 years
it may also turn up some quantitative differences of view
there are lots of things I think won’t happen before the world economy has doubled in 4 years
because on my model, as we approach the end times, AI was still pretty partial and also the world economy was lolnoping most of the inputs a sensible person would accept from it and prototypes weren’t being commercialized and stuff was generally slow and messy
prototypes of factories building factories in <2 years
“AI was still pretty partial” leads it to not do interesting stuff that Paul can rule out
like I guess I think tesla will try, and I doubt it will be just tesla
but the other parts of that permit AI to do interesting stuff that Paul can rule out
automated researchers who can do ML experiments from 2020 without human input
okay, see, that whole “factories building factories” thing just seems so very much after the End Times to me
yeah, we should probably only talk about cognitive work
since you think physical work will be very slow
okay but not just that, it’s a falsifiable prediction
it is something that lets Eliezer be wrong in advance of the End Times
what’s a falsifiable prediction?
if we’re in a world where Tesla is excitingly gearing up to build a fully self-duplicating factory including its mining inputs and chips and solar panels and so on, we’re clearly in the Paulverse and not in the Eliezerverse!
yeah
I do think we’ll see that before the end times
just not before 4 year doublings
this unfortunately only allows you to be right, and not for me to be right, but I think there are also things you legit only see in the Eliezerverse!
I mean, I don’t think they will be doing mining for a long time because it’s cheap
they are unfortunately late in the game but they exist at all!
and being able to state them is progress on this project!
but fully-automated factories first, and then significant automation of the factory-building process
I do expect to see
I’m generally pretty bullish on industrial robotics relative to you I think, even before the crazy stuff?
but you might not have a firm view
like I expect to have tons of robots doing all kinds of stuff, maybe cutting human work in manufacturing 2x, with very modest increases in GDP resulting from that in particular
so, like, it doesn’t surprise me very much if Tesla manages to fully automate a factory that takes in some relatively processed inputs including refined metals and computer chips, and outputs a car? and by the same token I expect that has very little impact on GDP.
refined metals are almost none of the cost of the factory
and also tesla isn’t going to be that vertically integrated
the fabs will separately continue to be more and more automated
I expect to have robot cars driving everywhere, and robot trucks
another 2x fall in humans required for warehouses
elimination of most brokers involved in negotiating shipping
if despite the fabs being more and more automated, somehow things are managing not to cost less and less, and that sector of the economy is not really growing very much, is that more like the Eliezerverse than the Paulverse?
most work in finance and loan origination
though this is something of a peripheral prediction to AGI core issues
yeah, I think if you cut the humans to do X by 2, but then the cost falls much less than the number you’d naively expect (from saving on the human labor and paying for the extra capital), then that’s surprising to me
I mean if it falls half as much as you’d expect on paper I’m like “that’s a bit surprising” rather than having my mind blown, if it doesn’t fall I’m more surprised
but that was mostly physical economy stuff
oh wait, I was making positive predictions now, physical stuff is good for that I think?
since you don’t expect it to happen?
…this is not your fault but I wish you’d asked me to produce my “percentage of fall vs. paper calculation” estimate before you produced yours
my mind is very whiffy about these things and I am not actually unable to deanchor on your estimate 😦
makes sense, I wonder if I should just spoiler
one benefit of discord
yeah that works too!
a problem for prediction is that I share some background view about insane inefficiency/inadequacy/decadence/silliness
so these predictions are all tampered by that
but still seem like there are big residual disagreements
sighgreat
since you have way more of that than I do
not your fault but
I think that the AGI stuff is going to be a gigantic megaproject despite that
I am not shocked by the AGI stuff being a gigantic megaproject
it’s not above the bar of survival but, given other social optimism, it permits death with more dignity than by other routes
what if spending is this big:
Google invests $100B training a model, total spending across all of industry is way bigger
ooooh
I do start to be surprised if, come the end of the world, AGI is having more invested in it than a TSMC fab
though, not… super surprised?
also I am at least a little surprised before then
actually I should probably have been spoiling those statements myself but my expectation is that Paul’s secret spoiler is about
$10 trillion dollars or something equally totally shocking to an Eliezer
my view on that level of spending is
it’s an only slightly high-end estimate for spending by someone on a single model, but that in practice there will be ways of dividing more across different firms, and that the ontology of single-model will likely be slightly messed up (e.g. by OpenAI Five-style surgery). Also if it’s that much then it likely involves big institutional changes and isn’t at google.
I read your spoiler
my estimate for total spending for the whole project of making TAI, including hardware and software manufacturing and R&d, the big datacenters, etc.
is in the ballpark of $10T, though it’s possible that it will be undercounted several times due to wage stickiness for high-end labor
I think that as
spending on particular AGI megaprojects starts to go past $50 billion, it’s not especially ruled out per se by things that I think I know for sure, but I feel like a third-party observer should justly start to weakly think, ‘okay, this is looking at least a little like the Paulverse rather than the Eliezerverse’, and as we get to $10 trillion, that is not absolutely ruled out by the Eliezerverse but it was a whoole lot more strongly predicted by the Paulverse, maybe something like 20x unless I’m overestimating how strongly Paul predicts that
Proposed modification to the “speculate about the future to generate kind-of-predictions” methodology: we make shit up, then later revise based on points others made, and maybe also get Carl to sanity-check and deciding which of his objections we agree with. Then we can separate out the “how good are intuitions” claim (with fast feedback) from the all-things-considered how good was the “prediction”
okay that hopefully allows me to read Paul’s spoilers… no I’m being silly. @ajeya please read all the spoilers and say if it’s time for me to read his
you can read his latest
I’d guess it’s fine to read all of them?
yeah sorry that’s what i meant
what should I say more about before reading earlier ones?
ah k
My $10T estimate was after reading yours (didn’t offer an estimate on that quantity beforehand), though that’s the kind of ballpark I often think about, maybe we should just spoiler only numbers so that context is clear 🙂
I think fast takeoff gets significantly more likely as you push that number down
so, may I now ask what starts to look to you like “oh damn I am in the Eliezerverse”?
big mismatches between that AI looks technically able to do and what AI is able to do, though that’s going to need a lot of work to operationalize
I think low growth of AI overall feels like significant evidence for Eliezerverse (even if you wouldn’t make that prediction), since I’m forecasting it rising to absurd levels quite fast whereas your model is consistent with it staying small
some intuition about AI looking very smart but not able to do much useful until it has the whole picture, I guess this can be combined with the first point to be something like—AI looks really smart but it’s just not adding much value
all of those seem really hard
strong upward trend breaks on benchmarks seems like it should be a point toward eliezer verse, even if eliezer doesn’t want to bet on a specific one?
especially breaks on model size -> perf trends rather than calendar time trends
I think that any big break on model size -> perf trends are significant evidence
meta-learning working with small models?
e.g. model learning-to-learn video games and then learning a novel one in a couple subjective hours
I think algorithmic/architectural changes that improve loss as much as 10x’ing model, for tasks that looking like they at least should have lots of economic value
(even if they don’t end up having lots of value because of deployment bottlenecks)
is the meta-learning thing an Eliezer prediction?
(before the end-of-days)
no but it’d be an anti-bio-anchor positive trend break and eliezer thinks those should happen more than we do
fair enough
a lot of these things are about # of times that it happens rather than whether it happens at all
yeah
but meta-learning is special as the most plausible long horizon task
e.g. maybe in any given important task I expect a single “innovation” that’s worth 10x model size? but that it still represents a minority of total time?
hm, AI that can pass a competently administered turing test without being economically valuable?
that’s one of the things I think is ruled out before 4 year doubling, though Eliezer probably also doesn’t expect it
[Yudkowsky: 👍] |
what would this test do to be competently administered? like casual chatbots seem like they have reasonable probability of fooling someone for a few mins now
I think giant google-automating-google projects without big external economic impacts
would it test knowledge, or just coherence of some kind?
it’s like a smart-ish human (say +2 stdev at this task) trying to separate out AI from smart-ish human, iterating a few times to learn about what works
I mean, the basic ante is that the humans are trying to win a turing test, without that I wouldn’t even call it a turing test
dunno if any of those are compelling @Eliezer
something that passes a like “are you smart?” test administered by a human for 1h, where they aren’t trying to specifically tell if you are AI
just to see if you are as smart as a human
I mean, I guess the biggest giveaway of all would be if there is human-level (on average) AI as judged by us, but there’s no foom yet
I think we both don’t expect that one before the End of Days?
or like, no crazy economic impact
I think we both expect that to happen before foom?
but the “on average” is maybe way too rough a thing to define
oh, wait, I missed that it wasn’t the full Turing Test
well, I suggested both
the lamer one is more plausible
full Turing Test happeneth not before the End Times, on Eliezer’s view, and not before the first 4-year doubling time, on Paul’s view, and the first 4-year doubling happeneth not before the End Times, on Eliezer’s view, so this one doesn’t seem very useful
9.13. GPT-n and small architectural innovations vs. large ones
I feel like the biggest subjective thing is that I don’t feel like there is a “core of generality” that GPT-3 is missing
I just expect it to gracefully glide up to a human-level foom-ing intelligence
the “are you smart?” test seems perhaps passable by GPT-6 or its kin, which I predict to contain at least one major architectural difference over GPT-3 that I could, pre-facto if anyone asked, rate as larger than a different normalization method
but by fooling the humans more than by being smart
like I expect GPT-5 would foom if you ask it but take a long time
that sure is an underlying difference
not sure how to articulate what Eliezer expects to see here though
or like what the difference is
something that GPT-5 or 4 shouldn’t be able to do, according to eliezer?
where Paul is like “sure it could do that”?
I feel like GPT-3 clearly has some kind of “doesn’t really get what’s going on” energy
and I expect that to go away
well before the end of days
so that it seems like a kind-of-dumb person
I expect it to go away before the end of days
but with there having been a big architectural innovation, not Stack More Layers
yeah
whereas I expect layer stacking + maybe changing loss (since logprob is too noisy) is sufficient
if you name 5 possible architectural innovations I can call them small or large
1. replacing transformer attention with DB nearest-neighbor lookup over an even longer context
okay 1’s a bit borderline
2. adding layers that solve optimization problems internally (i.e. the weights and layer N activations define an optimization problem, the layer N+1 solves it) or maybe simulates an ODE
if it’s 3x longer context, no biggie, if it’s 100x longer context, more of a game-changer
2 – big change
I’m imagining >100x if you do that
3. universal transformer XL, where you reuse activations from one context in the next context (RNN style) and share weights across layers
I do not predict 1 works because it doesn’t seem like an architectural change that moves away from what I imagined to be the limits, but it’s a big change if it 100xs the window
3 – if it is only that single change and no others, I call it not a large change relative to transformer XL. Transformer XL itself however was an example of a large change – it didn’t have a large effect but it was what I’d call a large change.
4. Internal stochastic actions trained with reinforce
I mean, is mixture of experts or switch another big change?
are we just having big changes non-stop?
4 – I don’t know if I’m imagining right but it sounds large
it sounds from these definitions like the current rate of big changes is > 1/year
5 – mixture of experts: as with 1, I’m tempted to call it a small change, but that’s because of my model of it as doing the same thing, not because it isn’t in a certain sense a quite large move away from Stack More Layers
I mean, it is not very hard to find a big change to try?
finding a big change that works is much harder
several of these are improvements
one gets a minor improvement from a big change rather more often than a big improvement from a big change
that’s why dinosaurs didn’t foom
like transformer -> MoE -> switch transformer is about as big an improvement as LSTM vs transformer
so if we all agree that big changes are happening multiple times per year, then I guess that’s not the difference in prediction
is it about the size of gains from individual changes or something?
or maybe: if you take the scaling laws for transformers, are the models with impact X “on trend,” with changes just keeping up or maybe buying you 1-2 oom of compute, or are they radically better / scaling much better?
that actually feels most fundamental
I had not heard that transformer -> switch transformer was as large an improvement as lstm -> transformers after a year or two, though maybe you’re referring to a claimed 3x improvement and comparing that to the claim that if you optimize LSTMs as hard as transformers they come within 3x (I have not examined these claims in detail, they sound a bit against my prior, and I am a bit skeptical of both of them)
so remember that from my perspective, I am fighting an adverse selection process and the Law of Earlier Success
I think it’s actually somewhat smaller
if you treat GPT-3 as a fixed thingy and imagine scaling it in the most straightforward possible way, then I have a model of what’s going on in there and I don’t think that most direct possible way of scaling gets you past GPT-3 lacking a deep core
somebody can come up and go, “well, what about this change that nobody tried yet?” and I can be like, “ehhh, that particular change does not get at what I suspect the issues are”
I feel like the framing is: paul says that something is possible with “stack more layers” and eliezer isn’t. We both agree that you can’t literally stack more layers and have to sometimes make tweaks, and also that you will scale faster if you make big changes. But it seems like for Paul that means (i) changes to stay on the old trend line, (ii) changes that trade off against modest amounts of compute
so maybe we can talk about that?
when it comes to predicting what happens in 2 years, I’m not just up against people trying a broad range of changes that I can’t foresee in detail, I’m also up against a Goodhart’s Curse on the answer being a weird trick that worked better than I would’ve expected in advance
but then it seems like we may just not know, e.g. if we were talking lstm vs transformer, no one is going to run experiments with the well-tuned lstm because it’s still just worse than a transformer (though they’ve run enough experiments to know how important tuning is, and the brittleness is much of why no one likes it)
I would not have predicted Transformers to be a huge deal if somebody described them to me in advance of having ever tried it out. I think that’s because predicting the future is hard not because I’m especially stupid.
I don’t feel like anyone could predict that being a big deal
but I do think you could predict “there will be some changes that improve stability / make models slightly better”
(I mean, I don’t feel like any of the actual humans on earth could have, some hypothetical person could)
whereas what I’m trying to predict is more like “GPT-5 in order to start-to-awaken needs a change via which it, in some sense, can do a different thing, that is more different than the jump from GPT-1 to GPT-3; and examples of things with new components in them abound in Deepmind, like Alpha Zero having not the same architecture as the original AlphaGo; but at the same time I’m also trying to account for being up against this very adversarial setup where a weird trick that works much better than I expect may be the thing that makes GPT-5 able to do a different thing”
this may seem Paul-unfairish because any random innovations that come along, including big changes that cause small improvements, would tend to be swept up into GPT-5 even if they made no more deep difference than the whole thing with MoE
so it’s hard to bet on
but I also don’t feel like it – totally lacks Eliezer-vs-Paul-ness if you let yourself sort of relax about that and just looked at it?
also I’m kind of running out of energy, sorry
I think we should be able to get something here eventually
seems good to break though
that was a lot of arguing for one day
Did you like this post? You may enjoy our other Analysis, Conversations posts, including: