In our last strategy update (August 2016), Nate wrote that MIRI’s priorities were to make progress on our agent foundations agenda and begin work on our new “Alignment for Advanced Machine Learning Systems” agenda, to collaborate and communicate with other researchers, and to grow our research and ops teams.
Since then, senior staff at MIRI have reassessed their views on how far off artificial general intelligence (AGI) is and concluded that shorter timelines are more likely than they were previously thinking. A few lines of recent evidence point in this direction, such as:1
- AI research is becoming more visibly exciting and well-funded. This suggests that more top talent (in the next generation as well as the current generation) will probably turn their attention to AI.
- AGI is attracting more scholarly attention as an idea, and is the stated goal of top AI groups like DeepMind, OpenAI, and FAIR. In particular, many researchers seem more open to thinking about general intelligence now than they did a few years ago.
- Research groups associated with AGI are showing much clearer external signs of profitability.
- AI successes like AlphaGo indicate that it’s easier to outperform top humans in domains like Go (without any new conceptual breakthroughs) than might have been expected.2 This lowers our estimate for the number of significant conceptual breakthroughs needed to rival humans in other domains.
There’s no consensus among MIRI researchers on how long timelines are, and our aggregated estimate puts medium-to-high probability on scenarios in which the research community hasn’t developed AGI by, e.g., 2035. On average, however, research staff now assign moderately higher probability to AGI’s being developed before 2035 than we did a year or two ago. This has a few implications for our strategy:
1. Our relationships with current key players in AGI safety and capabilities play a larger role in our strategic thinking. Short-timeline scenarios reduce the expected number of important new players who will enter the space before we hit AGI, and increase how much influence current players are likely to have.
2. Our research priorities are somewhat different, since shorter timelines change what research paths are likely to pay out before we hit AGI, and also concentrate our probability mass more on scenarios where AGI shares various features in common with present-day machine learning systems.
Both updates represent directions we’ve already been trending in for various reasons.3 However, we’re moving in these two directions more quickly and confidently than we were last year. As an example, Nate is spending less time on staff management and other administrative duties than in the past (having handed these off to MIRI COO Malo Bourgon) and less time on broad communications work (having delegated a fair amount of this to me), allowing him to spend more time on object-level research, research prioritization work, and more targeted communications.4
I’ll lay out what these updates mean for our plans in more concrete detail below.
1. Research program plans
Our top organizational priority is object-level research on the AI alignment problem, following up on the work Malo described in our recent annual review.
We plan to spend this year delving into some new safety research directions that are very preliminary and exploratory, where we’re uncertain about potential synergies with AGI capabilities research. Work related to this exploratory investigation will be non-public-facing at least through late 2017, in order to lower the risk of marginally shortening AGI timelines (which can leave less total time for alignment research) and to free up researchers’ attention from having to think through safety tradeoffs for each new result.5
We’ve worked on non-public-facing research before, but this will be a larger focus in 2017. We plan to re-assess how much work to put into our exploratory research program (and whether to shift projects to the public-facing side) in the fall, based on how projects are progressing.
On the public-facing side, Nate made a prediction that we’ll make roughly the following amount of research progress this year (noting 2015 and 2016 estimates for comparison). 1 means “limited progress”, 2 “weak-to-modest progress”, 3 “modest progress”, 4 “modest-to-strong progress”, and 5 “sizable progress”:6
logical uncertainty and naturalized induction:
- 2015 progress: 5. — Predicted: 3.
- 2016 progress: 5. — Predicted: 5.
- 2017 progress prediction: 2 (weak-to-modest).
decision theory:
- 2015 progress: 3. — Predicted: 3.
- 2016 progress: 3. — Predicted: 3.
- 2017 progress prediction: 3 (modest).
Vingean reflection:
- 2015 progress: 3. — Predicted: 3.
- 2016 progress: 4. — Predicted: 1.
- 2017 progress prediction: 1 (limited).
error tolerance:
- 2015 progress: 1. — Predicted: 3.
- 2016 progress: 1. — Predicted: 3.
- 2017 progress prediction: 1 (limited).
value specification:
- 2015 progress: 1. — Predicted: 1.
- 2016 progress: 2. — Predicted: 3.
- 2017 progress prediction: 1 (limited).
Nate expects fewer novel public-facing results this year than in 2015-2016, based on a mix of how many researcher hours we’re investing into each area and how easy he estimates it is to make progress in that area.
Progress in basic research is difficult to predict in advance, and the above estimates combine how likely it is that we’ll come up with important new results with how large we would expect such results to be in the relevant domain. In the case of naturalized induction, most of the probability is on us making small amounts of progress this year, with a low chance of new large insights. In the case of decision theory, most of the probability is on us achieving some minor new insights related to the questions we’re working on, with a medium-low chance of large insights.
The research team’s current focus is on some quite new questions. Jessica, Sam, and Scott have recently been working on the problem of reasoning procedures like Solomonoff induction giving rise to misaligned subagents (e.g., here), and considering alternative induction methods that might avoid this problem.7
In decision theory, a common thread in our recent work is that we’re using probability and topological fixed points in settings where we used to use provability. This means working with (and improving) logical inductors and reflective oracles. It also means developing new ways of looking at counterfactuals inspired by those methods. The reason behind this shift is that most of the progress we’ve seen on Vingean reflection has come out of these probabilistic reasoning and fixed-point-based techniques.
We also plan to put out more accessible overviews this year of some of our research areas. For a good general introduction to our work in decision theory, see our newest paper, “Cheating Death in Damascus.”
2. Targeted outreach and closer collaborations
Our outreach efforts this year are mainly aimed at exchanging research-informing background models with top AI groups (especially OpenAI and DeepMind), AI safety research groups (especially the Future of Humanity Institute), and funders / conveners (especially the Open Philanthropy Project).
We’re currently collaborating on a research project with DeepMind, and are on good terms with OpenAI and key figures at other groups. We’re also writing up a more systematic explanation of our view of the strategic landscape, which we hope to use as a starting point for discussion. Topics we plan to go into in forthcoming write-ups include:
1. Practical goals and guidelines for AGI projects.
2. Why we consider AGI alignment a difficult problem, of the sort where a major multi-year investment of research effort in the near future may be necessary (and not too far off from sufficient).
3. Why we think a deep understanding of how AI systems’ cognition achieves objectives is likely to be critical for AGI alignment.
4. Task-directed AGI and methods for limiting the scope of AGI systems’ problem-solving work.
Some existing write-ups related to the topics we intend to say more about include Jessica Taylor’s “On Motivations for MIRI’s Highly Reliable Agent Design Research,” Nate Soares’ “Why AI Safety?”, and Daniel Dewey’s “Long-Term Strategies for Ending Existential Risk from Fast Takeoff.”
3. Expansion
Our planned budget in 2017 is $2.1–2.5M, up from $1.65M in 2015 and $1.75M in 2016. Our point estimate is $2.25M, in which case we would expect our breakdown to look roughly like this:
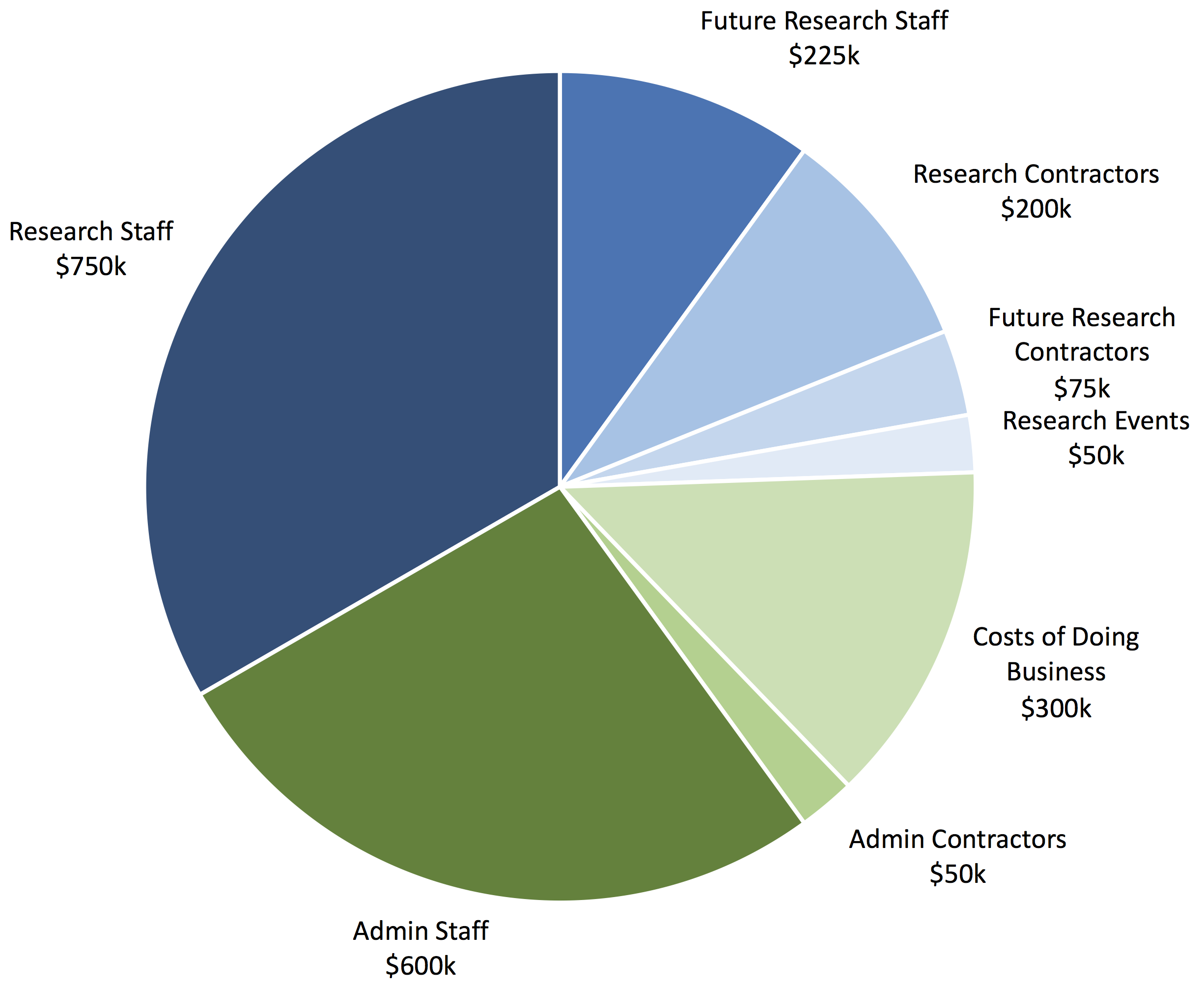
We recently hired two new research fellows, Sam Eisenstat and Marcello Herreshoff, and have other researchers in the pipeline. We’re also hiring software engineers to help us rapidly prototype, implement, and test AI safety ideas related to machine learning. We’re currently seeking interns to trial for these programming roles (apply here).
Our events budget is smaller this year, as we’re running more internal research retreats and fewer events like our 2015 summer workshop series and our 2016 colloquium series. Our costs of doing business are higher, due in part to accounting expenses associated with our passing the $2M revenue level and bookkeeping expenses for upkeep tasks we’ve outsourced.
We experimented with running just one fundraiser in 2016, but ended up still needing to spend staff time on fundraising at the end of the year after falling short of our initial funding target. Taking into account a heartening end-of-the-year show of support, our overall performance was very solid — $2.29M for the year, up from $1.58M in 2015. However, there’s a good chance we’ll return to our previous two-fundraiser rhythm this year in order to more confidently move ahead with our growth plans.
Our 5-year plans are fairly uncertain, as our strategy will plausibly end up varying based on how fruitful our research directions this year turn out to be, and based on our conversations with other groups. As usual, you’re welcome to ask us questions if you’re curious about what we’re up to, and we’ll be keeping you updated as our plans continue to develop!
- Note that this list is far from exhaustive. ↩
- Relatively general algorithms (plus copious compute) were able to surpass human performance on Go, going from incapable of winning against the worst human professionals in standard play to dominating the very best professionals in the space of a few months. The relevant development here wasn’t “AlphaGo represents a large conceptual advance over previously known techniques,” but rather “contemporary techniques run into surprisingly few obstacles when scaled to tasks as pattern-recognition-reliant and difficult (for humans) as professional Go”. ↩
- The publication of “Concrete Problems in AI Safety” last year, for example, caused us to reduce the time we were spending on broad-based outreach to the AI community at large in favor of spending more time building stronger collaborations with researchers we knew at OpenAI, Google Brain, DeepMind, and elsewhere. ↩
- Nate continues to set MIRI’s organizational strategy, and is responsible for the ideas in this post. ↩
- We generally support a norm where research groups weigh the costs and benefits of publishing results that could shorten AGI timelines, and err on the side of keeping potentially AGI-hastening results proprietary where there’s sufficient uncertainty, unless there are sufficiently strong positive reasons to disseminate the results under consideration. This can end up applying to safety research and work by smaller groups as well, depending on the specifics of the research itself.
Another factor in our decision is that writing up results for external consumption takes additional researcher time and attention, though in practice this cost will often be smaller than the benefits of the writing process and resultant papers. ↩
- Nate originally recorded his predictions on March 21, based on the progress he expected in late March through the end of 2017. Note that, for example, three “limited” scores aren’t equivalent to one “modest” score. Additionally, the ranking is based on the largest technical result we expect in each category, and emphasizes depth over breadth: if we get one modest-seeming decision theory result one year and ten such results the next year, those will both get listed as “modest progress”. ↩
- This is a relatively recent research priority, and doesn’t fit particularly well into any of the bins from our agent foundations agenda, though it is most clearly related to naturalized induction. Our AAMLS agenda also doesn’t fit particularly neatly into these bins, though we classify most AAMLS research as error-tolerance or value specification work. ↩