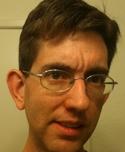
Luke Muehlhauser: One interesting feature of your own thinking (Hayworth 2012) about whole brain emulation (WBE) is that you are more concerned with modeling high-level cognitive functions accurately than is e.g. Sandberg (2013). Whereas Sandberg expects WBE will be achieved by modeling low-level brain function in exact detail (at the level of scale separation, wherever that is), you instead lean heavily on modeling higher-level cognitive processes using a cognitive architecture called ACT-R. Is that because you think this will be easier than Sandberg’s approach, or for some other reason?
Kenneth Hayworth: I think the key distinction is that philosophers are focused on whether mind uploading (a term I prefer to WBE) is possible in principle, and, to a lesser extent, on whether it is of such technical difficulty as to put its achievement off so far into the future that its possibility can be safely ignored for today’s planning. With these motivations, philosophers tend to gravitate toward arguments with the fewest possible assumptions, i.e. modeling low-level brain functions in exact detail.
As a practicing cognitive and neuroscientist I have fundamentally different motivations. From my training, I am already totally convinced that the functioning of the brain can be understood at a fully mechanistic level, with sufficient precision to allow for mind uploading. I just want to work toward making mind uploading happen in reality. To do this I need to start with an understanding of the requirements, not based on the fewest assumptions, but instead based on the field’s current best theories.
To use an analogy, before airplanes were invented one could argue that heavier-than-air flying machines must be possible in principle even if it meant copying a bird’s flapping motions etc. This is a sound philosophical argument but not one that engineers like the Wright Brothers would focus on. Instead they needed to know the crucial elements necessary for heavier-than-air flight –lift, drag, thrust. Understanding these allowed them to start building and testing, refining their theories and engineering.
If we want to create the technology to upload someone’s mind into a computer simulation and have that simulation have the same memories, intelligence, personality, and consciousness as the original, then we need to start with a top-level understanding of these cognitive functions. The place to look for these is the field of cognitive science and to highly researched computational models of intelligent behavior such as ACT-R.
I have focused my research on understanding how the computational elements of cognitive models such as ACT-R (symbolic representations, production rules, etc.) are likely mapped onto the neural circuits in the brain. This has led me to concrete predictions on exactly what technologies would be necessary to preserve, scan, and simulate a person’s brain. Those are the technologies I am currently working on.
Luke Muehlhauser: How widely used or accepted is ACT-R? Just looking through the literature, it doesn’t seem particularly dominant in cognitive neuroscience, though perhaps that’s just because no high-level model of its kind is dominant. E.g. ACT-R doesn’t seem to be mentioned in the 1100+ pages of the new Oxford Handbook of Cognitive Neuroscience, nor in the 1800+ pages of the Encyclopedia of Behavioral Neuroscience. I rarely see mention of ACT-R by people who aren’t its proponents.
Kenneth Hayworth: Looking at the main website for ACT-R I count a total of 1,035 individual publications related to ACT-R theory or using the ACT-R modeling framework. This publication record comes from hundreds of researchers and stretches back decades and continues strong today. ACT-R would not be considered a “cognitive neuroscience” theory since it does not talk about neurons (although there have been attempts to map ACT-R onto a neural framework which I will discuss). Instead, ACT-R is properly classified in the field of Cognitive Science. Of course there is a messy overlap across the fields of computer science, psychology, neuroscience, neurology, cognitive science, artificial intelligence, computational neuroscience, etc. and ACT-R certainly was designed with constraints from these various fields in mind.
Cognitive science can best be distinguished from neuroscience based on the typical level of abstraction of its models of intelligent behavior. Cognitive science is committed to modeling the mind as a computational system in the traditional sense of processing analog and symbolic representations of various complexity via algorithms. In general, cognitive science models of human intelligent behavior are couched in representational and algorithmic form. For example, a model of human attention and memory retention effects in a perceptual experiment might posit that our mind contains 4 buffers which can be used to store symbolic tokens representing individual letters that were briefly flashed on a viewing screen to the subject. Notice that cognitive science models like this do not talk about neurons or even brain regions, yet they make concrete testable predictions about behavioral responses, response timing, error rates, learning rates, etc. –effects which can be tested with great detail and precision in psychophysical experiments.
If you are looking for explanations for how humans understand natural language, or how they solve problems and reason about goals, or, crucially, how the mind creates and maintains a “self model” underlying our consciousness then cognitive science models at this level of abstraction are really the only place to look. Neuroscience should be seen as talking about the implementation details underlying the algorithms and representations assumed by such cognitive science models.
Now cognitive science as a field has been around for a long time and has been generating an enormous variety of small models and algorithmic hypotheses for how humans perform various actions. Way back in 1973 the great cognitive scientist Allen Newell wrote a paper called “You can’t play 20 questions with nature and win” in which he pointed out to the cognitive science community that it needed to strive for “unified theories of cognition” which were designed to explain not just one experimental result but all of them simultaneously. He proposed a particular computational formalism called a “production system” that could provide the basis for such a unification of different cognitive science models. He and others developed that proposal into the widely used SOAR model of human cognition (see his book “Unified Theories of Cognition” for a complete explanation of production systems as a model of the human mind).
There have been many production system models of the human mind based upon this original work but Anderson’s ACT-R theory is currently the standard-bearer of this class. As such, ACT-R should not really be thought of as just another computational model, instead you should think of ACT-R as attempting a summary of all results from the entire field of cognitive science –a summary in the form of a general computational framework for the human mind. Below is a screen capture of the ACT-R website which keeps a list of all publications and ACT-R models generated over the years. You can see that the categories span the entire field of cognitive science (e.g. language processing, learning, perception, memory, problem solving, decision making, etc.)
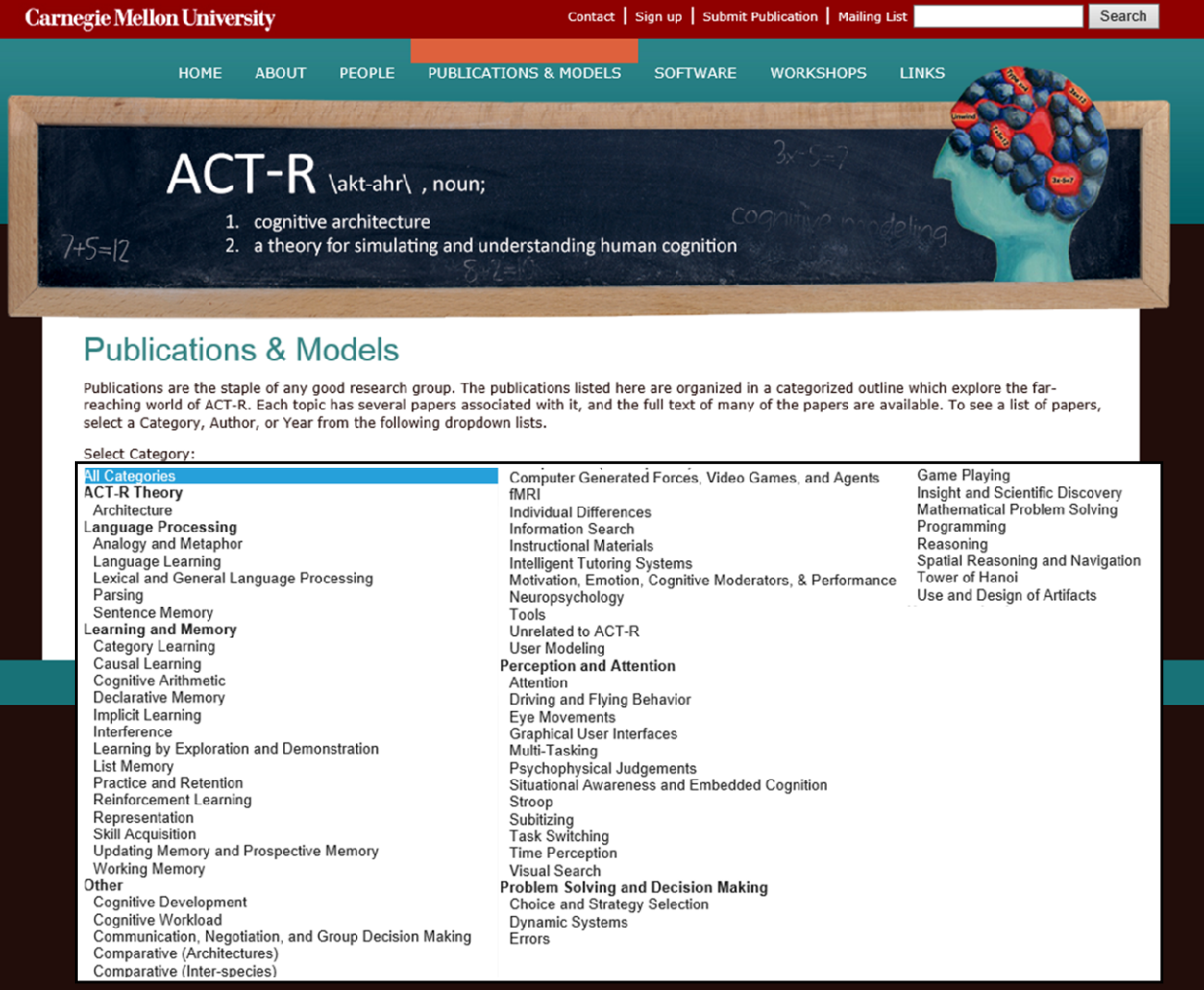
Also you should note that since ACT-R is a computational framework, models built within the ACT-R framework really “work” in the sense that an ACT-R model of sentence parsing will actually parse sentences.
I hear you when you say “[ACT-R] doesn’t seem particularly dominant in cognitive neuroscience”. This is absolutely true. Most of the neuroscientists I have met have never heard of ACT-R or production systems and usually are unfamiliar with most of the great discoveries in the field of Cognitive Science. This is a real tragedy since Cognitive Science and Neuroscience are properly seen as just two levels of description of the same organ –the brain. Without the abstractions of cognitive science (symbols, declarative memory chunks, production rules, goal buffers, etc.) neuroscience is faced with an insurmountable gap to cross in its attempt to explain how neurons give rise to mind. It would be like having to explain how the computer program Microsoft Word works by describing its operation at the transistor level. It just cannot be done. One must introduce intermediate levels of abstraction (memory buffers, if-then statements, while-loops, etc.) in order to create a theory of Microsoft Word which is understandable. The same is true when it comes to how mind is generated by the brain.
I argue that any complete theory of how the mind is generated by the physical brain must include at least the following four levels of description:
- Philosophical theories of consciousness and self (Example: Thomas Metzinger’s book “Being No One: The Self-Model Theory of Subjectivity”)
- Cognitive science models of the human cognitive control architecture (Example: John Anderson’s book “How can the human mind occur in the physical universe” –his most recent overview of ACT-R)
- Abstract “artificial” neural network architectures of hippocampal memory systems, perceptual feature hierarchies, reinforcement learning pattern recognition networks, etc. (Example: Edmund Rolls’ book “Neural Networks and Brain Function”)
- Electrical and molecular models of real biological neurons and systems (Example: Kandel, Schwartz and Jessell’s book “Principles of Neural Science”)
If you cannot deftly switch between these levels of description, understanding the core principles of each and how they are built upon one another, then you are unprepared to understand what is required to accomplish whole brain emulation. Simply understanding neuroscience is not enough.
One of the weakest links which exists today in this hierarchy of descriptive levels is the link between #2 and #3. This is why I have been putting considerable effort into showing how the symbolic computations assumed by ACT-R might be implemented by standard artificial neural network models of autoassocative memory etc. My 2012 publication “Dynamically partitionable autoassociative networks as a solution to the neural binding problem” is a first attempt at this. I am currently preparing a new publication and set of neural models designed to make this link even clearer. My goal is to demonstrate to the neuroscience community that the level of abstraction assumed by cognitive science models like ACT-R is not so removed from the neural network models which neuroscientists currently embrace. An effective bridging of this gap between cognitive science models and neural network models has the potential to release a wave of synergy between the fields, in which the top-down constraints of cognitive science can directly inform neuroscience models, and the bottom-up constraints of neuroscience can directly inform cognitive models.
Luke: How well-specified is ACT-R? In particular, can you give an example of ACT-R making a surprising, novel quantitative prediction that, upon experiment, turned out to be correct? (ACT-R could still be useful even if this hasn’t happened yet, but if there are examples of this happening then it’d be nice to know about them!)
Kenneth: One difficulty with answering that question is that ACT-R is really a “framework” in which to create models of brain function, it is not a model in and of itself. A researcher typically uses the ACT-R framework to create a model of a particular cognitive task –say modeling how a student learns to solve algebraic expressions. To be successful, the model must make quantitative predictions about the error rates and types of errors, the speed of responses and how this speed increases with practice, etc. Any particular ACT-R model consists of a set of initial “productions” (symbolic pattern matching if-then rules) and “declarative memory chunks” (symbolic memories) which are assumed to have already been learned by the individual. The model will produce intelligent behaviors using these initial production rules and memory chunks. It will also change the weights of the rules and chunks as it learns by trial and error, and it will produce new production rules and new memories as it interacts with its simulated environment.
Now one can ask your question about novel predictions regarding either a particular ACT-R model or regarding the ACT-R framework itself. To pick one particularly good example of an ACT-R model making “surprising and novel” predictions you might want to look at the paper: “A Central Circuit of the Mind” (Anderson, Fincham, Qin, and Stocco 2008). In that paper (and a host of follow up papers) an ACT-R model of a cognitive task was used to predict not only behavioral responses but fMRI BOLD activation levels and timing in subjects performing the cognitive task. Considering that the ACT-R community spent many years developing models of brain function without fMRI data as a constraint, it is particularly intriguing to see how well those models have fared when viewed against this new type of data.
As for predictions of the ACT-R architecture itself, I would like to point to perhaps ACT-R’s central prediction going straight back to its inception –the prediction that there are two types of learning: “procedural” and “declarative”. This distinction is so well established now (think of studies of the amnesia patient HM) that it is hard to remember that this was anything but settled when ACT-R was invented. In fact, this is the key difference between the ACT-R and the SOAR cognitive architectures. SOAR was built on an incorrect prediction –that all knowledge in the brain was encoded as procedural rules. This incorrect prediction is why SOAR lost favor with the cognitive science community and why ACT-R is still widely used.
You ask “How well-specified is ACT-R?”. This is a good question. The ACT-R architecture has changed significantly over the years as new information has become available. Over the last decade it went through a dramatic simplification in the complexity that was allowed for the pattern matching in production rules. It also has set the values of some of its core performance and learning parameters (for example the time it takes to execute a single production rule is now set to be 50ms). These ACT-R architectural parameters were of course determined by fitting data to behavioral experiments, but since ACT-R is meant to be a theory of the brain as a whole, individual models are not allowed to arbitrarily change these parameters to fit their particular data. In effect, these ACT-R architectural features have become a significant constraint on the degrees of freedom modelers have when proposing new ACT-R models of particular cognitive tasks. The success or failure of these tighter specifications of the ACT-R framework parameters are best judged by how successful it still is for modeling a wide range of behaviors. As for that I would again point to the wide range of publications using ACT-R.
I don’t think one should judge ACT-R on the basis of novel predictions however. It is designed as a summary theory to account for a large body of cognitive science facts. It should, and has, changed when its predictions were found unsound. For example, ACT-R’s original model of production matching was essentially found untenable given what we know about neural networks, so it was jettisoned for a new simpler version.
I think the best way to judge ACT-R is to understand what it is meant to do and what it is not meant to do. ACT-R is used to model cognitive tasks at the computational level, not at the neural implementation level. It is quite specific and well specified at the computational level and has been shown to provide satisfying (tentative) explanations for a wide range of intelligent human behaviors at this level. I have argued that it is our best model of the human mind at this computational level and therefore we as neuroscientists should use it as a starting point for understanding what types of high-level computations the neural circuits of the brain are likely performing.
Luke: Back to WBE. You were a participant in the 2007 workshop that led to FHI’s Whole Brain Emulation: A Roadmap report. Would you mind sharing your own estimates on some of the key questions from the report? In particular, Table 4 of the report (p. 39) summarizes possible modeling complications, along with estimates of how likely they are to be necessary for WBE and how hard they would be to implement. Which of those estimates do you disagree with most strongly? (By “WBE” I mean what the report calls success criterion 6a (“social role-fit emulation”), so as to set aside questions of consciousness and personal identity.)
Kenneth: First I would like to say how fantastic it was for Anders and the FHI to put on that workshop. It is amazing to see how far the field has progressed in the meantime. I agree with most of the main conclusions of that report, but since you asked for what I disagree with I will focus in on those few points on which I have reservations.
The report starts off with a section entitled “Little need for whole-system understanding” where the authors state: “An important hypothesis for WBE is that in order to emulate the brain we do not need to understand the whole system, but rather we just need a database containing all necessary low‐level information about the brain and knowledge of the local update rules that change brain states from moment to moment.” (p. 8)
I agree that it is technically possible to achieve WBE without an overall understanding of how the brain works, but I seriously doubt this is the way it will happen. I believe our neuroscience models, and the experimental techniques to test them, will continue their rapid advance over the next few decades so that we will reach a very deep understanding of the brain’s functioning at all levels well before we have the technology for applying WBE to a human. Under that scenario we will understand exactly which features listed in table 4 of the report are needed, and which are unnecessary.
As a concrete example (meant to be controversial), there are several types of cortical neurons thought to provide general feedforward and feedback inhibition to the cortex’s main excitatory cells. These inhibitory cells effectively regulate the percentage of the excitatory cells that are allowed to be active at a given time (i.e. the ‘sparseness’ of the neural representation). I doubt that the details of these inhibitory cells will be modeled at all in a future WBE since it would be much easier, and more reliable, in a computer model to enforce this sparseness of firing directly. Now this suggestion will probably strike many of your readers as a particularly risky shortcut, and they may ask “How can we be sure that the detailed functioning of these inhibitory neurons is not crucial to the operation of the mind?” This may turn out to be the case, but my point here is that by the time we have the technology to actually try a human WBE we will know for sure what ‘shortcuts’ are acceptable. The experiments needed to test these hypotheses are much simpler than WBE itself.
Luke: In your view, what are some specific, tractable “next research projects” that, if funded, could show substantial progress toward WBE in the next 10 years?
Kenneth: Over the next ten years progress in WBE is likely to be tied to progress in the field of connectomics (automated electron microscopic (EM) mapping of brain tissue to reveal the precise connectivity between neurons). Since the 2004 invention of the serial block face scanning electron microscope (SBFSEM, or SBEM) there has been an explosion of interest in this area. SBFSEM was the first automated device to really show how one could, in principle, “deconstruct brain tissue” and extract its precise wiring diagram. Since then the SBFSEM has undergone dramatic improvements and has been joined by several other automated electron microscopy tools for mapping brain tissue at the nanometer scale (TEMCA, ATUM-SEM, FIB-SEM). Kevin Briggman and Davi Bock (two of the leaders in this field) wrote a great review article in 2012 covering the current state of all of these connectomics imaging technologies. In short, neuroscientists finally have some automated tools which can image the complete synaptic connectivity of the neural circuits they have been studying for years by behavioral and electrophysiological means. Several “high profile” connectomics publications have recently come out (Nature 2011, Nature 2011, Nature 2013, Nature 2013, Nature 2014) giving a taste of what can be accomplished with such tools, but these publications represent just the “tip of the iceberg” of what is likely to be a revolution in the way neuroscience is done, a revolution that over the long run will lead to WBE.
There is, however, one thing that is currently holding back the entire field of connectomics – the lack of an automated solution to tracing neural connectivity. Even though there has been fantastic progress in automating 3D EM imaging, each of these connectomics publications required a small army of dedicated human tracers to supplement and “error-correct” the output of today’s inadequate software tracing algorithms. This roadblock has been widely recognized by connectomics practitioners:
“[A]ll cellular-resolution connectomics studies to date have involved thousands to tens-of-thousands of hours of [human labor for neural] reconstruction. Although imaging speed has substantially increased, reconstruction speed is massively lagging behind. For any of the proposed dense-circuit reconstructions (mouse neocortex [column], olfactory bulb, fish brain and human neocortex [column]…), analysis-time estimates are at least one if not several orders of magnitude larger than what has been accomplished to date… requirements for these envisioned projects are around several hundred thousand hours of manual labor per project. These enormous numbers constitute what can be called the analysis gap in cellular connectomics: although imaging larger circuits is becoming more and more feasible, reconstructing them is not.” – Moritz Helmstaedter (Nature Methods review 2013)
A full solution to this “analysis gap” will likely require advances on three fronts:
- Improved 3D imaging resolution
- Improved tissue preservation and staining
- More advanced algorithms
Most of today’s connectomics imaging technologies still rely on physical sectioning of tissue which practically limits the “z” resolution of EM imaging to >20nm. However, the FIB-SEM technique instead uses a focused ion beam to reliably “polish” away a <5nm layer of tissue between each EM imaging step. This means that FIB-SEM datasets can image tissue with voxel resolutions as low as 5x5x5nm. This extra resolution is crucial when trying to follow tubular neuronal processes which can often shrink to <40nm in diameter. This improved FIB-SEM resolution has been demonstrated to dramatically increase the effectiveness of today’s tracing algorithms. I have been working on extending this FIB-SEM technique to make it capable of handling arbitrarily large volumes. I do this by using a heated, oil-lubricated diamond knife to losslessly subdivide a large block of tissue into chunks optimally-sized (~20 microns thick) for high-resolution, parallel FIBSEM imaging. I will have a paper on this coming out later this year.
This higher resolution (FIB-SEM) imaging, along with likely advances in tracing algorithms, should finally be able to achieve the goal of fully-automated tracing of neural tissue –i.e. it should overcome the “analysis gap”, eliminating the reliance on human tracing.
That is, as long as the tissue is optimally preserved and stained for EM imaging. Unfortunately, today’s best tissue preservation and staining protocols are limited to <200 micron thick tissue slabs. This volume limit also represents a fundamental roadblock to connectomics, and there is only one researcher that I know of that has seriously taken up the challenge to remove this limitation –Shawn Mikula. He has made substantial progress toward this goal, but considerable work remains.
So regarding your question for “specific, tractable next research projects”, I would offer up the following: A project to develop a protocol for preserving, staining, and losslessly subdividing an entire mouse brain for random-access FIB-SEM imaging, along with a project to build a “farm” of hundreds of inexpensive FIB-SEM machines capable of imaging neural circuits spanning large regions of this mouse’s brain.
I believe that the Mikula protocol can be extended to provide excellent EM staining for all parts of a mouse brain (Mikula, personal communications). I also believe, with sufficient work and funding, that this mouse protocol could be made compatible with my “lossless subdivision” technique which would allow the entire mouse brain to be quickly and reliably chopped up into little cubes (~50x50x50 microns in size) any of which could be loaded into a FIB-SEM machine for imaging. I also believe, with sufficient funding, that today’s generation of expensive (>1million USD) FIB-SEM machines could be redesigned, and drastically simplified for mass-manufacture.
I think that such a project is indeed tractable over a ten year time frame, and it would in effect provide neuroscientists with a tool (what I have termed a “connectome observatory”) to map out the neural circuits involved in vision, memory, motor control, reinforcement learning, etc. all within the same mouse brain. Allowing researchers to see not only an individual region’s circuits, but also the long distance connections among these regions which allow them to act as a coherent whole controlling the mouse’s behavior. I think it is at this level that we will begin to see rudiments of the types of coordinated, symbol-level operations predicted by cognitive models like ACT-R, the types of executive control circuits which in us evolved to underlie our unique intelligence and consciousness.
We must remember and accept that the long-term goal of human whole brain emulation is almost unimaginably difficult. It is ludicrous to expect significant progress toward that goal in the next decade or two. But I believe we should not shrink away from saying that that goal is the one we are ultimately pursuing. I believe neuroscientists and cognitive scientists should proudly embrace the idea that their fields will eventually succeed in revealing a complete and satisfying mechanistic explanation of the human mind and consciousness, and that this understanding will inevitably lead to the technology of mind uploading. There is so much hard work to be done that we cannot afford to neglect the tremendous payoffs awaiting in the event we succeed.
Luke: Thanks, Kenneth!
Did you like this post? You may enjoy our other Conversations posts, including: